ArcGIS Data Reviewer, an extension to ArcGIS, automates, simplifies, and improves data quality control workflows to deliver geospatial data you can trust. It enables you to lower data management costs and reduce risk in decision-making by providing a unified set of capabilities to detect, manage, and report data errors.
In this latest release, ArcGIS Data Reviewer introduces simplified workflows to implement Attribute rule-based data validation.
Attribute Rule Integration
Starting with ArcGIS Pro 2.3, Data Reviewer integrates and supports data validation workflows using Attribute rules—user-defined rules that automatically populate attributes, restrict invalid edits during editing operations, and assess quality of existing features. The goal of this integration, which will continue to be built over the next several releases, is to bring Data Reviewer’s automated data validation and error management capabilities into a common framework of Attribute rule-based workflows.
Automated Validation
In ArcGIS Pro 2.5 and ArcGIS Enterprise 10.8, Data Reviewer enables users to implement attribute validation rules using configurable checks that identify common quality issues. These data checks are configurable and easy to implement as they don’t require specialized programming skills in Arcade. Data Reviewer’s checks are supported in both: attribute constraint workflows, where they enhance quality assurance in data production, and in validation workflows, where they assess quality of features that already exist in the database.
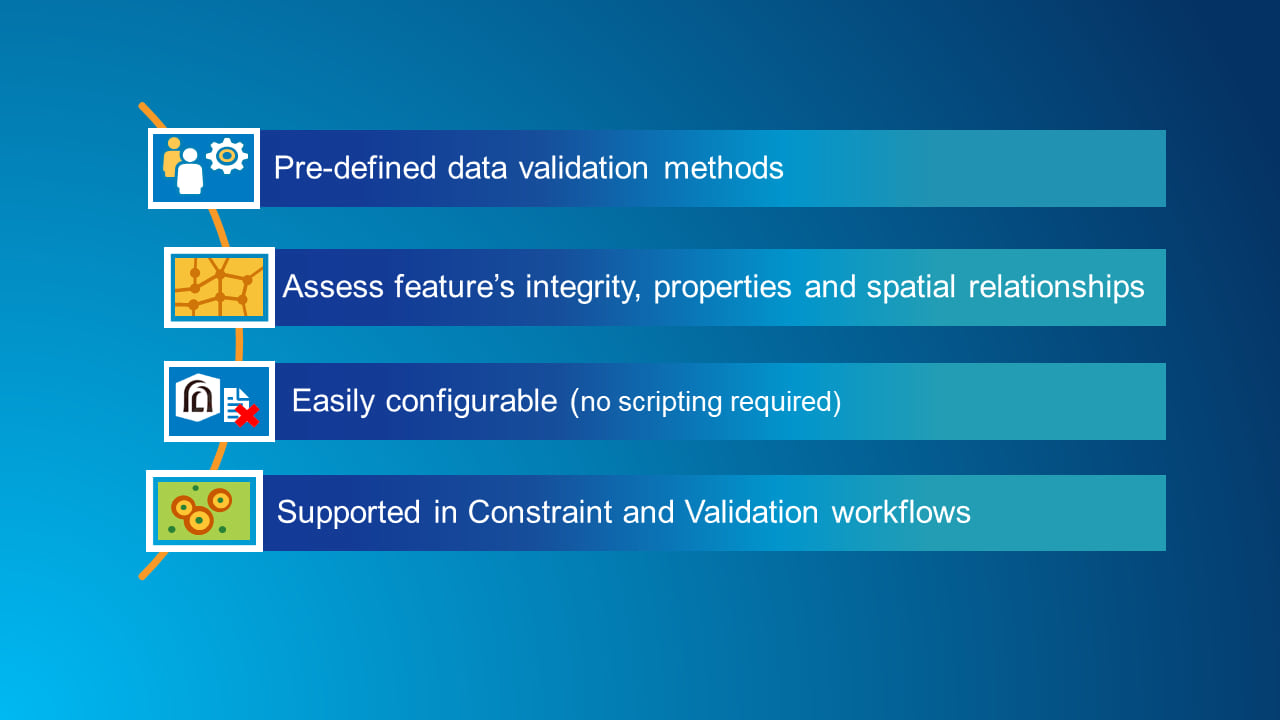
Data Reviewer checks that support automated validation workflows include assessing a feature’s geometric integrity, attribution, and its relationship with other features.
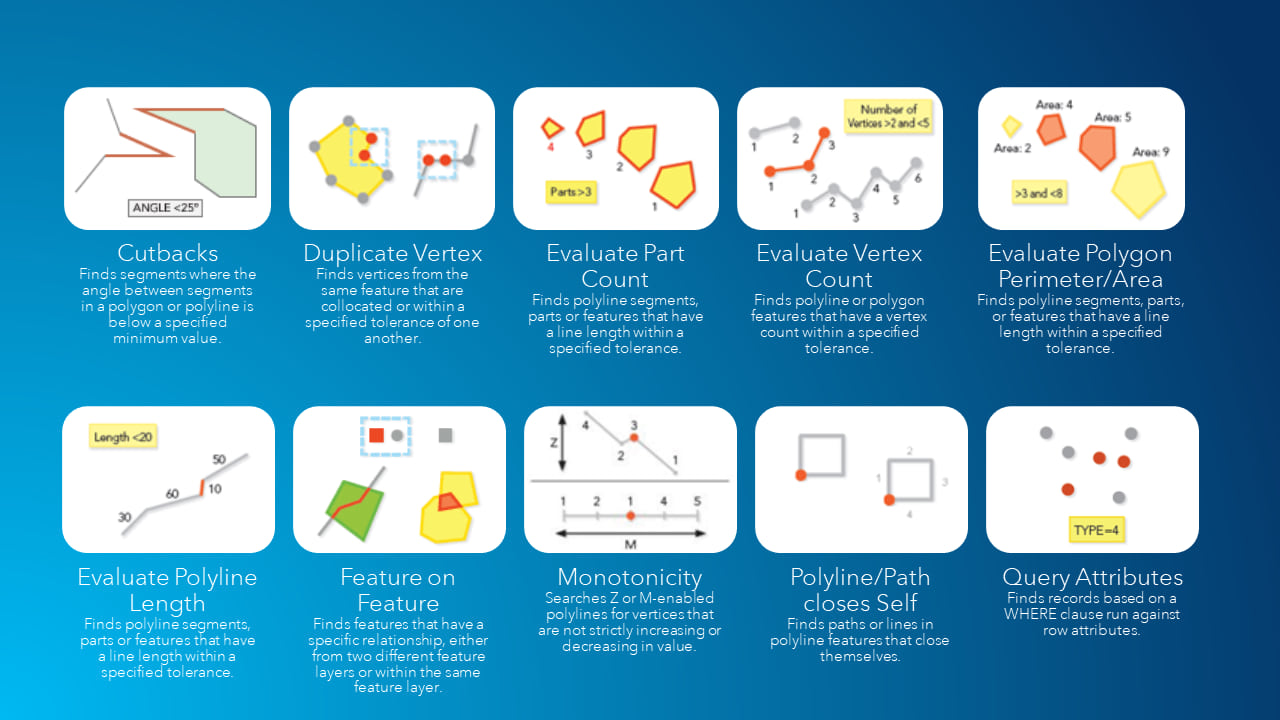
This video shows how Data Reviewer’s configurable checks are used to implement data quality requirements.
Error Management
Understanding the scope and source of poor-quality data enables organizations to efficiently address quality issues and provide transparency to data consumers. Error features created using Data Reviewer’s automated checks provide a greater amount of detail than when using Arcade-based Attribute rules. In the below example, a facility feature should not overlap another facility feature. Using the Feature on Feature check, the area of the feature overlap is used as the error feature’s geometry rather than the original feature’s geometry. Having errors represented more accurately makes it faster and easier for corrective actions, since editors will spend less time looking for the problem.
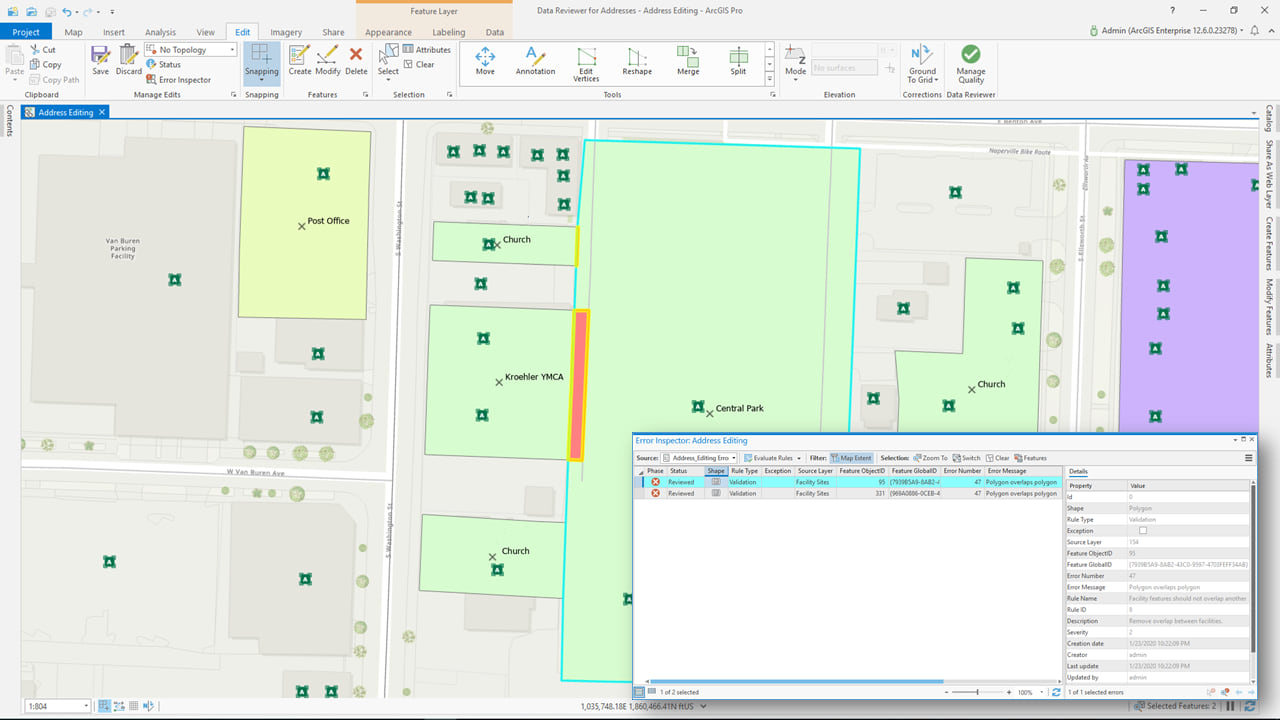
Data Reviewer extends error workflows by applying a life cycle approach to error management. This approach enables the tracking of how and when errors are corrected, as well as who corrected them.
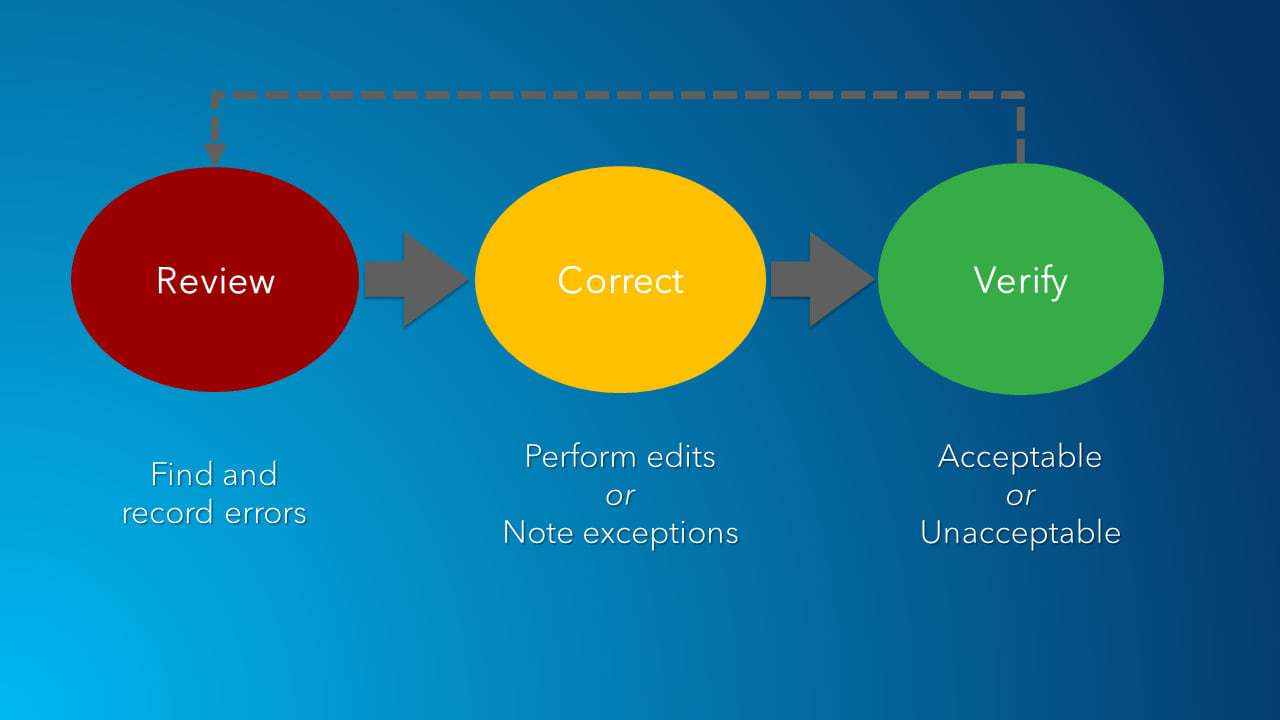
Life cycle information is stored with error features and automatically updated as errors travel through corrective workflows, saving time for editors from manually updating error status. In addition, this level of reporting enables organizations to measure progress on their data quality goals and identify opportunities for process improvement.
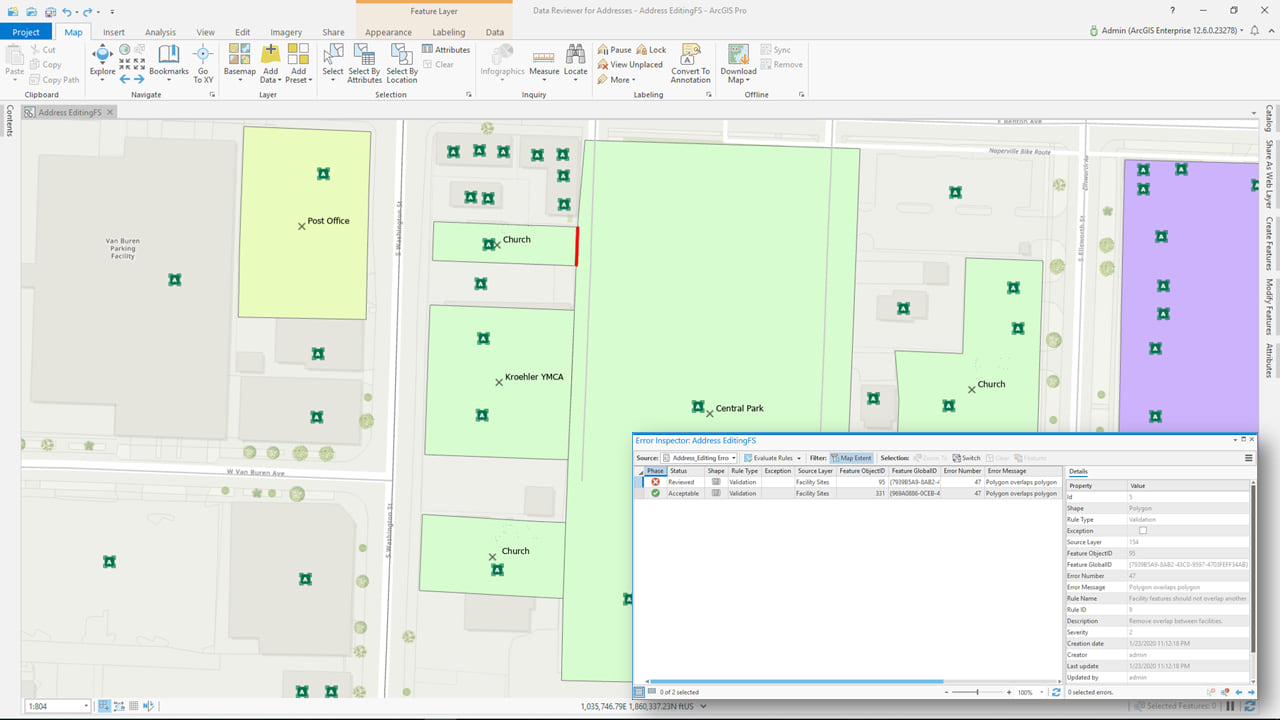
This video shows how Data Reviewer’s automated checks can be used to identify features in your database that do not comply with data quality requirements.
Watch entire video here.
Article Discussion: