Generative AI continues to advance, and we are finding more ways to include these AI assistants in helping us solve various tasks. Included are the discovery of new drugs, elementary particles, and even new laws of physics.
Here we are going to talk about another such discipline, where AI-assistants might play a significant role. A role which may open the art of Urban Planning to more communities, democratize it, make it accessible, and transparent. Urban Planning – the art and science of how we, as a social species, establish and improve our urban centers.
Depletion of ecosystems, rising temperatures and sea levels, massive forest fires, torrential rains, and thawing permafrost – this is the new world we live in. We need to adapt, learn, and then adapt and learn again. According to UN, 68% of world’s population will be living in urban areas by 2050. What can we do in our cities to mitigate the adverse effects of climate change? How can we make our cities more efficient, yet livable and comfortable? How can we make our cities carbon-neutral, pedestrian friendly, and supportive of diverse, vibrant communities with green parks full of shade and cool ponds instead of oversized parking lots?
Perhaps this is where AI may help. With AI, we can build cities that are not only smarter but also more sustainable and enjoyable for everyone.
What is Urban Planning?
Urban Planning is a technical and political discipline focused on use of land, the design of built spaces, transportation and infrastructure, population distribution, and facility accessibility. The Urban Planning process is driven by a complex set of requirements and expectations, including sustainability, zoning and land use mix, diversity, population distribution, resident and guest experience, equal access to public resources and green space, as well as the preservation of culture, history, and tradition.
Urban planners strive to create cities that are functional, equitable, and sustainable. They address issues such as traffic congestion, housing shortages, and environmental impact by developing comprehensive plans that balance growth with the needs of the community. For example, effective urban planning can lead to more walkable neighborhoods, improved public transportation systems, and increased green spaces, all of which enhance the quality of life for residents.

Challenges of modern Urban Planning
Modern urban planning presents numerous challenges. The field requires a steep learning curve, the absence of universal processes, and decades of combined team experience in infrastructure, architecture, operations research, sociology, history, and culture.
When tackling a project, urban planning teams often face a myriad of complex requirements. Sustainability, livability, and resilience are at the forefront, with goals such as achieving carbon-neutral or even carbon-positive outcomes. Ensuring equal access to facilities and services is critical, promoting walkability, public transportation, and car-free zones. Moreover, modern urban plans must incorporate advanced power infrastructure, be friendly to renewable energy, support water recycling, and consider the orientation of prevailing winds. These demands are often entangled and sometimes conflicting, such as balancing gross floor area, energy consumption, daylight index, and school access.
Multiple unknowns further complicate urban planning. Subjective perceptions of what constitutes a good city vary, and the unclear causality between urban planning decisions and environmental performance adds to the complexity. The evolution of a city depends on numerous phenomena developing over time, making it difficult to capture these dynamics as formal rules.
Urban plans must align with the existing cultural context while fostering a modern lifestyle and enhancing the quality of life. Plans must create unique and recognizable environments that offer quality experiences that cultivate a sense of place.
Optimally meeting planning requirements is both an art and an optimization problem, which requires human expertise and demands smooth communications channels that connect the urban planning team with the local government, residents, and the AEC (Architecture Engineering, and Construction) community. Frequent community engagements demand a fast-planning cycle, transparency, and explainability of proposals. All of this must be accomplished within time and budget constraints.
Why AI?
Transitioning from a conceptual plan to a detailed masterplan involves numerous iterations of massing studies, each evaluated against both quantitative and qualitative criteria. As the scale of the project increases, this process becomes increasingly time consuming, even with modern procedural modeling tools.
Imagine having an assistant that learns from exemplary “prototypical” cities known for their outstanding urban planning and high standard of living. During the training, this AI would capture the complex and unstructured properties of these cities. Once trained, it could help planners seamlessly apply these best practices to new developments, from neighborhoods to entire cities.
The pencil and computer are, if left to their own devices, equally dumb and only as good as the person driving them.
In this quote Norman Foster underscores the idea that technology is only as effective as the creativity and skill of the person using it, which will continue to be the case as generative AI tools become available.
A data and knowledge driven AI solution, working side-by-side with human experts, could be the key to rapidly creating efficient, livable, and well-designed urban spaces.
What is Urban Massing Generator?
The Urban Massing Generator focuses on the Massing Studies phase of Urban Planning, which is a labor-intensive process. During this phase, flat-roof buildings are strategically placed within the boundaries of city blocks and roads. Zoning assignments are adjusted, population distribution is optimized, facilities are allocated, and accessibility goals are refined. The Urban Massing Generator streamlines this process, allowing for efficient and effective planning.
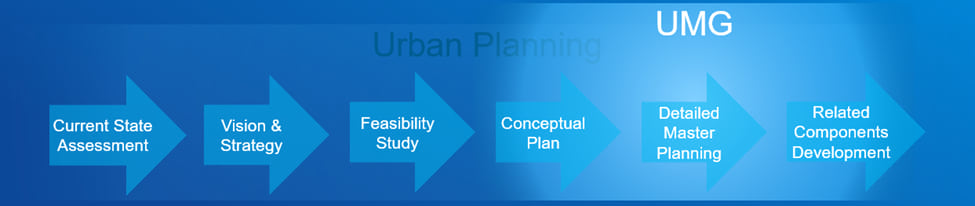
The user workflow starts with defining the streets, which can be imported from Open Street Map, generated procedurally, and manually edited. In this case we are looking at a portion of Paris, France.
The street network implicitly defines the blocks where the building LOD1.3 models will be placed, thus also distributing the future residential population.
Figure 1. ArcGIS CityEngine is used here as a user front-end allowing for convenient planner’s interaction with the UMG geoprocessing service. This is not a released functionality of CityEngine, but rather an internal prototype at this point.
Each block has a zoning category attribute, as well as two numeric density attributes: Building Coverage Ratio (BCR) and Floor Area Ratio (FAR). These attributes determine the size and height of the buildings within the block.
These metrics are standard tools that urban planners use to guide the Massing Studies.
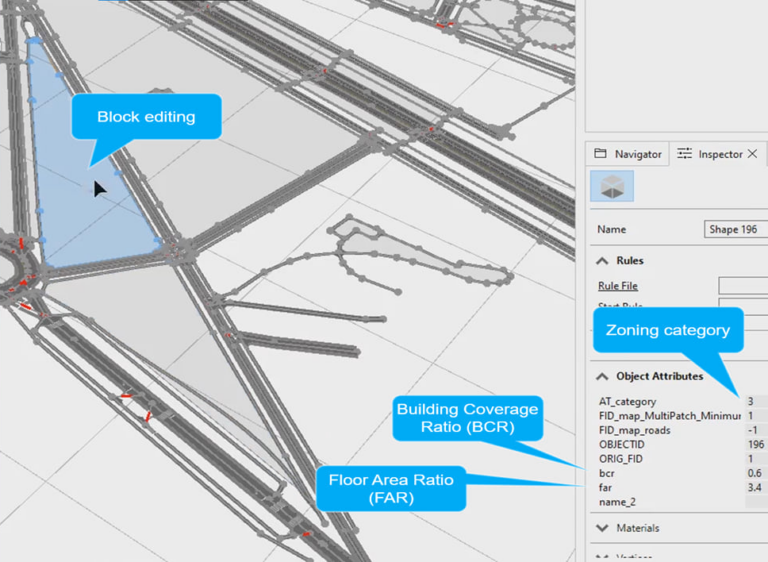
Figure 2. Planner specified block attributes: Zoning (AT_category), Building Coverage Ratio (BCR) and Floor Area Ratio (FAR) densities. The values will determine the size and height of the buildings which will be generated in this block.
Once the street network and block attributes are defined, the AI-assistant creates the first massing model. These buildings are generated by an AI-assistant trained on existing “prototypical” cities known for their high urban planning standards, which include ample green spaces, ubiquitous pedestrian access, and well-developed infrastructure. During the generation process, the assistant applies the learned best practices from these prototypical cities to new development projects. The building shapes, granularity, and their meaningful orientation relative to the roads and each other are derived from generalizing the knowledge gained from these exemplary cities. While the AI-assistant provides creative inspiration, the building footprint sizes and heights are strictly controlled by the user specified numeric FAR and BCR metrics.
Figure 3. The UMG service, with the help of generative AI, creates initial Massing models based on the user specified streets and blocks.
Planners can easily edit streets to design new neighborhoods. For instance, in Figure 4, the planner adds new streets, splitting a large block into four smaller ones. Afterwards, the blocks are rebuilt, and the default block attributes are redefined. The planner switches the zoning to Residential and requests smaller, low-rise buildings by adjusting the FAR and BCR attributes.
Next, the planner decides that the top-left block needs to be of the Mixed-residential zoning category to enhance accessibility to city services and shopping for local and neighboring residents.
When the yellow buildings appear in the last edited block, note how these new taller buildings have more of a commercial appearance, reflecting the patters learned earlier by the AI assistant.
Figure 4. Brownfield development use case: replanning a neighborhood by adding new roads and changing zoning category.
After constructing the massing model, the population distribution is calculated. Considering travel costs along the street network, critical facilities such as green zones, shopping centers, and schools are strategically placed to maximize pedestrian accessibility and minimize overall travel time for residents.
Call for partners!
We are seeking urban planning organizations and local governments of large metropolitan areas to collaborate on AI-assisted Urban Planning, focusing on both greenfield and brownfield developments. If you are interested in partnering with us, please reach out to us via aip_umg@esri.com
Article Discussion: