With greater consumption of imagery than ever before, the need to reliably use AI to automate feature extraction has amplified. Pretrained deep learning models eliminate the need for huge volumes of training data, massive compute resources, and extensive artificial intelligence (AI) knowledge. Users can accelerate their geospatial workflows with ready-to-use models or fine-tune to a specific geography. We have added several new models to our growing collection in the Living Atlas, making deep learning more accessible than ever before.
As of May 2022, with the release of 10 new models, users can now choose from 32 different pretrained models to use. These models are available as deep learning packages (DLPKs) that can be used with ArcGIS Pro, ArcGIS Image Server and ArcGIS API for Python. Here is a brief overview of the new models we’ve added.
Classification
High Resolution Land Cover Classification—USA
Land cover maps are designed to support decisions in urban planning, resource management, change detection, agriculture, and a variety of other applications in which information related to the Earth surface is required. This pretrained deep learning model was trained to classify at approximately 1 meter spatial resolution. Users can leverage this model to automate the tedious manual process and significantly reduce the time and effort required.
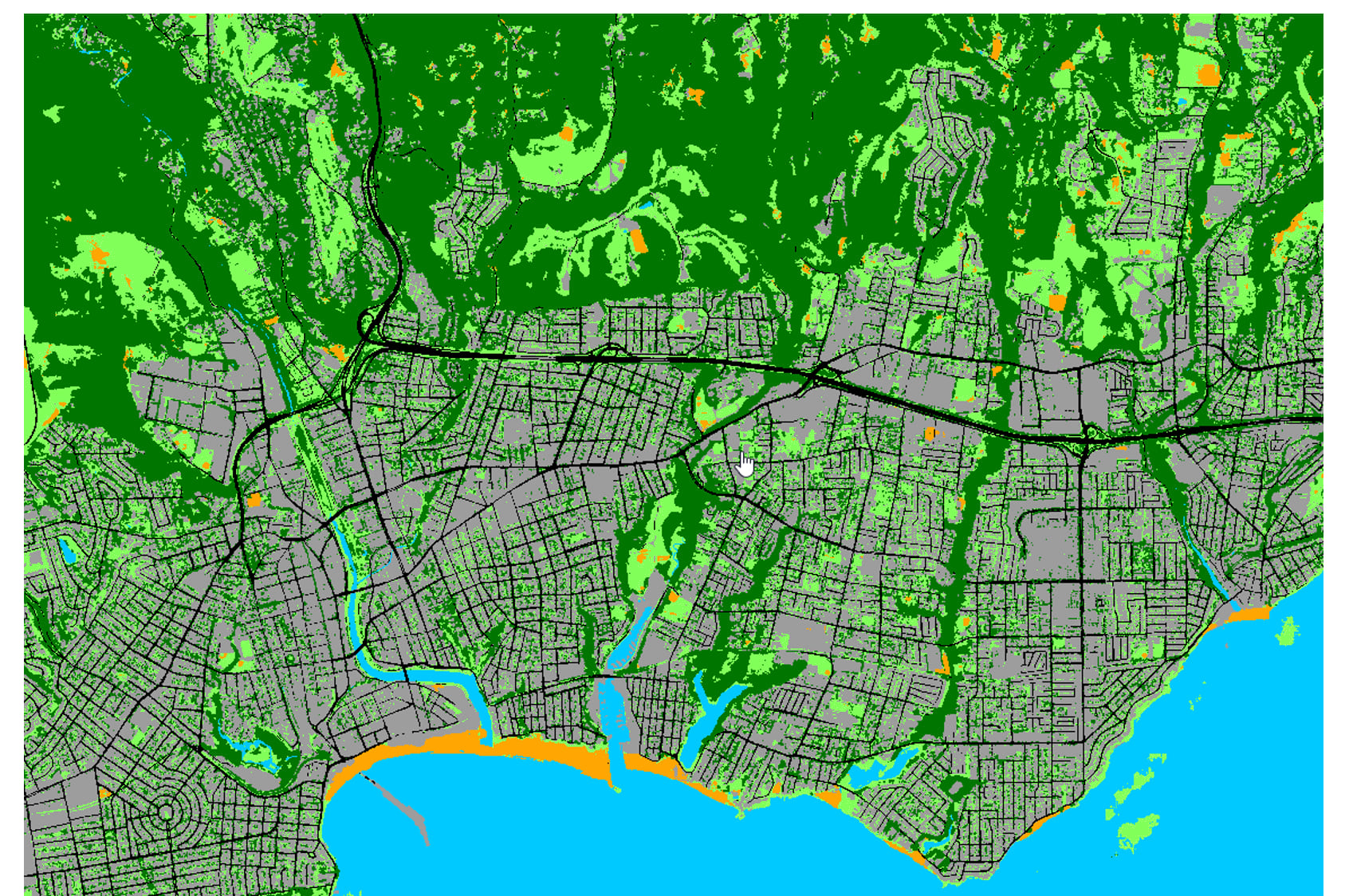
Mangrove Classification (Landsat 8)
Mangroves are critical to the maintenance and conservation of healthy coastal ecosystems. However, due to various climatic phenomena and coastal activity such as reclamation for development, mangrove forests are rapidly shrinking. This warrants active monitoring and conservation efforts of mangroves. This deep learning model enables rapid monitoring of mangrove forests from Landsat 8 imagery.
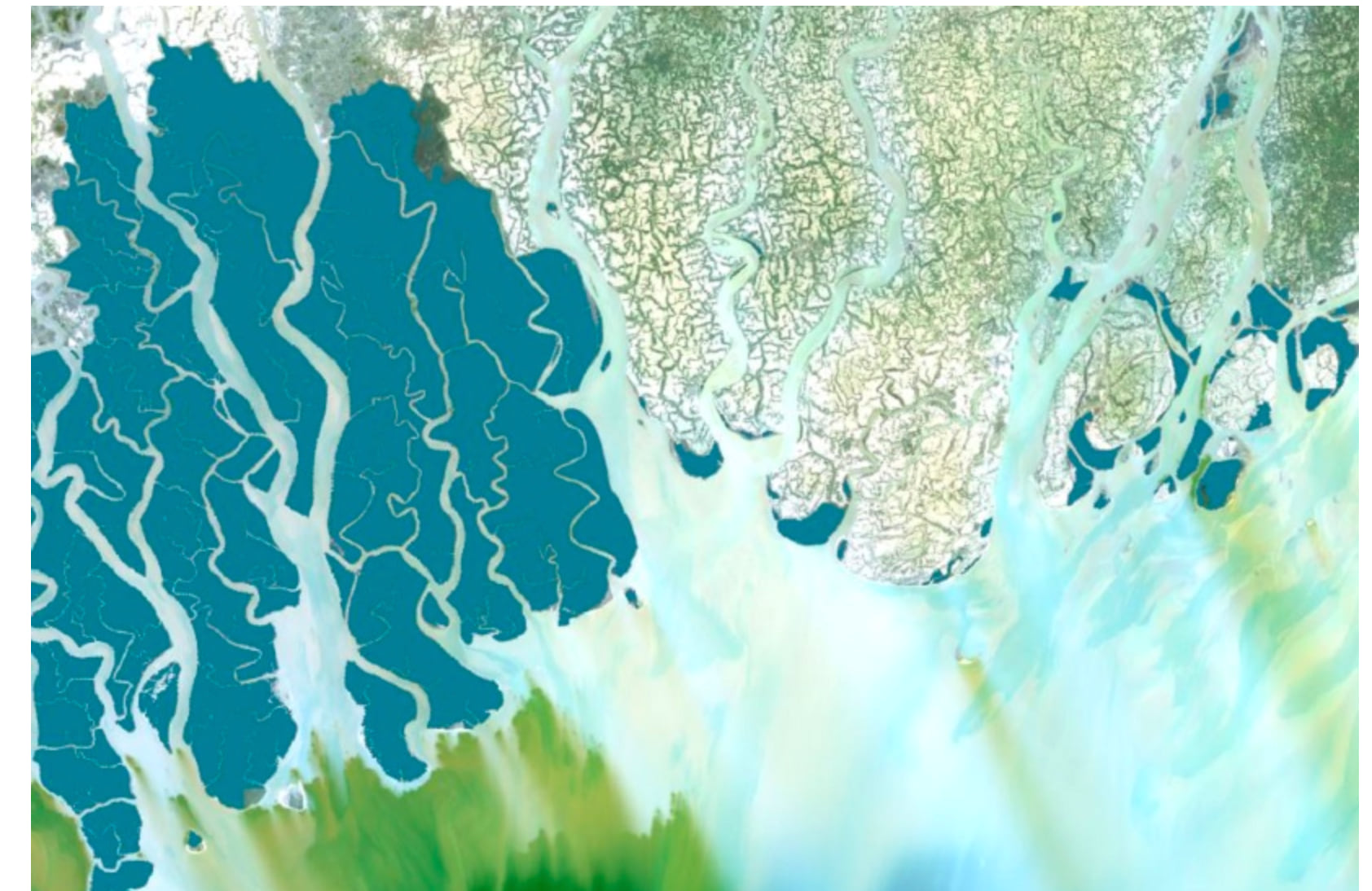
Feature Extraction
Building Footprint Extraction—Australia
The building footprints model developed for the US was met with such positive interest that we developed a similar model for the Australian region. With this pretrained model, you can extract building footprints from high-resolution three-band imagery for Australia. The building footprint layers are useful in preparing base maps and analysis workflows for urban planning and development, change detection, infrastructure planning, and a variety of other applications.
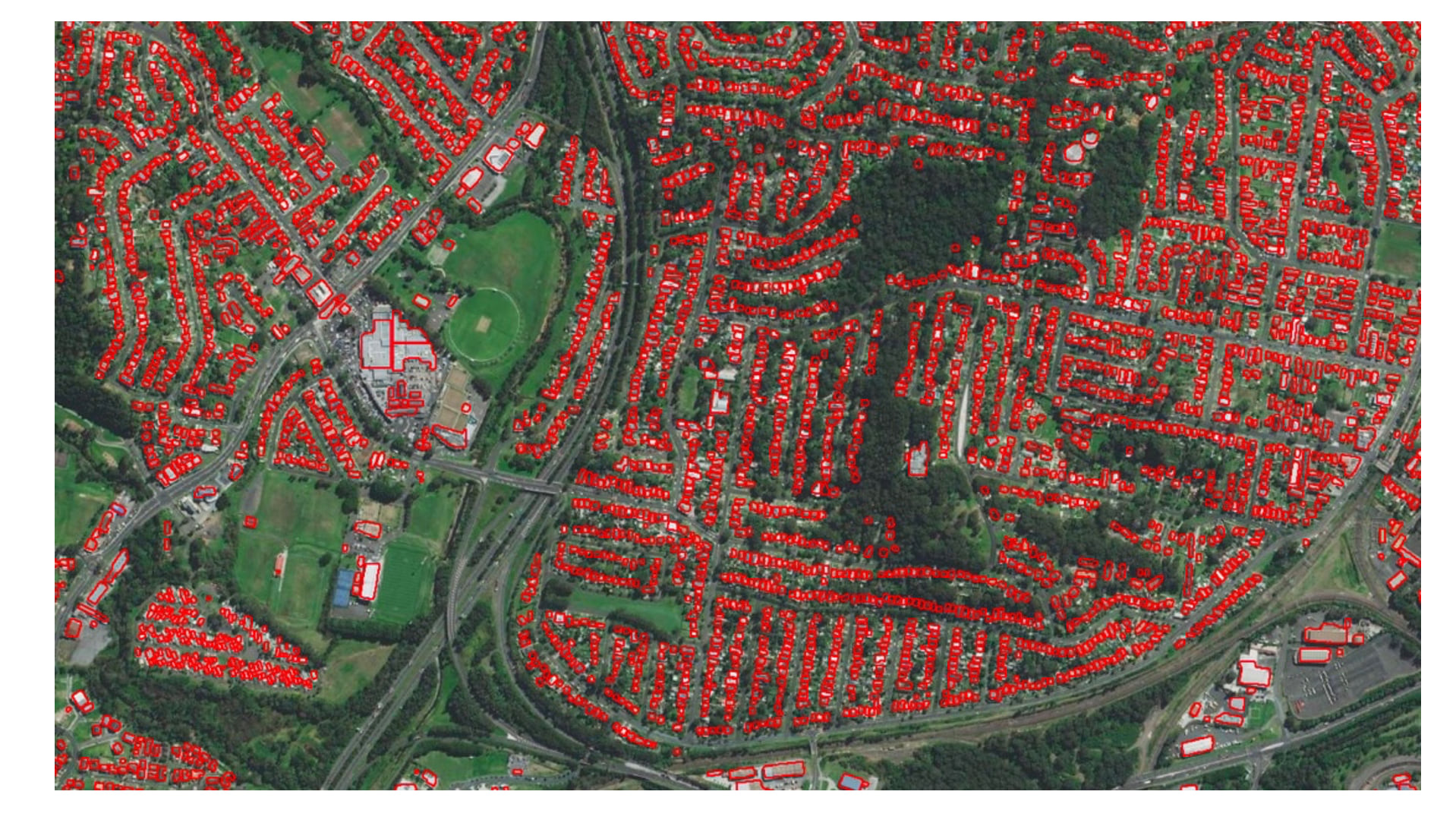
Road Extraction—Global
Since digitizing roads manually from imagery can be a time-consuming task, this deep learning model was designed to automate the process and reduce the time and effort required for acquiring road layers. This model can extract roads from high resolution (1 meter) aerial/satellite imagery.
Detection
Arctic Seal Detection
Monitoring the count of any animal is important for understanding its habitat and helps in its conservation. Seals form an important part of the food chain in the cryosphere region of the Arctic. With drones being able to work in extreme climatic conditions and cover vast areas in minimal time, high-resolution drone imagery of the Arctic region is used to detect seals.
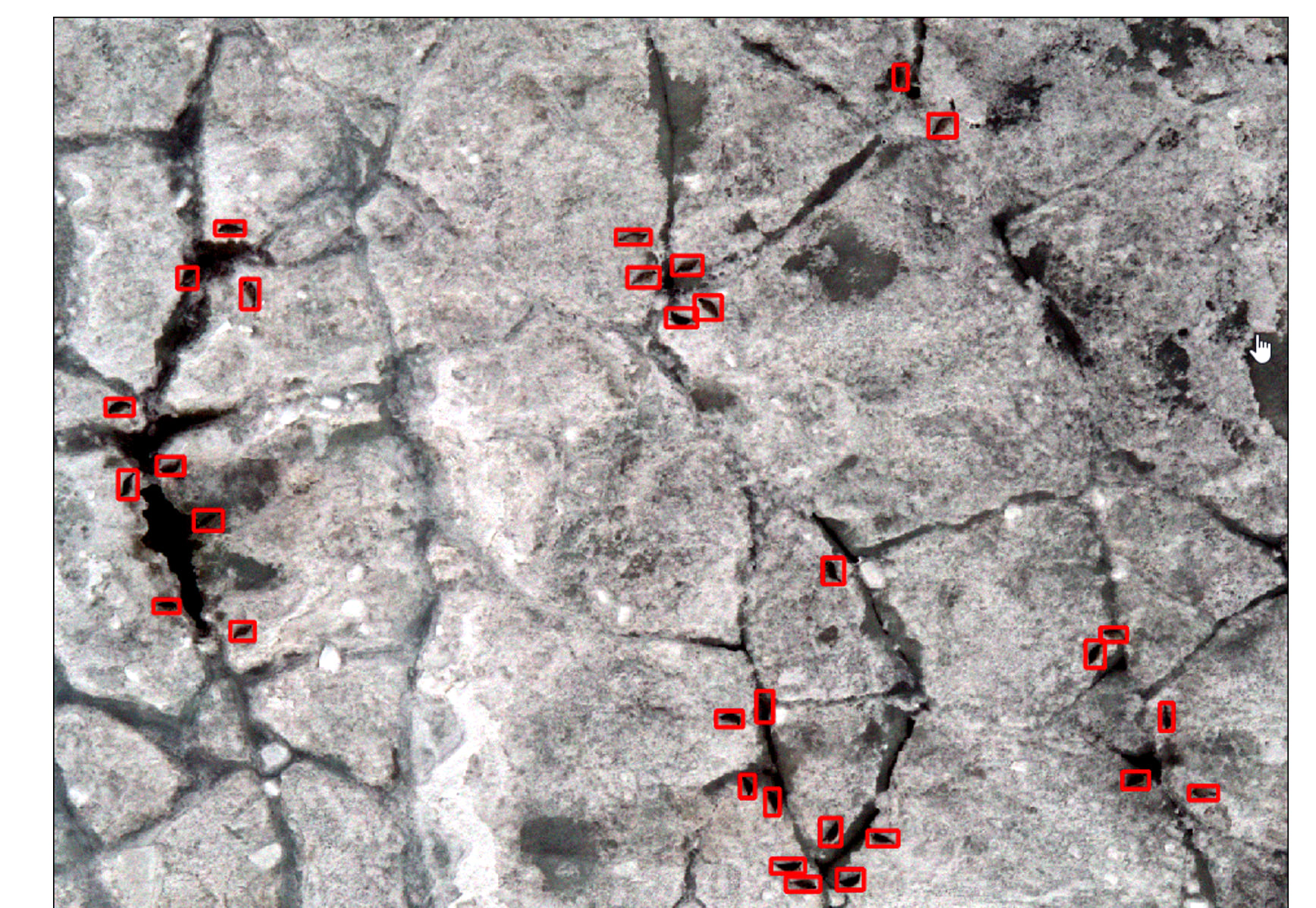
Pavement Crack Detection
Deterioration of road surfaces may be caused by poor construction, heavy load, or weather factors, negatively affecting road safety, driving comfort, and the wear and tear of vehicles. Civic authorities often need to accurately identify these cracks and perform repair work. This model helps automate the task of detecting pavement cracks and potholes in drone imagery to enable better monitoring and maintenance of roads.
Wind Turbine Detection
Another new detection model we added is for wind turbines. With increased importance on renewable energy options, there is rapid growth in the number of wind turbine installations worldwide. These installations are visible in high resolution aerial imagery but can be tedious to analyze and mark these installations manually. Using our model, you can automate the detection of wind turbines by interpreting high resolution imagery.
Well Pad Detection—Permian Basin
Well pads are relatively flat areas that are cleared for drilling and extraction of oil and natural gas. This pretrained model detects well pads on Sentinel-2 imagery and can be used to monitor the progress of new drilling. It can provide competitive intelligence as well as potentially help identify illegal drilling. Additionally, knowing where the new well pads are coming up can enable better planning and resource allocation.
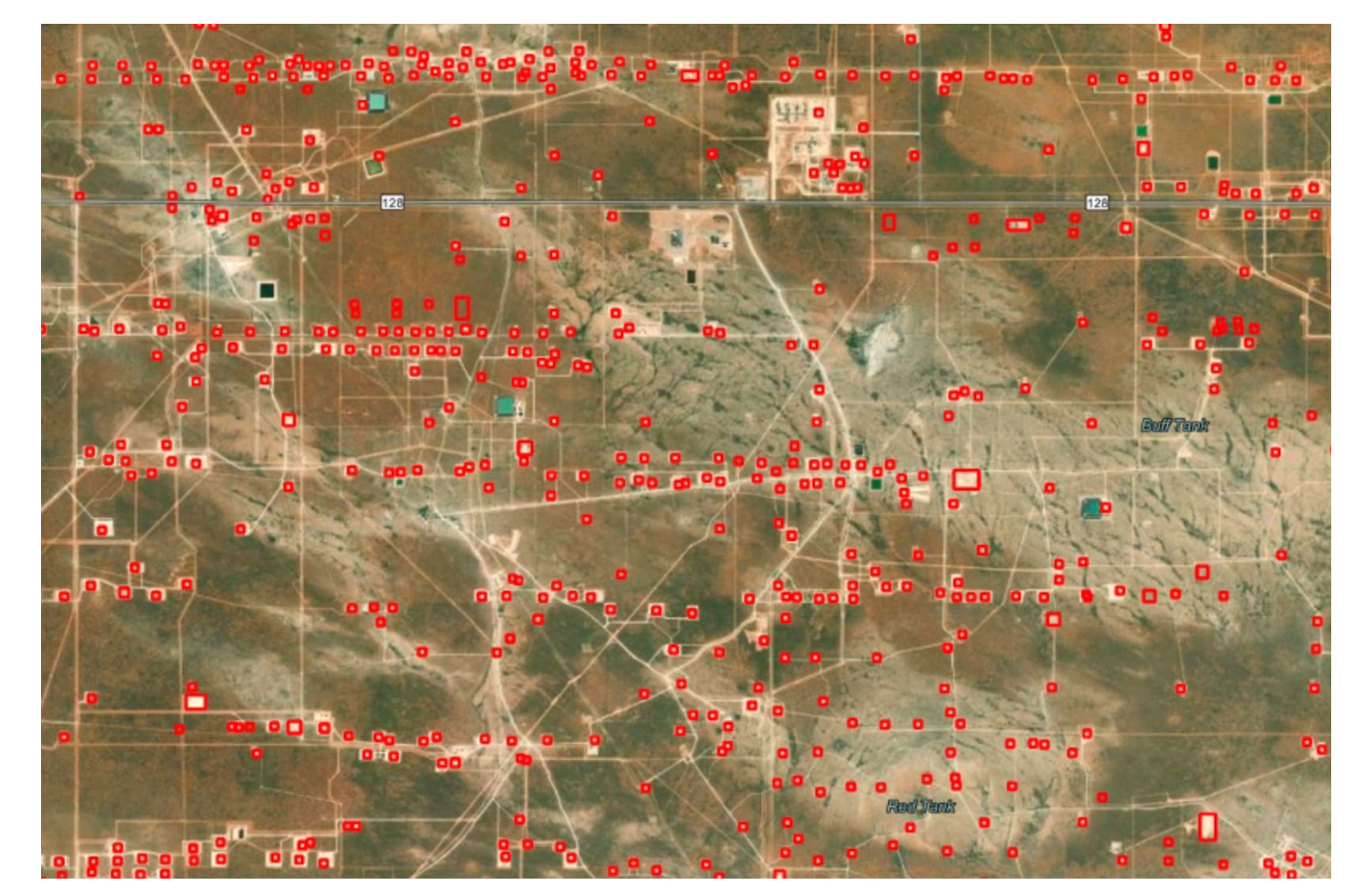
Palm Tree Detection
This pretrained deep learning model is used to detect palm trees in high resolution drone or aerial imagery. Palm trees detection can be used to create an inventory of palm trees, monitor their health and location, and predict the yield of palm oil, etc.
Other
Scene Text Parsing
Extracting text from natural scenes in our environment such as road signs, billboards and house numbers can provide additional context and details about the places the text describes and the information it conveys. This pretrained model was trained on a large dataset of different types and styles of text with diverse background and contexts, allowing for precise text extraction. It can be applied to various tasks such as automatically detecting and reading text from sign boards, scanned maps, etc., thereby converting images containing text to actionable data.
Conclusion
Based on user request and suggestions, these are a few of the models that have been developed recently to automate and simplify your workflows. Available on ArcGIS Living Atlas of the World, each model includes helpful documentation to get you started.
Have questions? Let us know on the Imagery & Remote Sensing community how these models are supporting your work.
What other feature extraction tasks would you like AI to do for you?
Article Discussion: