With the latest version of ArcGIS Pro, new features have been added to enhance the AI capabilities for imagery. Here’s a rundown of capabilities that you can look forward to.
AI-assisted Labeling
We have greatly expanded and enhanced labelling with AI-assisted support for text prompts. This has the potential to improve the productivity of the labelling process.
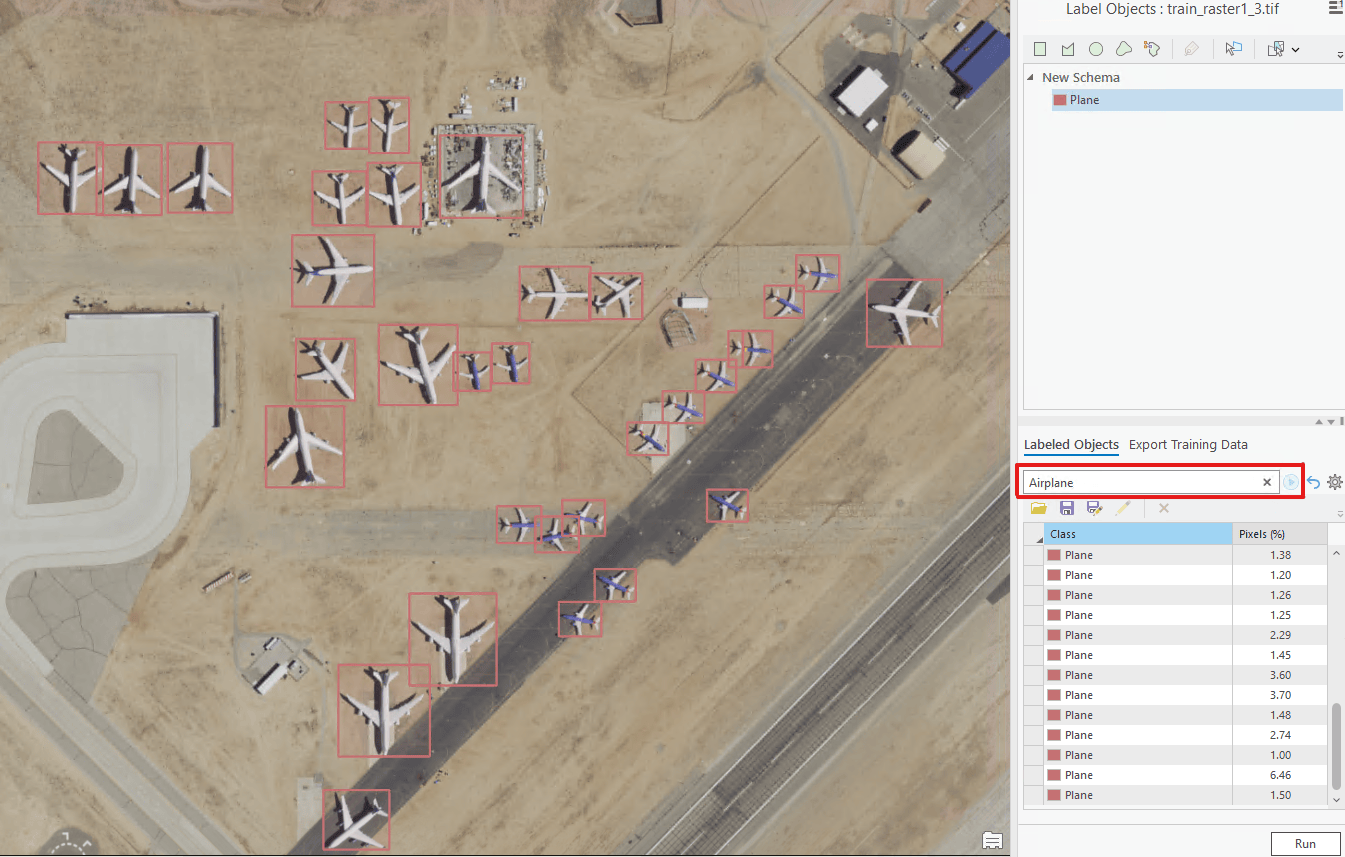
SAMLoRA Model Type
Meta’s Segment Anything (SAM) model is trained on a diverse set of 11 million images and over 1 billion masks. This makes the model highly robust in identifying object boundaries and differentiating between various objects across domains, even though it might have never seen them before. The new SAMLoRA model type applies the low-rank-adaptation (LoRA) technique to the SAM model and fine-tunes it for geospatial image segmentation with relatively low computing resources and a smaller training dataset.
The following graphic shows buildings extracted using the fine-tuned SAMLoRA model on natural color imagery with a fairly small training dataset. This example used only 270 image chips and 20 epochs for the training, which took less than 20 minutes.
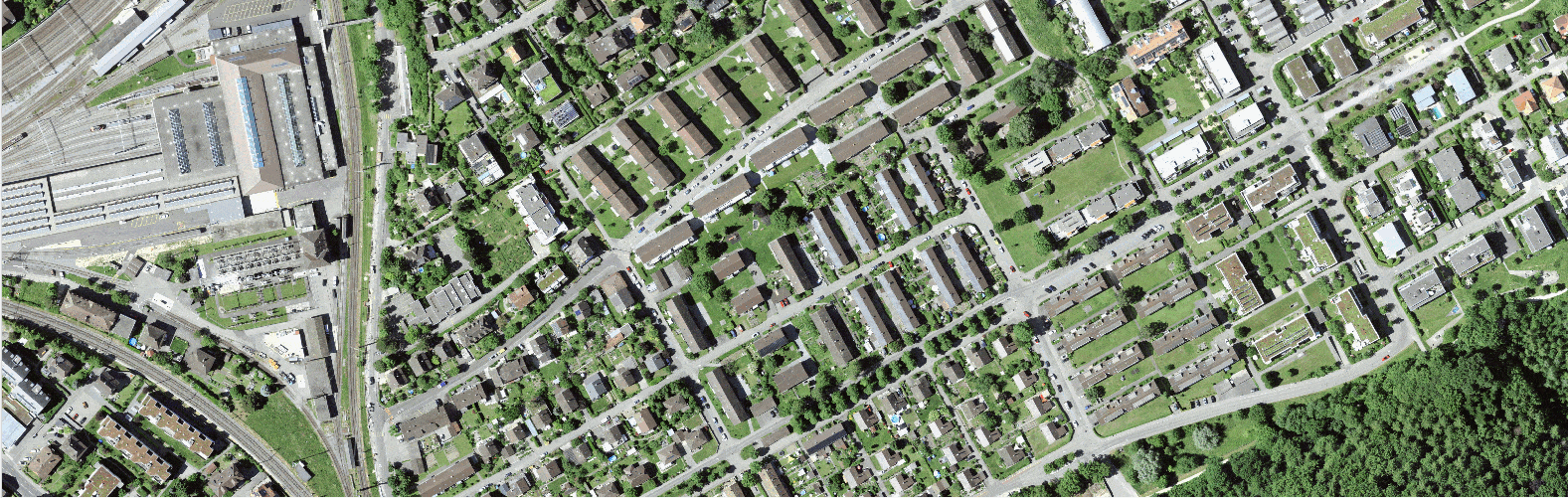
Super Resolution with SR3 backbone
Super Resolution is a set of techniques used to enhance the resolution of images. In ArcGIS, it is available as a model type for you to build and use models to artificially enhance the resolution of your images. The SR3 is a new backbone model for the Super Resolution model type. It adapts a denoising diffusion probabilistic model (DDPM) to conditionally generate images and performs super-resolution.
The animated gif below shows the low-resolution input image and the output of the Super Resolution and the SR3 model.
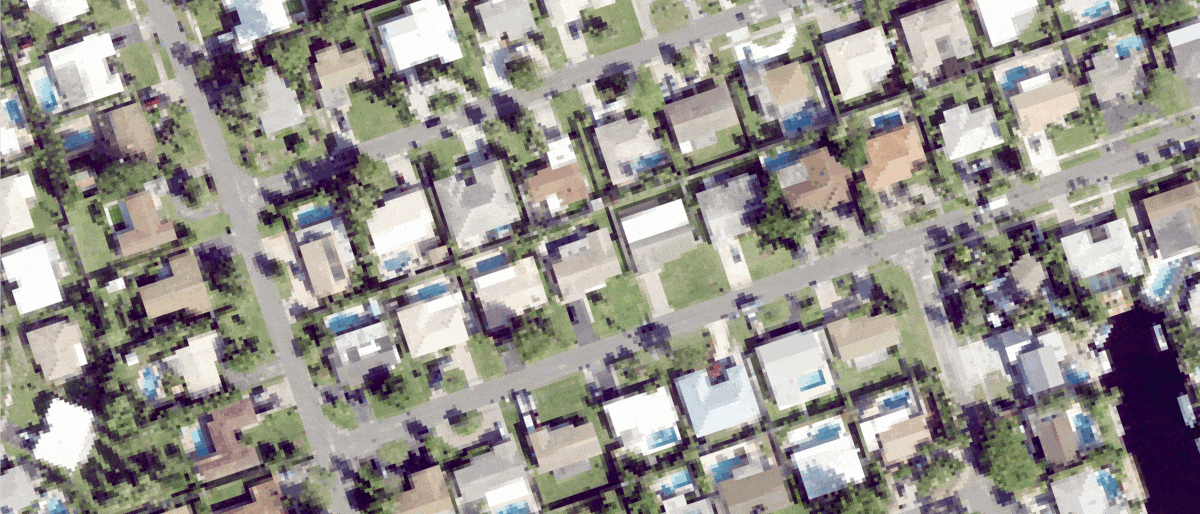
Model Assessment Report
Understanding deep learning models is essential before using them in inferencing. Reviewing a model gives you an indication of how it was trained and how it might perform. In many cases, you may have multiple models to compare. The Review Deep Learning Model pane is used to review the deep learning models that were trained and created. You can use this pane to generate a report on all the models that were trained. The report compares the model metrics to give you the most optimal models for each class being trained.
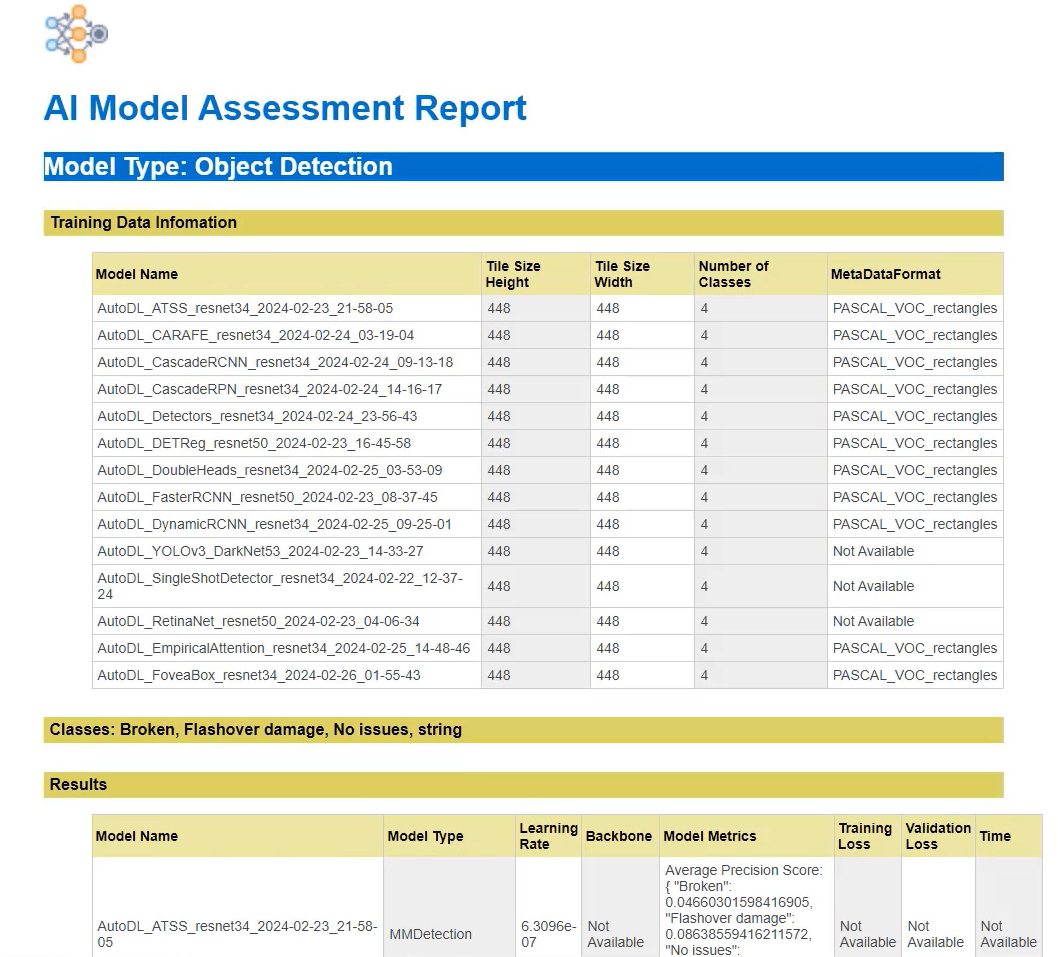
Multiple GPU support for inferencing
Starting from this release, all inferencing tools will use multiple GPUs, if available. To use a specific GPU, specify the GPU ID in the tool Environment. When the GPU ID is not set, the tools use all available GPUs. This is default.
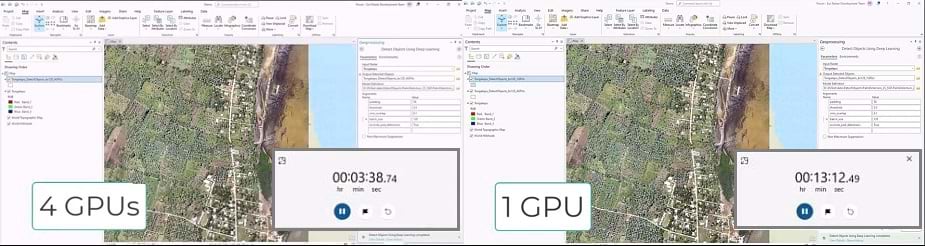
For further information, please visit the ArcGIS Pro page or the What’s New documentation and post your questions in the ArcGIS Pro board or ArcGIS Image Analyst board in Esri Community.
Commenting is not enabled for this article.