In 2019, The Midwestern Floods, AKA Great Floods, affected 14 million people in the Midwestern and Southern United States. To conduct an analysis of its impact, a flood mapping and landcover inundation was performed in St. Louis, Missouri, one of the areas most affected during the flood. Typically, optical imagery supports performing visual interpretation or identifying changes. However, optical imagery is not helpful when the areas of interest are cloud covered, as is the case in this scenario. This is where an alternative source, like radar, becomes effective.
Why Radar?
Synthetic Aperture Radar (SAR) data provides a consistent (unobscured by cloud) view of the properties and shape of the earth’s surface. Unlike optical imagery, SAR can be captured day or night and in any climatic condition, enabling frequent observations.
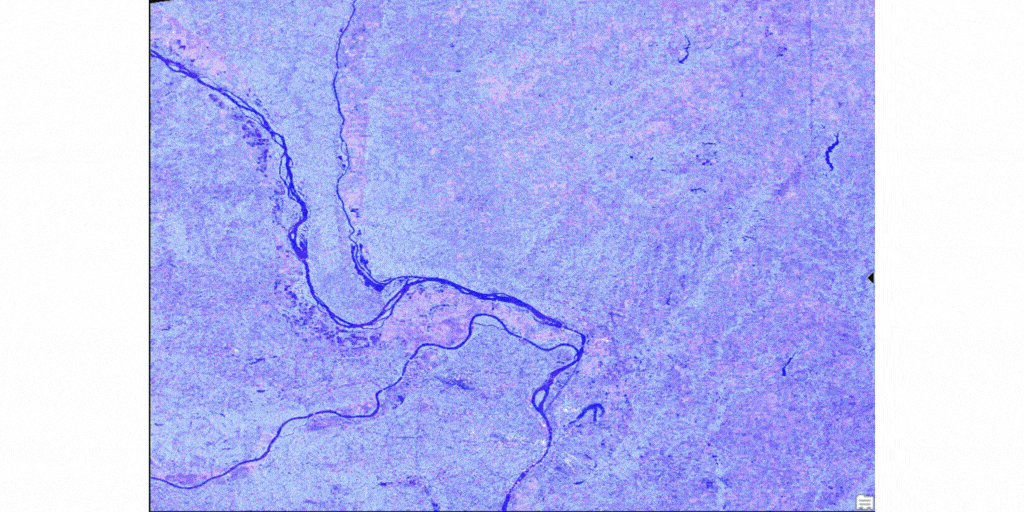
Create an Analysis-Ready Sentinel-1 Radar Product
To get to our analysis, we will first need to create a Sentinel-1 SAR analysis ready dataset using tools from our Synthetic Aperture Radar toolset. First, we start with a Level-1 Sentinel-1, interferometric wide (IW) swath mode GRD dataset, and a 10 mt resolution DEM.

- Download Orbit File and Apply Orbit Correction – Download and apply updated orbit data that is later used for terrain correction.
- Remove Thermal Noise – SAR images are distorted by additive thermal noise. The Remove Thermal Noise tool uses Sentinel-1 product metadata to correct thermal noise.
- Apply Radiometric Calibration and Terrain Flattening – Radiometric calibration is the process of converting SAR products from image pixel digital number (DN) to the physical quantity of SAR backscatter intensity per unit area. The Apply Radiometric Calibration tool uses the Sentinel-1 product metadata to retrieve meaningful backscatter values. The Apply Radiometric Terrain Flattening tool corrects artificial radiometric values originating from complex topography and the sensor’s viewing geometry.
- Despeckle – Inherent noisy anomalies characterized in SAR images is removed using the Despeckle tool. This process improves the signal-to-noise ratio of the SAR image.
- Apply Geometric Terrain Correction – The Apply Geometric Terrain Correction tool corrects geometric distortions, shifting the pixels to their correct geolocation.
- Convert SAR Units – The Convert SAR Units tool simplifies the data for interpretation by converting the units into decibels.
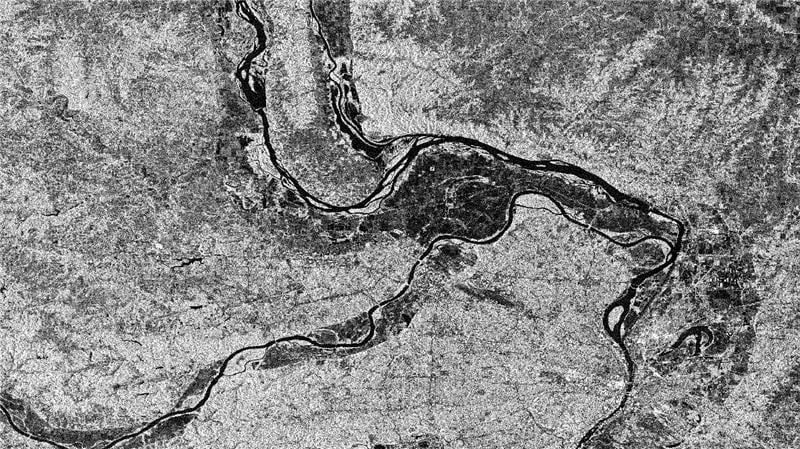
Analyzing the Processed ARD data using Deep Learning to Delineate Floods
Many of our functions work with this analysis ready dataset. Additionally, we have deep learning capabilities to explore these datasets and derive insights.
Using a deep learning model that was trained to delineate floods using SAR imagery and the processed Sentinel-1 ARD dataset, we can derive our flood map (a pretrained deep learning model is also available in ArcGIS Living Atlas of the World).
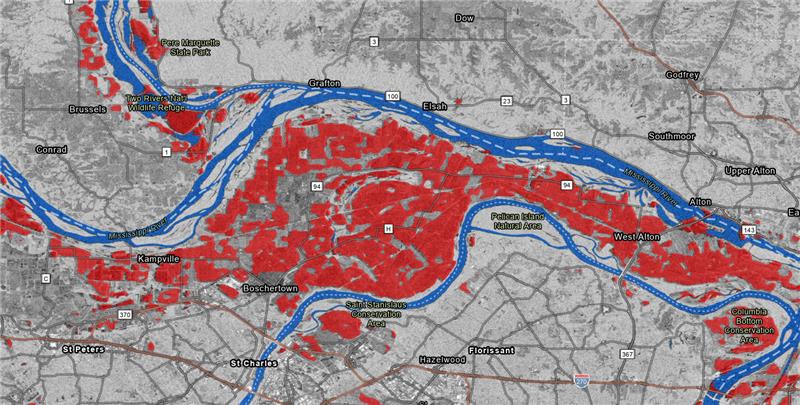
To get a better understanding of how land use was affected, Sentinel-2 imagery was used over the area of interest. Using a pretrained model available on ArcGIS Living Atlas of the World, you can generate a land use map and clip it to the extents of the floods. This now helps quantify the area of different landcover types that was affected by the floods. These insights help first responders prioritize post event operations. This describes an end-to-end workflow that highlights the new radar processing and analysis capabilities in ArcGIS.
And yes, the analysis aspects of this scenario are also possible in ArcGIS Online using ArcGIS Image for ArcGIS Online!
Check out the video below to see this workflow in action.
Resources
What’s new in the ArcGIS Image Analyst Extension (ArcGIS Pro 3.0)
Introduction to synthetic aperture radar
Article Discussion: