Digital transformation provides cities with ever-increasing ways to capture data—from connected streetlights to CCTVs to traffic flow and collision information transmitted from smart roads. But many cities fall short of their digital potential because they focus on collecting and using that data in narrow, traditional ways.
A truly smart city goes beyond these, improving quality of life and the climate for business by analyzing data in service of specific challenges and using it to make good decisions in real time on a range of critical issues, from public safety to open data to economic investment. Probing deeply into big data and pinpointing solutions to important problems demand a chief analytics officer (CAO), rather than a chief data officer (CDO). Both are essential to a smart city or smart business, but they are quite different.
Smart City, Efficient City
Smart cities use location-driven analysis and ground truthing (testing expectations by observing conditions on the ground) to understand where things are happening—even where they are likely to happen. This empowers government agencies to address issues more efficiently, even as it improves quality of life—for example, by helping safeguard firefighters or keeping bad landlords from forcing good tenants out of their apartments.
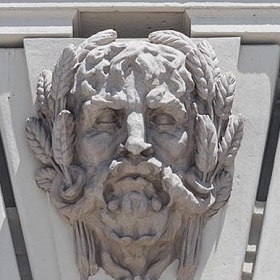
A 2016 Forbes article notes that while job searches for CAOs are growing quickly, the concept of a CAO remains so new that those who fill CAO positions must excel at explaining the function and value of their team.
In this case, I speak with firsthand knowledge. I was the chief analytics officer for New York City; it was—and remains—the only city with a CAO. In fact, the title and nature of the duties are so new that there are relatively few CAOs in the private sector, even though many businesses rely on analytics to increase operational efficiency and boost sales.
The company or city that understands the strategic capability that CAOs and their team can bring will gain an economic advantage through the ability to resolve pressing issues quickly. By efficiently untangling problems, a company can become more profitable and a city safer and more livable, prosperous, and attractive to residents, tourists, and new businesses. It is not at all surprising that several recent articles indicate that the number of CAOs in the private sector and the number of cities with analytics teams are starting to grow.
The First Mayoral Department of Analytics
When I had the honor of being chief of the Mayor’s Office of Data Analytics in New York City, the group consisted of about a dozen people who all had to learn how to sift through the big data produced by a city of 8.5 million people. For instance, we received an hourly feed from the city’s 311 system, which logged 100,000 contacts each day, and another feed from the 911 system, which averaged 30,000 calls a day.
We searched for trends that would help lower the risk of firefighters dying on the job, including the incidence of fire associated with different types of building permits. We also uncovered patterns that helped prosecutors convict unscrupulous landlords who were forcing residents out of rent-stabilized apartments. In both cases, we generated algorithms that accurately predicted locations where problems were likely to happen and used a geographic information system (GIS) to communicate those predictions on a map.
If your smart city is suddenly confronted with a health or safety or economic crisis and you need to find a solution—to find that proverbial needle hidden somewhere inside big data—you need a CAO and an analytics team to lead the way. Chief analytics officers and their teams are practical, discovery driven, and results oriented. Every project must conclude quickly and deliver clear guidance on what to do next.
Chief Analytics Officers versus Chief Data Officers
Smart cities, of course, are built on digitized data, which is increasingly derived from the Internet of Things (IoT). As storehouses of digital data grow, many cities and businesses have recognized the need for a chief data officer. Chief data officers are essential. They know how to collect and manage data.
But having massive amounts of well-organized data is something like having a landscape dotted with silos of corn and grain. They contain enormous potential for fulfilling many needs, but you must know how to use the raw materials—and where to look for what you need. A chief analytics officer turns an organization’s data into decisions that solve its problems, increase efficiency, and identify opportunities.
By way of illustration, consider this: a chief data officer might plot the known incidents of traffic accidents on a map to publicize the issue of road safety. A chief analytics officer would enlist location analytics—examining the day of the week, the time of day, traffic volume, weather, roadway surfaces, and more—to explain why those incidents are occurring and how they might be reduced.
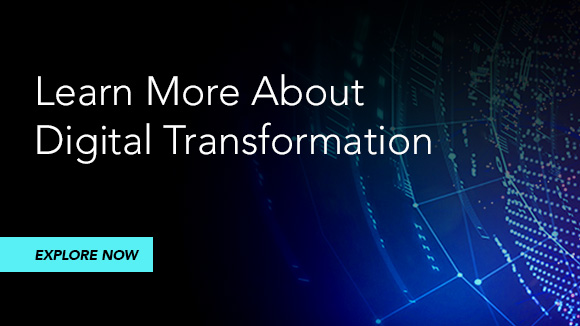
The analytics team always tells a well-documented story accompanied by specific ground truth recommendations—or, as we like to say, the do-these-things-now list.
Using Data to Stop Illegal Rental Practices
When unscrupulous landlords in New York City were forcing residents out of rent-regulated apartments to make buildings more valuable to buyers, it created several emergencies.
Those forced out of their units were hard-pressed to find similarly priced housing. Parents might be compelled to live much farther from their jobs, and children may have no choice but to change schools. Families might be pressured into residing in less safe areas. New Yorkers grew concerned about cruel practices that turned working families into economically stressed or even homeless citizens. If not countered, the problem could undermine the sense of security for tens of thousands of people.
Mayor Bill de Blasio asked us several questions: Where has this been happening? How is it happening? How can we predict where it’s <em>likely</em> to happen? and How can we stop it? Answers to pressing questions on topics like tenant harassment don’t just fall at your feet. And no algorithm existed to help us find ready-made solutions through artificial intelligence.
We knew we had to leverage location intelligence and GIS technology to mine our vast data for key indicators and uncover previously hidden trends. And before we offered information that could form the basis of enforcement activity or prosecutions, we had to ground-truth our findings.
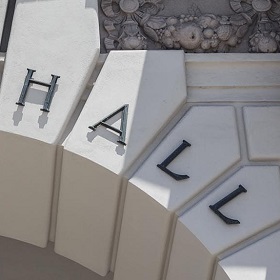
Smart cities use location-based analysis and ground truthing to understand where things are happening—even where they are likely to happen.
Location Intelligence Delivers Results
Housing and landlord data was scattered across several New York City departments. We had to talk to prosecutors, code enforcers, lawyers, residents, housing advocates, and others. But there was no time to have long discussions or wait to set up departmental presentations. Members of an analytics team must know what they’re looking for, what questions to ask, and what key traits and patterns to note.
We devised new approaches to finding the right data. We began by asking the question, Can we identify a timeline of harassment in city data? We found a tipping point: if a building that was on the market or had been sold recently lost five or more rent-regulated apartments, that was a red flag. Residents of rent-stabilized apartments pay rents much lower than market value. Unscrupulous owners use various methods of squeezing tenants out so that the landlords can rent the units for more money and make the building more valuable to buyers.
When we analyzed buildings that had reached that tipping point, we found complaints about landlords who, under the guise of renovating, were making apartments uninhabitable by removing stoves or refrigerators or by creating thick construction dust. Within weeks, we were able to predict where such harassment was happening or likely to take place. We did this by examining sales trends in terms of the following:
- Who was buying and where
- What the socioeconomic and demographic factors of the neighborhoods were
- What building sales were followed by extensive renovations
- Which landlords had a history of complaints against them
One common thread running through the data was location. With GIS, we correlated a cluster of critical factors—ranging from sales to landlord complaints—with locations on a city map.
Our analysis of the data allowed city prosecutors to zero in and secure more convictions. We had produced a dependable guide to uncovering trouble spots that relied, in part, on location intelligence. It even helped city officials send a general warning to those thinking about tenant harassment.
(Learn more about how local governments are unlocking opportunities in big data in this podcast.)
When Big Data Mining Saves Lives
After an NYC firefighter perished in a building fire caused by illegal structural changes and poorly installed electrical circuits, there was immediate concern about how widespread that problem might be.
Our analytics team could not rely on a simple analysis of code-violation locations—in a city of 8.5 million people, thousands of violations go undetected and unreported. Instead, the team focused on how to protect residents and firefighters. We wanted to know how code inspectors could help prevent fires by concentrating on areas where there was a high likelihood of dangerous violations, and how firefighters might be made aware of a high probability of life-threatening conditions when responding to a blaze.
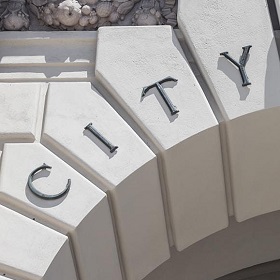
The CAO responds to problems raised by city officials, with the backing of a team of troubleshooters hungry to learn why an event is happening and how it can be fixed.
To home in on the illegally subdivided apartments, we had to find key factors buried in the data—and do it quickly. We used GIS to discern patterns of economic and demographic factors in neighborhoods, plus pertinent records of violations—that is, the locations of fires where illegal renovations were discovered or suspected. We then developed location intelligence algorithms that weighed these factors, sifted for key indicators, and predicted where we would find dangerous violations. We had to ground-truth our assumptions repeatedly, suggesting areas and buildings for fire officials to inspect. Sometimes, they turned up no significant violations, so we sharpened our algorithms and winnowed out the extraneous variables. Our early efforts to identify buildings with severe violations produced a 21 percent hit rate, but with repeated refinement, we improved that to 71 percent.
Ultimately, we were able to provide firefighters with a way to predict areas and buildings with a high likelihood of illegal, dangerous renovations. The algorithm improved safety for first responders, which is crucial to the ability of firefighters to effectively battle blazes.
Characteristics of Top Analytics Teams
There are no college degrees or majors dedicated to producing analytics officers. But we now know from experience what type of people you need to staff an analytics team that’s adept at going down unexplored paths in pursuit of answers.
In a crowded, complicated city like New York, where health, economic, or social problems can quickly multiply, data analysis cannot become a years-long doctoral thesis. It often is a life-and-death matter that demands quick answers. Getting things done in New York meant jumping in to attack problems that threatened residents’ health, safety, and quality of life, and analyzing those issues on the run.
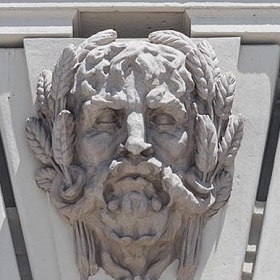
When a task force investigated NYC landlords who were forcing residents out of rent-regulated apartments to make buildings more valuable to buyers, the chief analytics officer rallied a team to address the problem, with the help of location analytics.
Members of a CAO team need to know how to talk with lawyers, police, firefighters, renters, landlords, doctors, nurses, and city officials in all manner of departments and bureaus. Those same people need to understand something of the ways that economics, social trends, and politics can affect the problem, and be willing to explore new avenues of inquiry.
The people doing this work for cities or businesses—especially the leaders of analytics departments—must be curious, quick on their feet, able to speak the language of other experts, creative in their approaches, aggressive in their pursuit of the right data, and able to use location analysis to bolster their findings.
When a smart city assembles such a team of problem solvers, the result is improved efficiency and money saved for taxpayers. In the case of the NYC firefighters and the illegally renovated buildings, these analytical efforts helped save lives and prevent injuries. I’m proud of that work. I know that many other cities can create similarly skilled teams and generate similar results.
Building Your Analytics Team
In addition to the curious, aggressive, flexible people I talked about above, smart cities really do need a strong data team, which begins with a good chief data officer. A CDO doesn’t compete with a chief analytics officer. They complement each other.
You can’t pull great answers out of poorly organized data. Your CDO, whether in the public or private sector, needs to make sure that the categories of data fit the organization and are readily accessible to those searching for answers to urgent problems. Citywide problems will cut across departmental lines, so the easier the access and the wider the data sharing, the better the outcomes.
Most companies and cities have huge stores of data, but that information may simply sit there, like volumes of neatly stacked encyclopedias. A skilled analytics team, led by a chief analytics officer, can draw on that data to improve efficiency in solving problems and strengthen decision-making.