Artificial intelligence (AI) is dominating discussions everywhere. Specifically, the rapid rise of generative AI—AI that can create new data, such as text, images, videos, audio, and 3D models—from platforms such as ChatGPT has led to a lot of debate about the ethics and quality of information used to train AI models. Discussions extend to predictive and insight capabilities, as well as whether to integrate these tools for automation in daily workflows. The combination of these questions and the concerning press coverage surrounding AI, including potential security threats, its influence on search engines, and its impact on employment, has created a frenzy.
GIS and geospatial technology are not immune to this discussion. AI and location intelligence have converged to create geospatial artificial intelligence (GeoAI). As explained in “Demystifying GeoAI” from the fall 2023 issue of ArcNews, “GeoAI fuses AI with geospatial data, science, and technology to accelerate workflows, uncover valuable insights, and solve spatial problems.”
When examining GeoAI and its potential benefits to geospatial professionals, it’s necessary to think about the potential dangers and harms that have been encountered by other AI models. For example, facial recognition algorithms have been notoriously unjust to Black women. The rapid evolution of AI and its widespread public use pose a scientific challenge: how to create generative AI without the same biases and damaging effects as general AI algorithms.
As we build and use GeoAI, we must consider who is training it. Who is building the user interfaces and for what kinds of users (for example, only for affluent white male users, or users of all genders, cultural backgrounds, technical abilities, and disabilities)? And how can toxins such as hallucinations be removed from AI?
In truly defining and evolving GeoAI, the scientific research community is seeking to discover whether the technology can do more than just automate tasks or answer geographic questions that we already know or can guess the answers to. Can it provide new insights such as predicting emerging spatial patterns, connections, events, or trends in human systems and nature?
I believe that GeoAI is capable of such feats. But to realize these achievements means that GeoAI needs to abide by the principles of digital resilience. Generally, resilience is the ability to deal with change and threats; to absorb disturbance, stress, and catastrophe; and to recover quickly from challenges and return to a desired state. We often hear about the necessity of environmental and climate resilience for our survival on Earth, considering the effects of people’s influence on the atmosphere, biosphere, and geosphere. However, digital resilience is also a key factor.
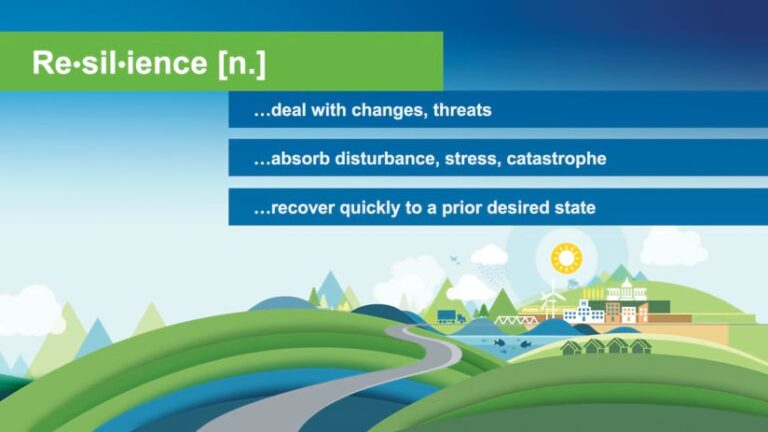
Given GeoAI’s potential to bolster community resilience by providing data and evidence-based advice, it’s essential to ensure that the geotools, their related approaches, and the data they are based on are digitally resilient as well. Digital resilience means that, to the greatest extent possible, data and tools should be freely accessible, up-to-date, high-quality, interchangeable, and operational. This way, the communities or entities using them can be resilient.
Moreover, given the destructive power of natural disasters caused by human-induced climate change, it is essential that geotools keep pace and be a critical part of 21st-century mapping. If we want to create a more resilient world, we need to ensure more resilient digital technologies. This includes the most promising and yet the most disruptive digital technology of all: GeoAI.
Here are five fundamental principles of digital resilience. In several blog posts, journal articles, and talks (some cited in the sidebar), I’ve posed up to eight. And of course, many more are possible.
- Making data and code “available” is not enough. If GIS professionals are making data open to access, especially in the name of open science, we need to be transparent about what we are doing with our data and our code, including how it was trained as part of a GeoAI model. Transparency can be achieved by sharing the workflows done with the data in a “virtuous chain” or “virtuous cycle.” This chain of GIS processes allows one result to build on the preceding one, leading to continuous improvement, and can be done using tools such as ModelBuilder scripts and models, ArcGIS and other Jupyter-flavored Python notebooks, and even various kinds of process logs. This is akin to sharing scientific methods—especially if rigor is important, since repeatability of an experiment, approach, or algorithm is a hallmark of the scientific method. Can a GIS user replicate the workflow with GeoAI? Can the user reconstruct and understand the scientific process that was used?
- Integrate via interoperability. To ensure digital resilience, we as a GIS community must foster interoperability by utilizing tools that can be translated into other formats. For example, we can use a Python environment that’s suitable for scientific computing, we can integrate with scientific tools and software libraries made by the community, or we can incorporate deep learning GeoAI models. No matter the role of GeoAI, following FAIR principles is the best way to enable interoperability. This means that geospatial data is findable when described by rich and accurate metadata, accessible when shared in a multitude of machine-readable formats, interoperable when easily published and shared using international open data standards, and reusable when released with clear and accessible data usage licenses.
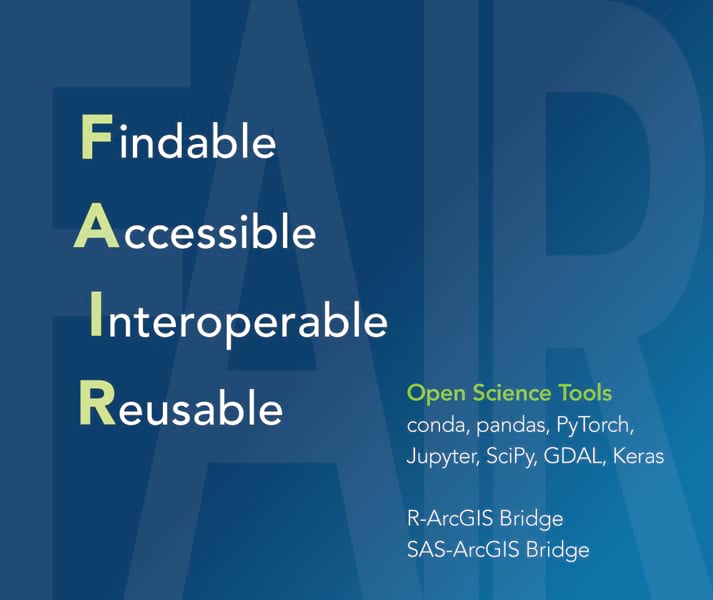
- Ease leads to exposure, and exposure leads to adoption. This is especially the case with easy-to-use apps that work on any device, anywhere, at any time. Apps are a great way to practice true interoperability. Such apps can expand GIS and GeoAI concepts, approaches, and code to appeal to broader audiences. Students are embracing apps in droves, as demonstrated by a popular computational art course at Santa Barbara High School that was highlighted during Computer Science Education Week. Courses such as this could spur increased app development. Some see this as an advantage, arguing that geography, GIScience, and data science instruction should teach students to be creators of software tools, not just users. Plus, thanks to the emphasis on app building in these courses, more young women and people of color are being drawn to the tech industry. Greater exposure and adoption can also be accomplished by following self-guided tutorials online, such as this one from Esri.
- Consider ethics, empathy, equity, and explainability. This subject is worthy of its own article or even a whole college course. In short, the goal is to develop responsible GeoAI, build trust, and shape policy. Users and developers of GeoAI must consider ethical issues such as location privacy and cartographic integrity alongside issues of empathy such as data representativeness and proper inference. (For details, see the last resources in the sidebar.) Developers of responsible GeoAI must also consider equity issues such as the use of geospatial technologies for social justice and democratizing access to these technologies. Ensuring that GeoAI is equitable means that AI must be explainable, with a set of processes and methods that allows human users to comprehend and trust the results and output created by machine learning and deep learning algorithms. Further, explainable AI should check GeoAI models and training data during the planning stages to make algorithms more responsible before the results are computed. It should also assess GeoAI results after they are computed.
- Promote a culture of sharing, engagement, and collaboration. This means enabling people to securely share data, apps, and GeoAI tools across all teams and networks and helping people understand their role in and use of GeoAI. Furthermore, this is not just a stated principle or a onetime occurrence; it’s a critical, natural part of how people do their GIS work every day, and it stems from their attitudes about that work. Importantly, people in an organization should be regularly rewarded—with praise, recognition, and promotions—for embracing digital resilience. They should also be encouraged to publish or speak about how these principles of digital resilience have been implemented.
At Esri, we acknowledge the important role that GeoAI will play in the future of GIS, and we choose to embrace it. But we want to do it with good understanding, responsibility, and transparency. Ensuring the longevity and effective use of the data, tools, and platforms that we develop is a critical part of our work. This is an undertaking that involves the whole geospatial community, not just Esri.
Let’s all leverage these new advances in geospatial science and technology to build greater digital—and, ultimately, community—resilience to the shocks and disruptions that will occur in a world facing accelerating change. If we want a resilient world, we need to start with resilient data.