When wildfires ravaged Lahaina, Hawaii, the scars left behind weren’t just physical but emotional and societal. The subsequent challenges of conducting a damage assessment helped underscore a pressing need for improved tools and methodologies. While traditional methods have their merits, the scale and severity of such disasters demand something more efficient. Therefore, we are excited to announce a new damage assessment deep learning model.
Historically, in the wake of a disaster, conducting a damage assessment has been a manual and time-consuming endeavor. Damage assessment teams need to cover vast areas, often under hazardous conditions, to document and assess damage. This not only elongates any potential response times but also can sometimes result in inconsistencies due to the sheer scale of the disaster.
Esri’s deep learning model was designed with the primary goal of addressing these challenges. By processing high-resolution satellite and aerial imagery, the new damage assessment model can identify patterns of destruction, differentiating between damaged and undamaged structures. This automation speeds up the assessment process with surprising accuracy. This blog will provide a high-level overview of the workflow and detail where you can locate the model to start using it yourself.
Practical Application in Lahaina
In the wake of the Lahaina wildfires, we developed and put to the test a new damage assessment model. The results were outstanding. What would traditionally take hours of labor and manual surveying was achieved in a fraction of the time with 95 percent accuracy. Rapid damage assessments with this level of accuracy revolutionize postdisaster assessment processes by providing access to previously unobtainable decision support information during initial response. For instance, with rapid and accurate damage assessments, authorities can prioritize areas for search and rescue and allocate resources to heavily affected areas immediately.
To classify damage, it is necessary to have building footprint information for the area of interest. This information allows the damage assessment model to classify buildings as damaged or undamaged by creating boundaries from which to reference and label.
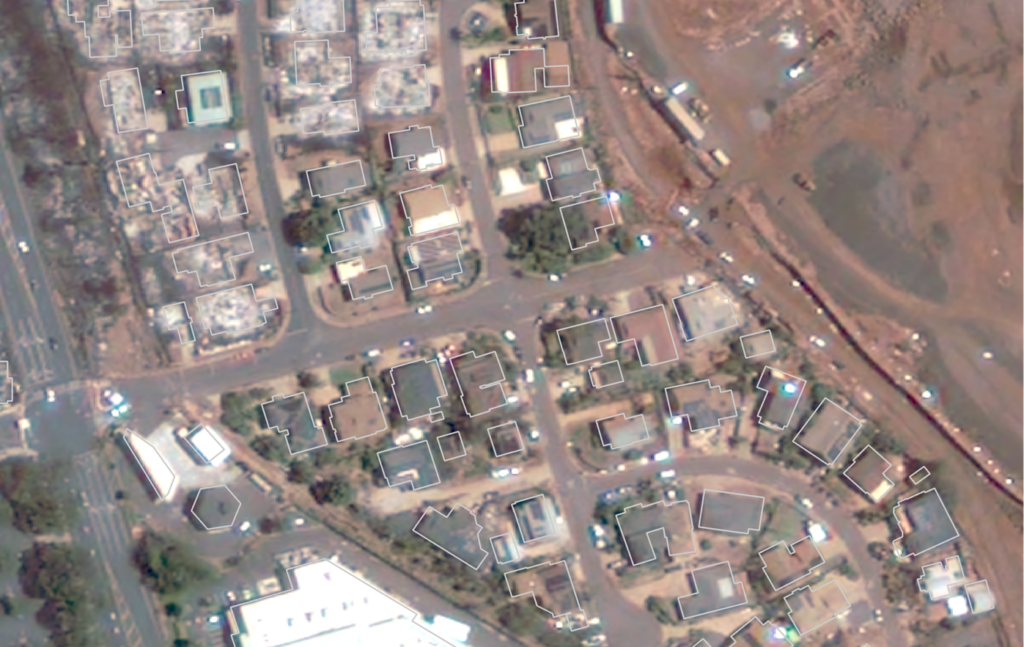
The accuracy of the building footprint layer is also important and may vary depending on the method used to create the data. The building footprints should be accurate to the incident imagery you are planning on utilizing to run the model. If building footprints don’t exist, the user needs predisaster imagery so that they can use different AI models to extract building features. To provide additional context for the buildings, the building footprint data we utilized also indicated building occupancy type. This allows the model to help quantify the number of homes and other infrastructure that may be damaged.
For help in extracting building footprints from imagery, Esri offers a high-resolution model in ArcGIS Living Atlas of the World: Deep Learning Model to Extract Building Footprints
After obtaining building footprint information, the next step is to locate postdisaster imagery for the affected area. Postdisaster imagery can come from a variety of sources including satellites or unpiloted aerial system (UAS) technology. Obtaining postdisaster imagery from a satellite sounds difficult or prohibitively expensive. In truth, neither is the case! The resources available via the links below will help you learn about tasking satellites and how easy it can be:
Another great resource for postdisaster imagery is Maxar’s Open Data Program at https://www.maxar.com/open-data.
Once postdisaster imagery is obtained, it is best to refine the deep learning model. For Lahaina, we manually inspected 500 buildings from the postdisaster imagery, classifying them as either damaged or undamaged. This helped refine the model to different geographies, account for differences in building size and shape, and incorporate different extents of damage into a model’s output. In short, we took our postdisaster imagery and conducted a drive-by or windshield survey to help the model classify buildings.
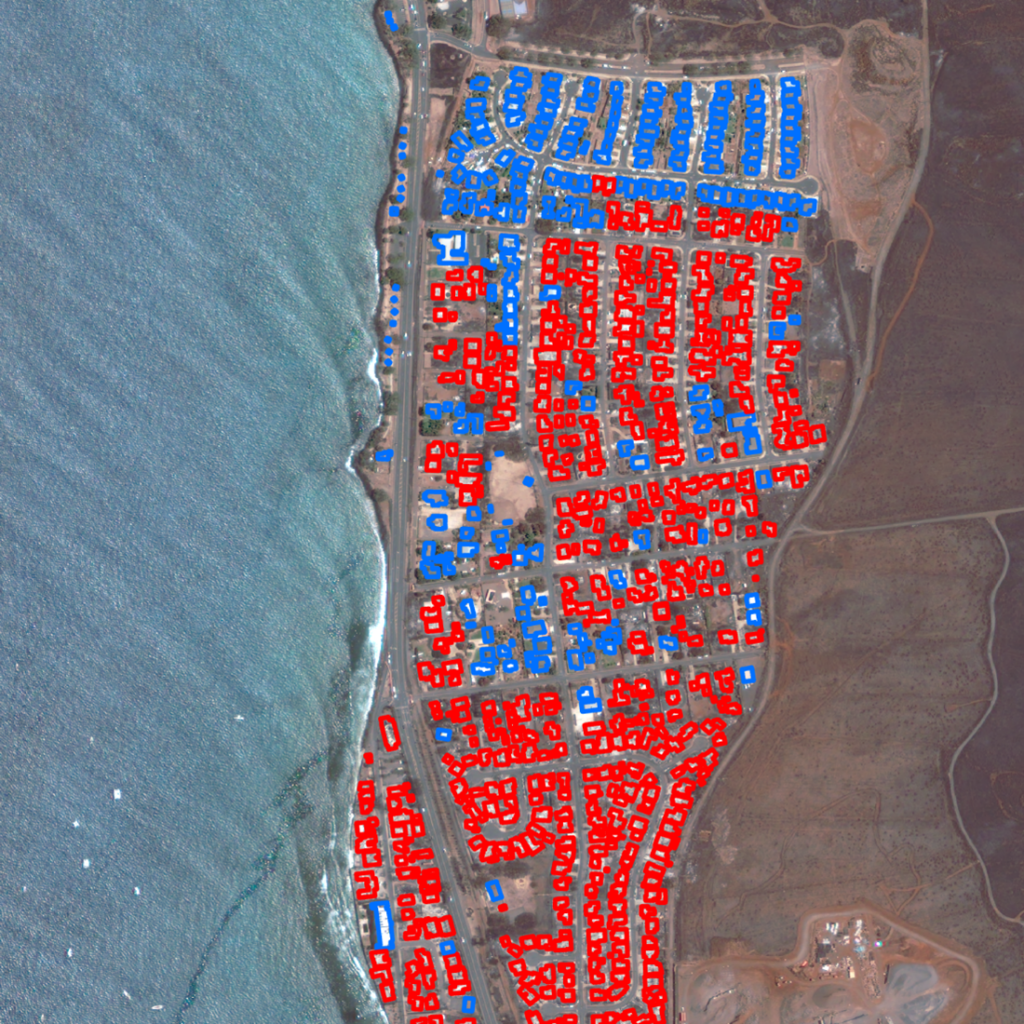
Once the model is refined, users can use ArcGIS Pro or ArcGIS Online to run the deep learning model and produce their own damage assessments. Esri published both the results of the analysis and the deep learning model in ArcGIS Online in hopes of spurring efficient collaboration, creating new tools for incident response, and democratizing geospatial artificial intelligence (GeoAI).
The damage classification model is publicly available here: Esri Damage Assessment Model for Download
The model’s results can easily be placed into a web map, dashboard, or web app, depending on the user’s need. In the case of the Lahaina fire, a dashboard was chosen so that the results could be visualized and organizations could use it to help guide response and recovery efforts as well as inform public outreach.
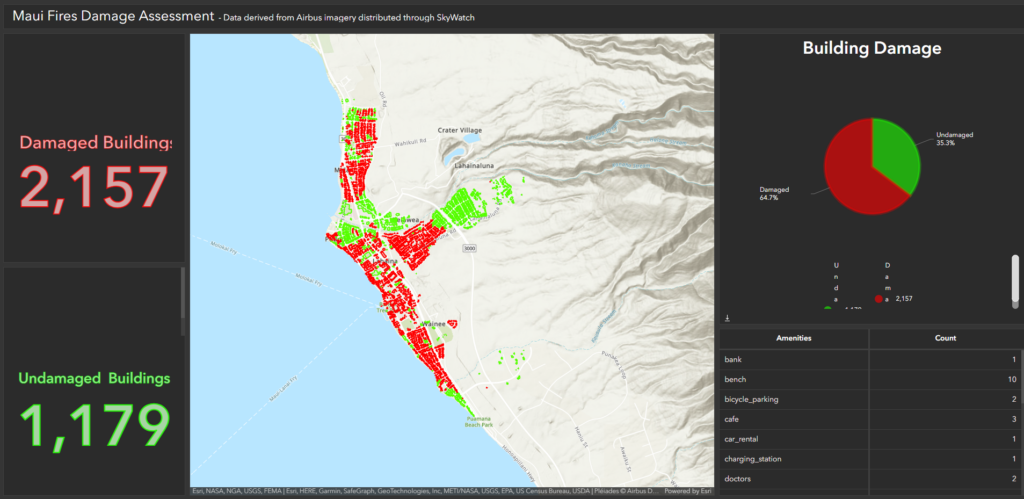
The Way Forward
The tragic wildfires in Lahaina served as a stark reminder of the vulnerabilities we face and the importance of timely and accurate postdisaster assessments. Like most artificial intelligence applications in wildland fire, deep learning is not a replacement for on-the-ground verification and expertise but rather a tool that augments human capabilities.
As wildland fire and disaster management continue to evolve, the blending of these models with traditional methods will only serve to refine our disaster response mechanisms. Regardless, as technology continues to progress, the goal remains steadfast: to support communities in their time of need, ensuring that they have the best tools at their disposal for disaster preparedness, mitigation, response, and recovery.
Additional Resources
Below are the resources linked within the blog as well as further data and information from the Federal Emergency Management Agency (FEMA) Geospatial Resource Center specific to the Lahaina fire: