In a world more rich with data than ever, what if the true challenge and strength lie not only in its use, but also in the power to derive new data to serve our evolving needs? Land cover classification exemplifies this challenge as a complex exercise that is difficult to perform using traditional remote sensing techniques. Esri’s library of pretrained deep learning models is here to help!
Available on ArcGIS Living Atlas of the World, you can take advantage of a collection of pretrained models for deriving land cover maps from high- to low-resolution imagery. These models are designed to extract meaningful insights from imagery without requiring extensive training data, significant computational resources, or extensive artificial intelligence (AI) expertise.
The land cover maps generated by these models, which describe the physical land types on the Earth’s surface, are useful in urban planning, resource management, change detection, environmental monitoring and similar workflows.
Esri’s expanding library of pretrained deep learning models helps you streamline your geospatial workflows. You can use these pretrained models to optimize workflows like building footprint detection, damage classification, land cover classification and more…
Pretrained models for land cover classification
Deep learning models are highly capable of learning and characterizing complex landcover semantics to produce high-quality land cover maps. Land cover maps generated from different satellite imagery sources like NAIP, Sentinel, and Landsat serve different workflows primarily due to differences in spatial resolutions and sensor revisit periods. In our library of models, we’ve provided a collection of models that are focused on deriving land cover maps from these different imagery sources. Here’s more information on the models we’ve provided, with more on the relevant use cases.
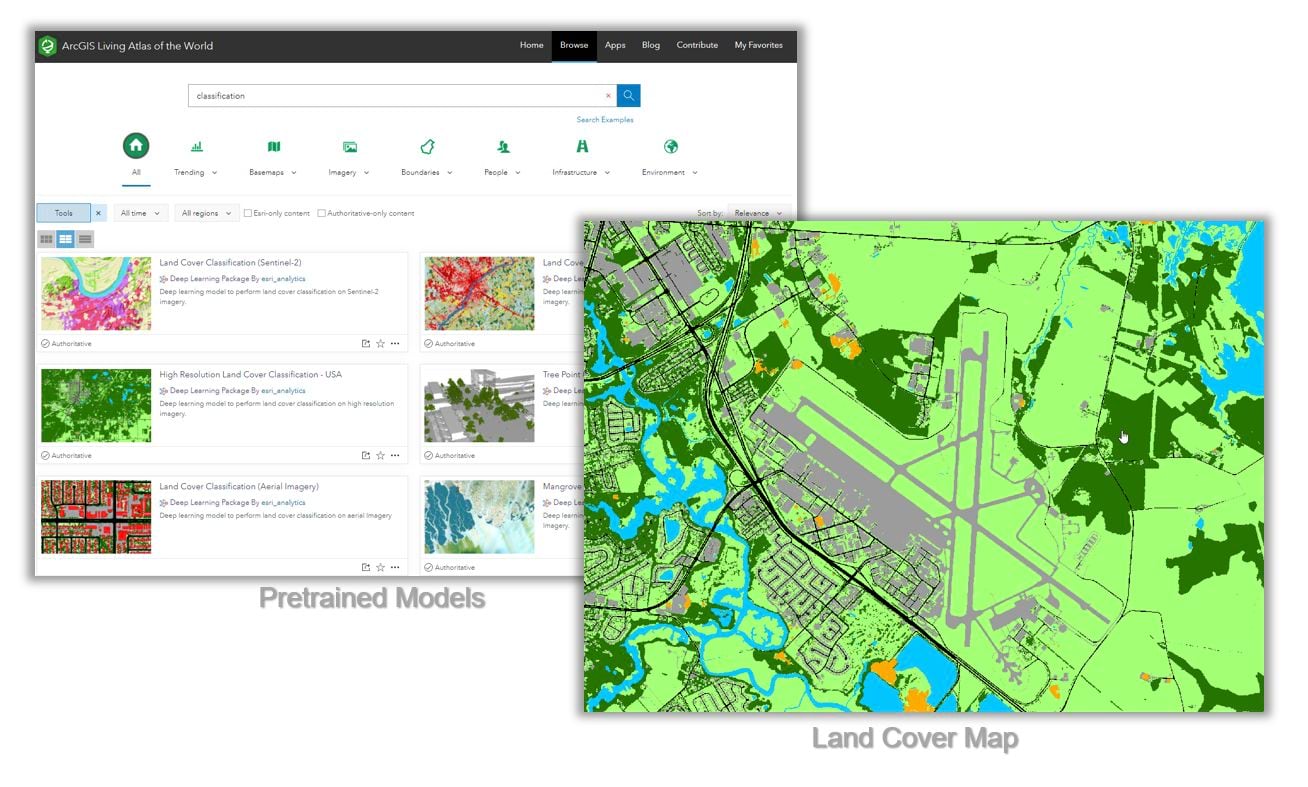
High-Resolution Imagery
While several agencies, such as the United States Geological Survey (USGS), United States Department of Agriculture (USDA), and National Aeronautics and Space Administration (NASA), have produced land cover maps for the entirety of Europe and the USA with lower spatial (e.g. 30 meter) and temporal (e.g. every 1-5 years) resolution, there remains a gap in generating real-time land cover maps at finer spatial resolution, such as 10 centimeters, for specific regions. High Resolution Land Cover Classification – USA and the Land Cover Classification (Aerial Imagery) are LULC models tailored for such high-resolution data. These models produce land cover maps from high-resolution imagery, creating essential insights for applications such as monitoring crop types, evaluating crop health, infrastructure planning, tree species identification, assessing vegetation condition, and natural resource monitoring for diverse ecosystems such as wetlands, forests, grasslands, and other habitats.
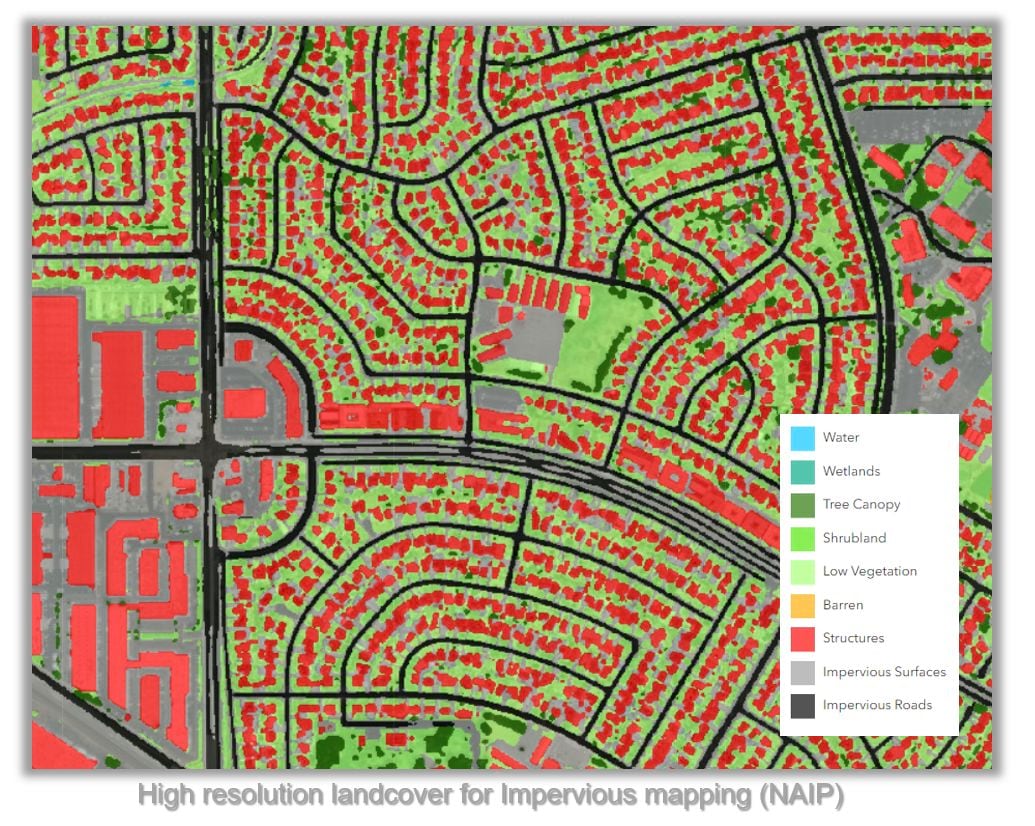
Medium-Resolution Imagery
The Land Cover Classification (Sentinel-2) model is specifically designed for Sentinel-2 imagery. At 10m resolution, landcover maps from Sentinel-2 satellite imagery are instrumental for environmental monitoring (tracking vegetation, water bodies, and detecting hazards) and disaster management (providing timely disaster information, including floods and landslides) as well as aiding land use planning by offering insights into land cover changes and urban expansion.
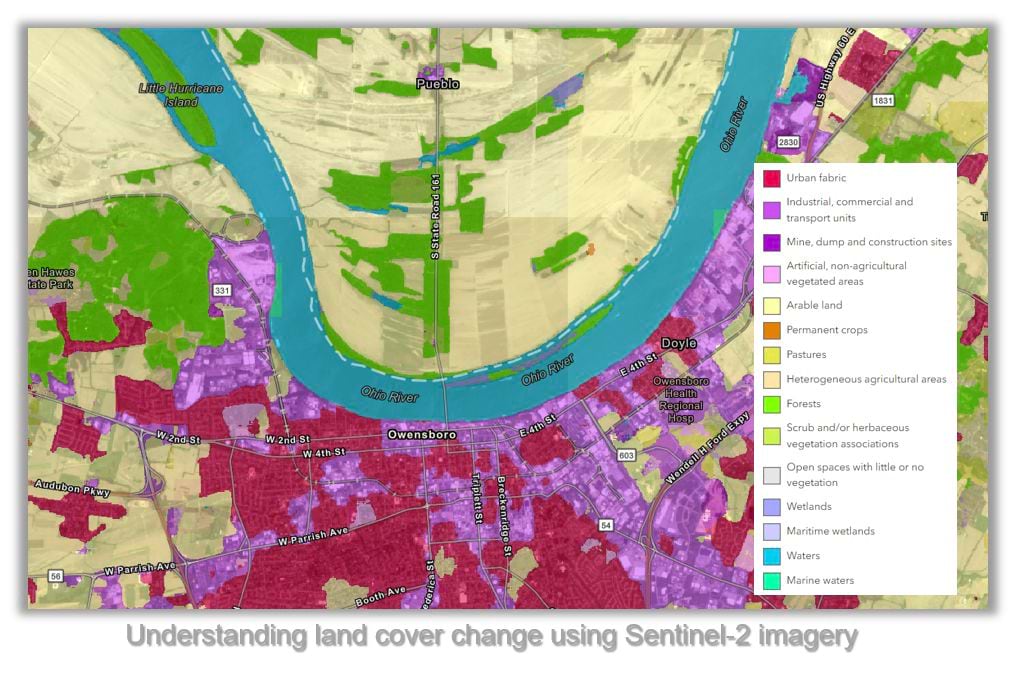
Low-Resolution Imagery
The Land Cover Classification (Landsat 8) model is an indispensable resource for producing land cover maps on Landsat 8 imagery. Trained on the 2016 National Land Cover Database (NLCD) and matched with corresponding Landsat 8 scenes. At 30m resolution, landcover maps derived from Landsat 8 satellite are ideal for long-term analysis and resource management. They are applied in land cover change analysis, resource management (forests, water bodies, and agricultural lands), and climate change studies by quantifying changes in carbon storage, albedo, and land surface temperatures.
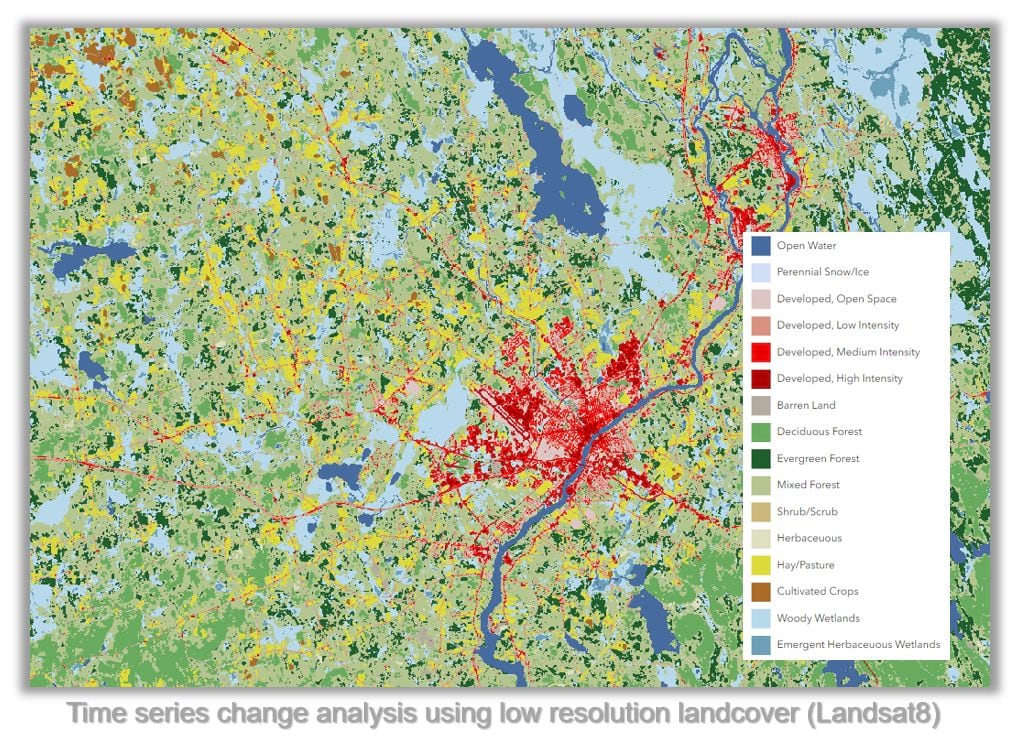
In summary, the choice of satellite imagery sources and the utilization of pretrained DL models for land cover mapping can be aligned for specific applications and requirements.
Land cover maps, complemented by additional data layers available in ArcGIS Living Atlas, provide a holistic picture of both the human and natural footprint on the planet.
Next steps
Dive into the world of pretrained deep learning models in ArcGIS Living Atlas and discover their immense potential. Learn how to use these models effectively within ArcGIS with our detailed instructions in Introduction to ArcGIS pretrained models. If you have any questions or insights about their performance or ideas for additional AI-powered feature extraction tasks, join the conversation at Esri Community. Your feedback and ideas are the cornerstone of our innovation, driving us to broaden our horizons and expand our offerings.
For more detailed use cases, please refer to the examples provided below.
References
https://livingatlas.arcgis.com/landcover/
https://medium.com/geoai/high-resolution-land-cover-mapping-using-deep-learning-7126fee571dd
https://learn.arcgis.com/en/projects/classify-mangroves-using-deep-learning/
https://developers.arcgis.com/python/samples/measure-shrinking-lakes-using-deep-learning/
Article Discussion: