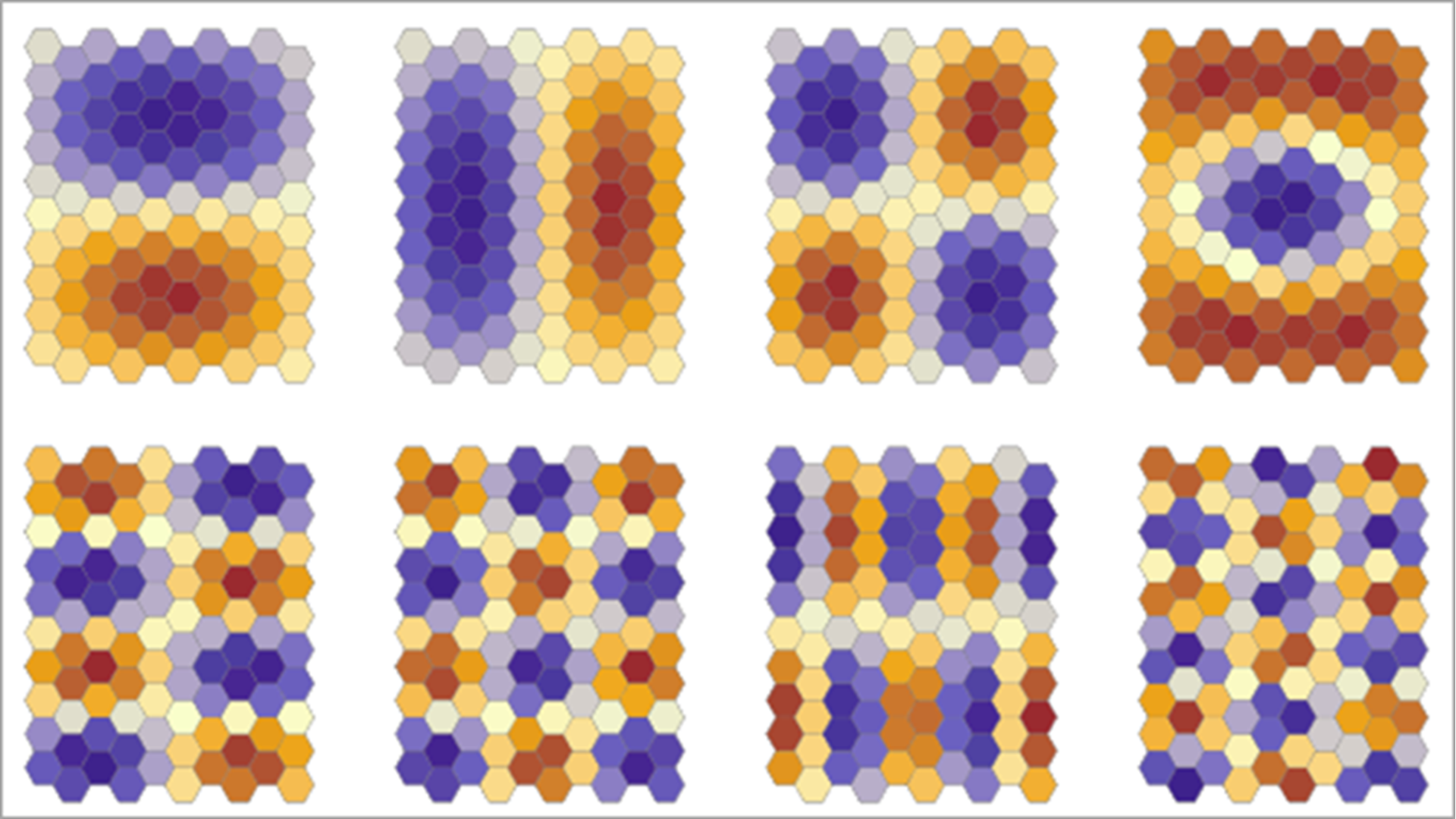
New in ArcGIS Pro 3.4, the Spatial Component Utilities (Moran Eigenvectors) toolset includes four tools that each utilize spatial components (also called Moran eigenvectors) to help with various spatial statistics workflows.
Spatial components are constructed by decomposing a spatial neighborhood (also called a conceptualization of spatial relationships) into a series of components that represent various possible spatial patterns of spatial variables. The first several components usually represent broad spatial trends (such as east-west or north-south trend), and later components represent more local patterns. The following image shows various spatial components of a hexagonal tessellation:
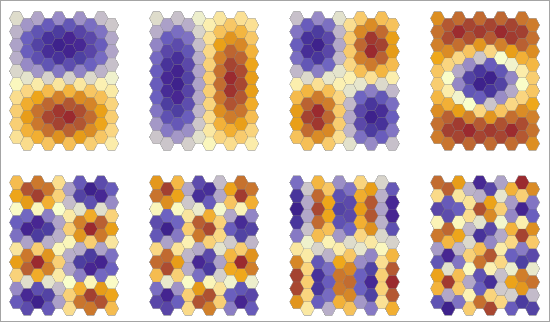
You can combine these components together in various ways to represent more complicated spatial patterns of your data. For example, if a spatial variable has a broad west-to-east trend but also contains small clusters of low and high values, the spatial pattern of the variable could be represented by combining two components: one representing the west-to-east trend and the other representing the clusters.
Learn more about spatial components.
The four tools in the new toolset each employ spatial components to help with different spatial statistics tasks:
- Compare Neighborhood Conceptualizations—Suggests a neighborhood and weighting scheme that most accurately represents the spatial patterns of one or more fields of a feature class. The output is a spatial weights file that can be used in other tools in the Spatial Statistics toolbox, such as the Bivariate Spatial Association (Lee’s L), Hot Spot Analysis (Geti-Ord Gi*), and Cluster and Outlier Analysis (Anselin Local Moran’s I) tools.
- Create Spatial Component Explanatory Variables—Creates and selects a set of components that can be included as explanatory variables (in addition to other explanatory variables) in a prediction model. The selected components best represent or explain the spatial patterns of multiple analysis fields and allow you to improve the model by accounting for the spatial patterns of the variables.
- Filter Spatial Autocorrelation From Field—Removes the spatial autocorrelation from a field by separating it into spatial components and a nonspatial component (the spatially filtered version of the input field). The filtered field maintains the core statistical properties of the field while factoring out spatial effects, such as trends and clusters.
- Decompose Spatial Structure (Moran Eigenvectors)—Creates the set of components that have the largest possible Moran’s I value for the spatial neighborhood. The components created by the tool can be mapped to visualize the various spatial patterns.
To see how these tools can be integrated into larger workflows, see the following blogs:
Article Discussion: