2023 will be remembered as the year in which AI took center stage. With today’s AI models, computers can now see and perceive, read and comprehend, analyze and interpret, and even generate human-like text and art.
Pretrained AI models in ArcGIS have democratized geospatial AI (GeoAI) and made it more accessible than ever. These models are suited to perform a variety of AI tasks to address different business needs, such as extracting building footprints for urban planning, classifying structures for insurance purposes, generating change maps from time series data, or creating a pervious map for taxation purposes. These models also serve as an excellent starting point for you to fine-tune on your local geography or features of interest. These pretrained AI models unlock productivity in so many areas of GIS, helping automate data extraction at scale and uncover valuable insights faster than ever.
This year, our library of pretrained models has doubled from 30 to nearly 70, and they’re getting more popular by the day! Join us on a journey to explore these cutting-edge models and discover the possibilities they offer in the realm of geospatial AI.
Here is a compilation of our latest models that apply to different business sectors:
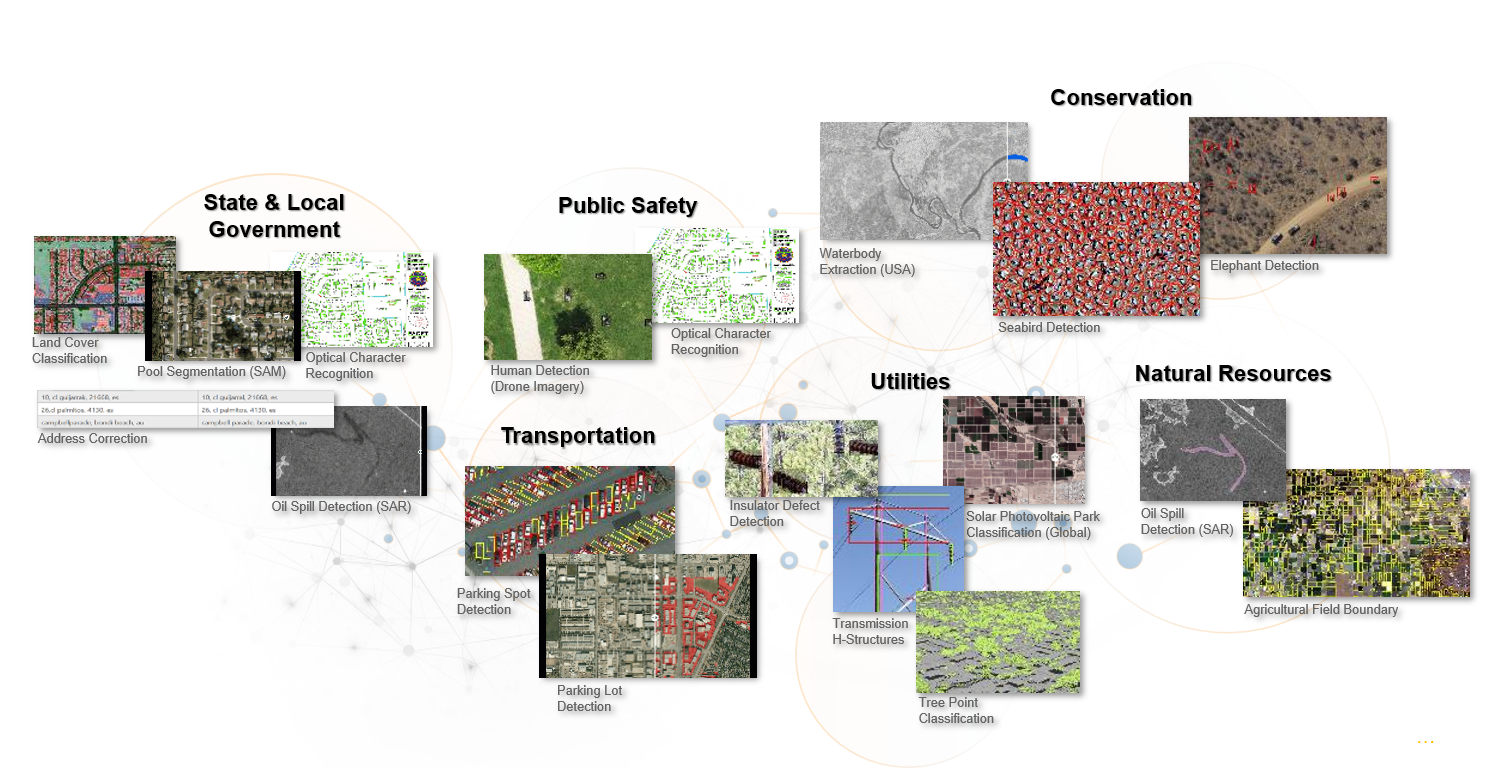
Transportation
Using AI in transportation improves efficiency, safety, and sustainability. In addition to the problem sets below, AI helps in developing predictive maintenance systems to create a more streamlined and resilient transportation ecosystem. A couple of models we introduced this year to streamline workflows for this sector are:
Parking Lot Classification — USA
Parking lots occupy significant land areas, particularly in urban and suburban areas. By installing solar panels on parking structures, it is possible to utilize parking spaces for solar energy generation without requiring additional land. This not only provides shade for parked vehicles but also generates clean energy. Automating the task of parking space classification and solar panel capacity calculation using AI models offers numerous benefits, including improved efficiency, accuracy, scalability, adaptability, real-time monitoring, and integration with renewable energy goals. To view model results, see this story.
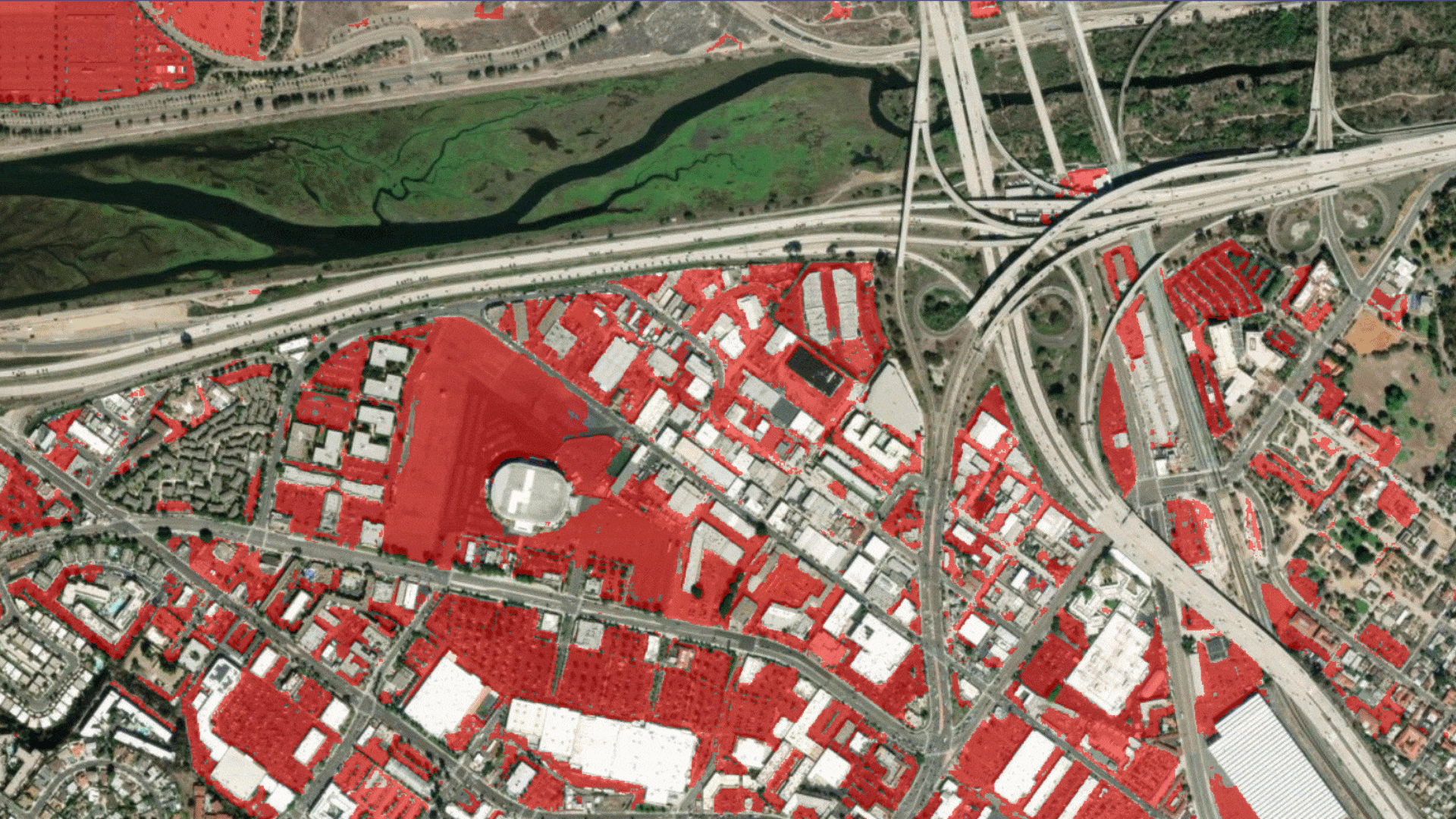
Parking Spot Detection—USA
Parking spot detection can enhance urban mobility, reduce vehicular emissions, and reduce congestion by providing real-time information on available parking spaces. Additionally, it serves as a valuable proxy for economic activity, as higher demand for parking spaces often correlates with increased commercial and residential activities in a given area.
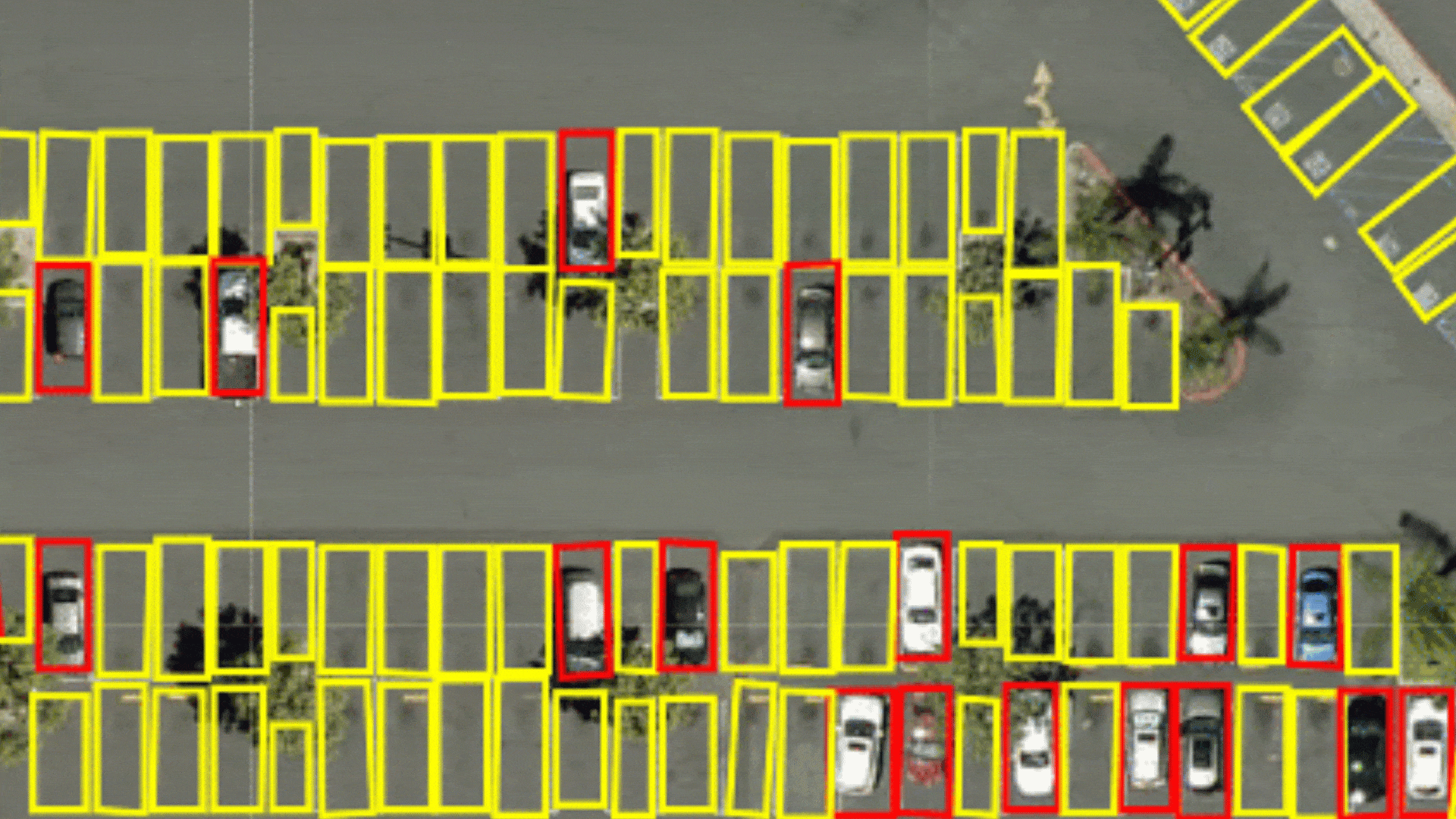
Natural resources
In the natural resources sector, AI is instrumental in resource exploration, extraction, and sustainability. AI-driven automation enhances and expedites decision-making, aids in sustainable resource management, mitigates environmental impact, and ensures responsible utilization of natural assets. We’ve released four new models to support this sector.
Plant Leaf Disease Classification
Fruit and vegetable planters can prevent the spread and severity of infestations, minimizing crop losses and maximizing yields, by detecting plant diseases early. This model enables planters to take appropriate preventive measures against diseases by accurately classifying different categories of plant infestations from leaf images. Manual identification of plant diseases is time-consuming and challenging, making this automated approach invaluable. This efficient identification of plant diseases aids in the implementation of suitable pest control measures, ensuring the health and productivity of fruit and vegetable crops.
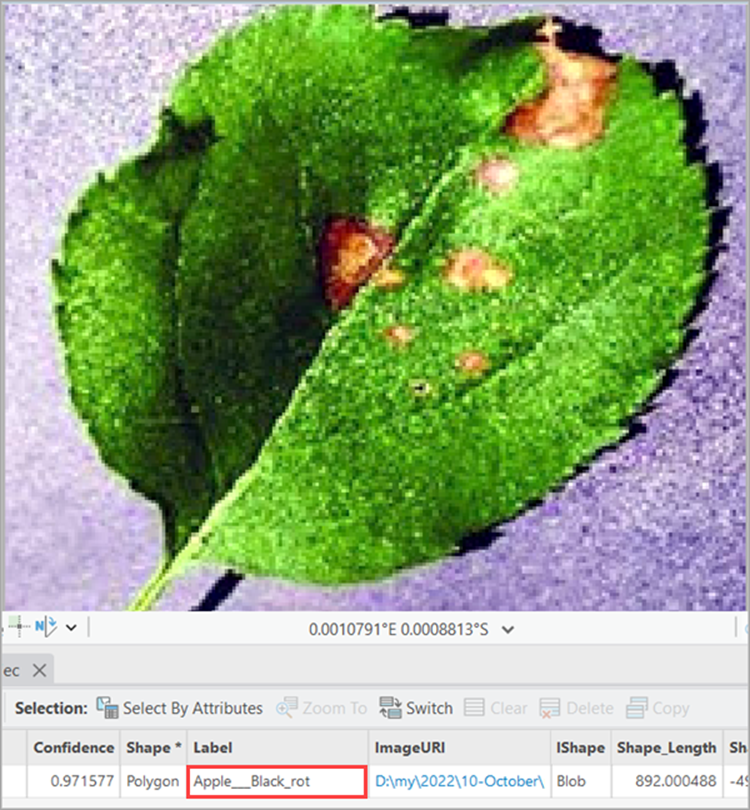
Tree Segmentation
Tree segmentation can be used for applications such as vegetation management, forestry, urban planning, and so on. High-resolution aerial and drone imagery can be used for tree detection because of its high spatiotemporal coverage. This AI model integrates the DeepForest open-source model with Meta’s Segment Anything Model (SAM), internally combining their capabilities to not only identify the location of trees but also delineate their spatial extent, offering valuable data for vegetation encroachment analysis.
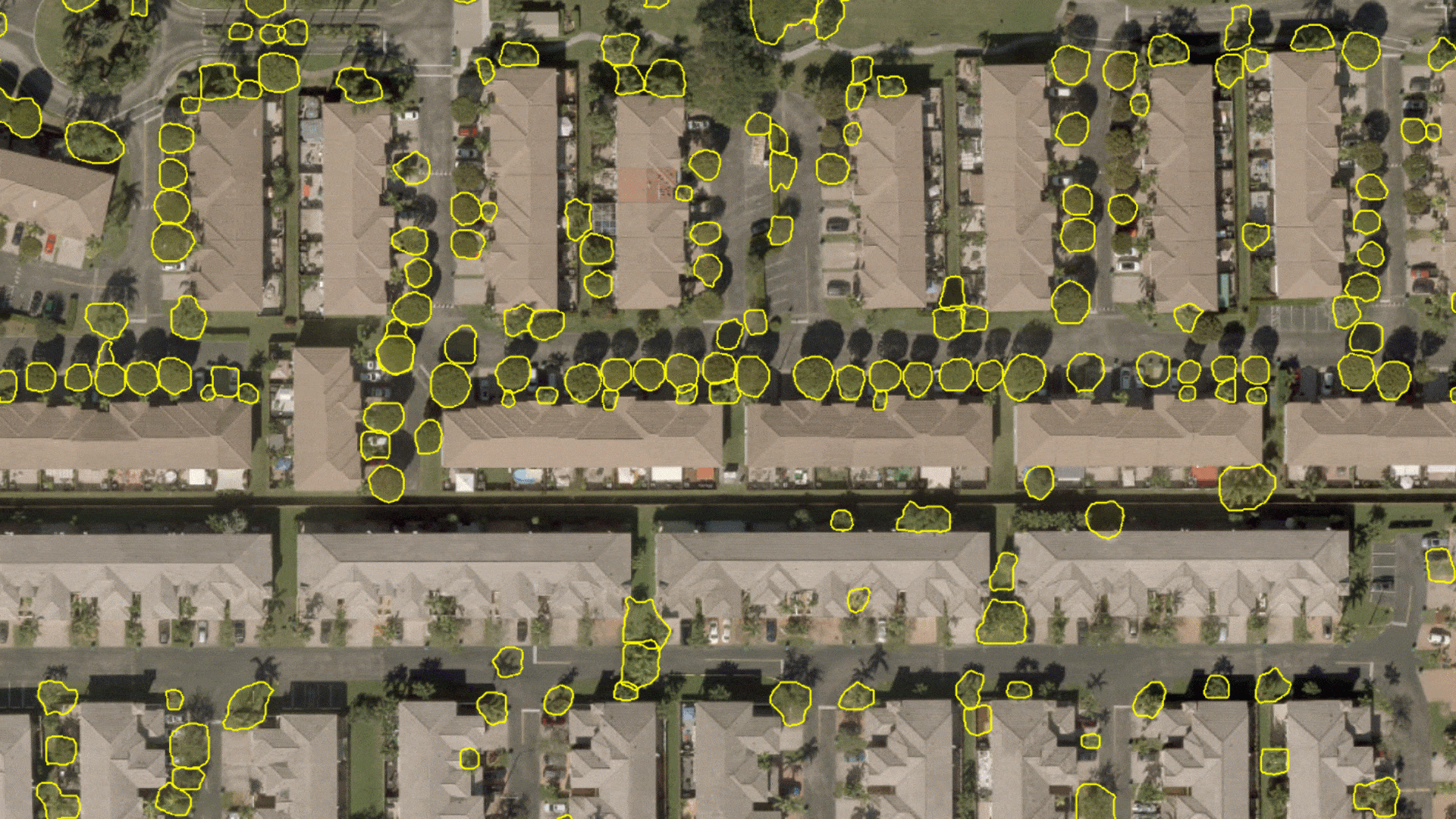
Oil Spill Detection (SAR)
Oil spills have a significant impact on the marine environment, economy, and ecosystems by releasing toxic chemicals that can persist in the ocean for years. While some spills are accidental, deliberate dumping by cargo ships also contributes to the problem. Detecting and removing oil from the ocean surface is challenging, but routine monitoring can help prevent illegal dumping and support cleanup efforts. This deep learning model automates the detection of potential oil spills using Sentinel-1 SAR data, aiding in the efficient identification and monitoring of oil spills.
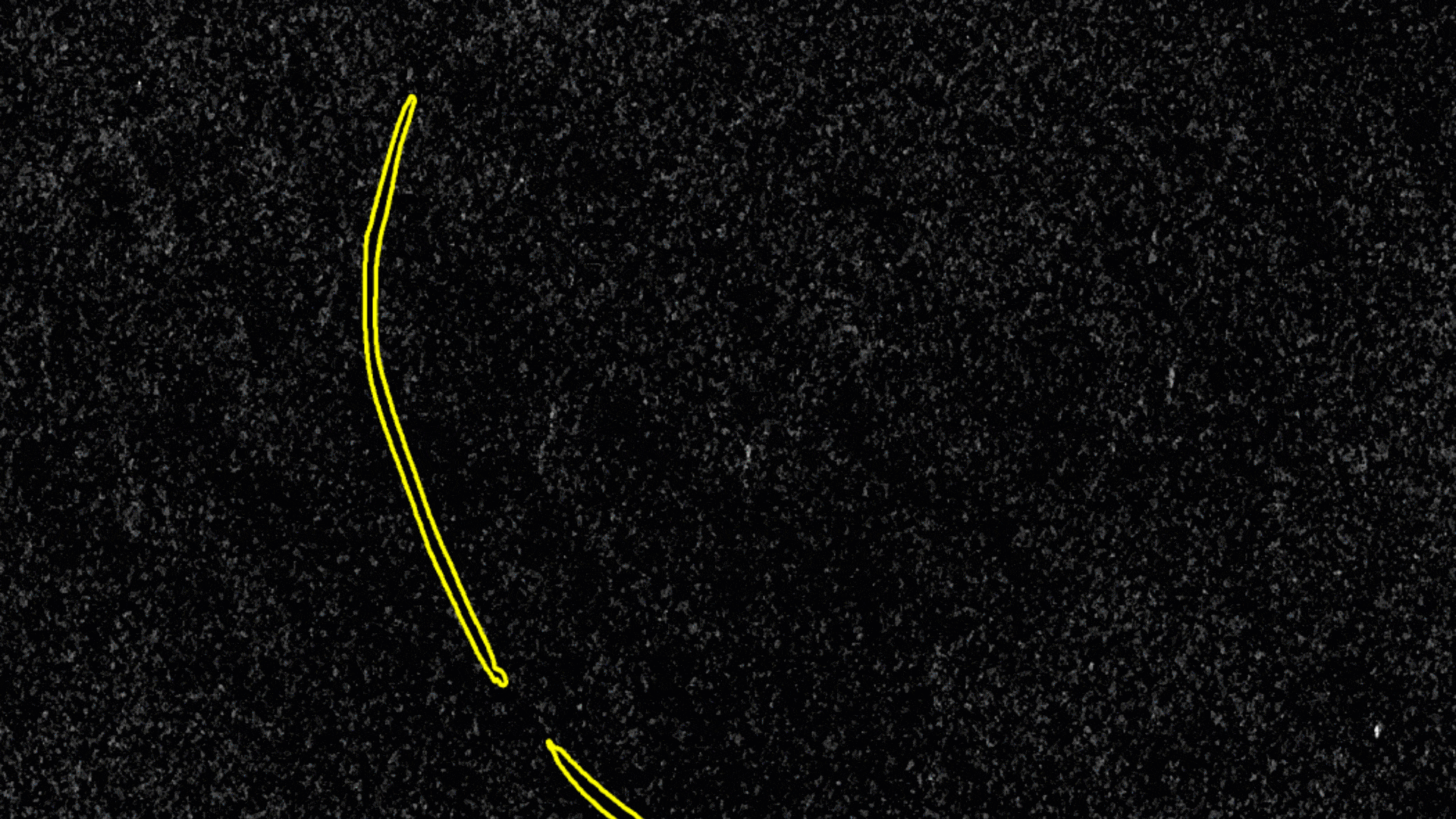
Oil Tank Detection
Oil tank detection holds immense significance in the oil and gas industry, helping monitor and manage critical infrastructure and enabling proactive maintenance, leak prevention, and emergency response. The ability to automatically detect and locate oil tanks enhances operational efficiency, safety, and environmental stewardship while also reducing the risk of accidents and ensuring regulatory compliance. This deep learning model enables accurate identification of oil storage tanks from high-resolution satellite imagery.
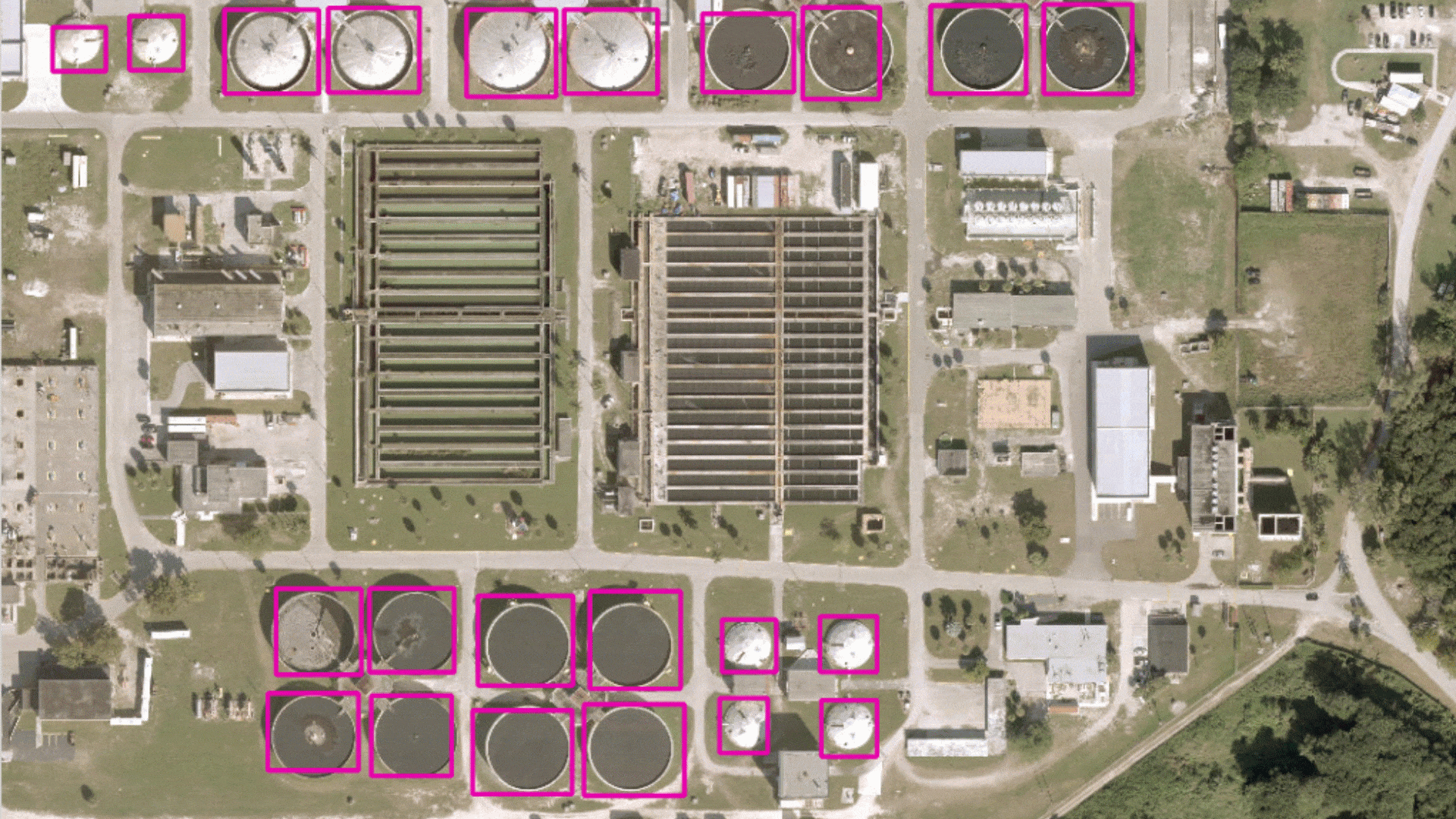
Utilities
In the utilities sector, AI plays a crucial role in optimizing operations and enhancing reliability. AI contributes to a more resilient and efficient utility infrastructure. Here are 3 models that we have added to support the utilities sector:
Cooling Tower Detection – USA
Cooling towers are commonly used in industrial and commercial settings to release the heat absorbed by circulating water in a building’s HVAC system or industrial machinery. Detecting cooling towers in aerial imagery can be particularly useful for city planners to understand the heat dissipation infrastructure, monitor land use patterns, and optimize zoning regulations. Moreover, identifying cooling towers aids environmentalists in assessing the impact of industrial activities on local ecosystems, air quality, and overall sustainability.
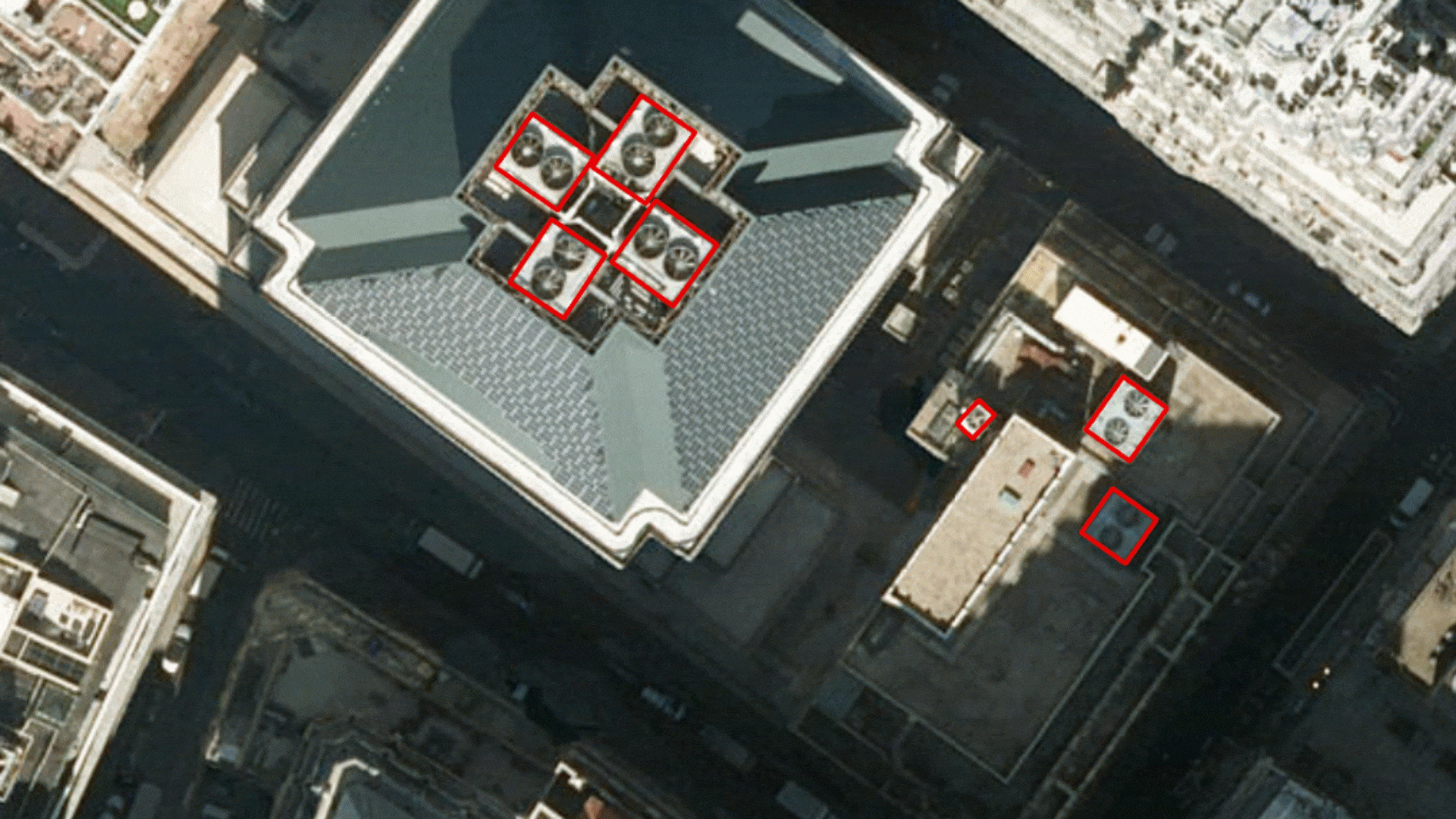
Pylon Detection – USA
Pylons are essential structures in the utility industry and play a crucial role in supporting power lines. Identification of pylons enhances infrastructure management and safety, ensuring reliable electricity transmission and minimizing the risk of potential hazards. Use this deep learning model to automate the task of detecting pylons in high-resolution satellite or aerial imagery.
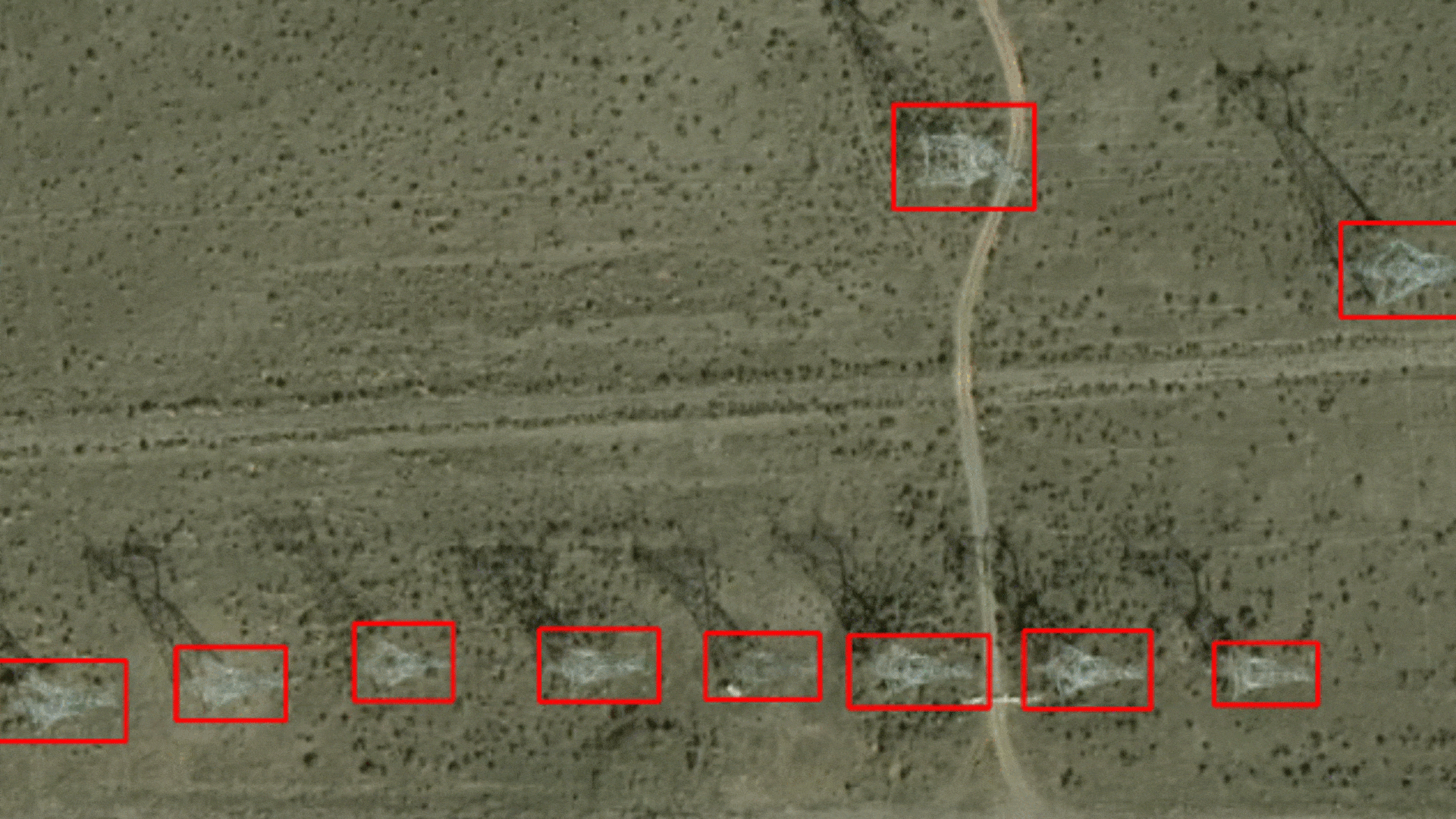
Transmission H-Structure Detection
Automating the detection of H-frame structures and their components can greatly assist in the maintenance and repair prioritization of transmission infrastructure. This model can automate the tedious task of identifying H-frame structures and their various parts, enabling more efficient and accurate analysis. This automation helps monitor damage to the transmission infrastructure, prevents energy loss, and aids in providing an uninterrupted power supply.
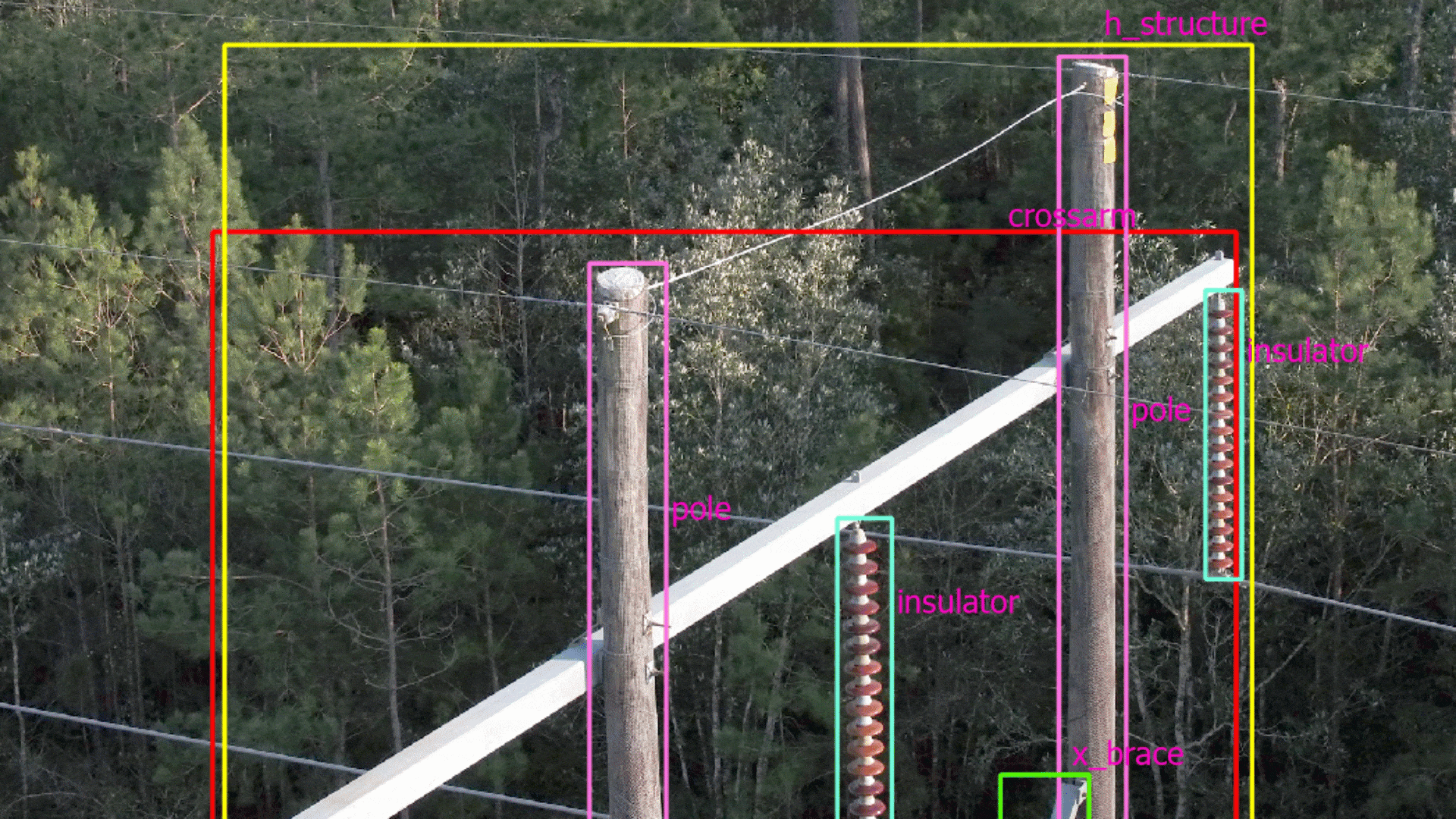
Insulator Defect Detection
Electric transmission towers employ insulators to prevent current leakage to the ground, but high-voltage electricity can damage them. This model automates the identification of insulator defects from high-resolution, oriented imagery, aiding in prioritizing maintenance.
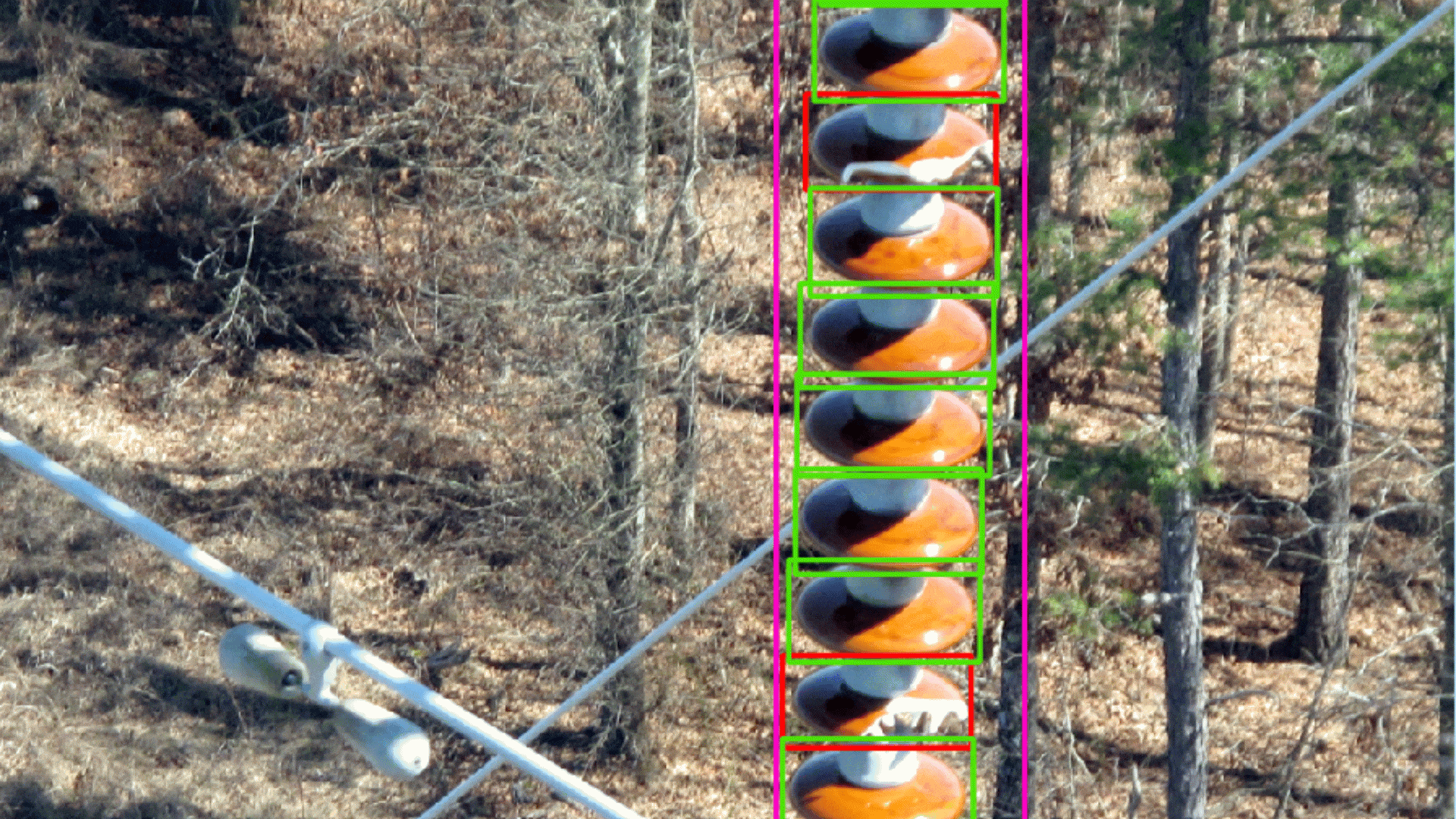
Insurance
In the insurance sector, AI plays a crucial role in estimating damage and verifying claims, contributing to a more reliable system. Here is a model that we have added to support the insurance sector:
Ship Detection (RGB)
Ship detection plays an important role in defense and security, port management, environmental monitoring, insurance and risk assessment, and maritime search and rescue operations. The Ship Detection (RGB) pretrained model detects and localizes ships in high-resolution optical satellite images. It can handle both dense and sparse ship patterns. The detected polygons align with the ships’ rotation angles to provide more accurate and precise information about the ship’s orientation and enhance the spatial representation of ships. To view model results, see this story.
This model goes beyond just the insurance sector and is relevant to transportation, defense and intelligence (SOM workflows), and public safety.
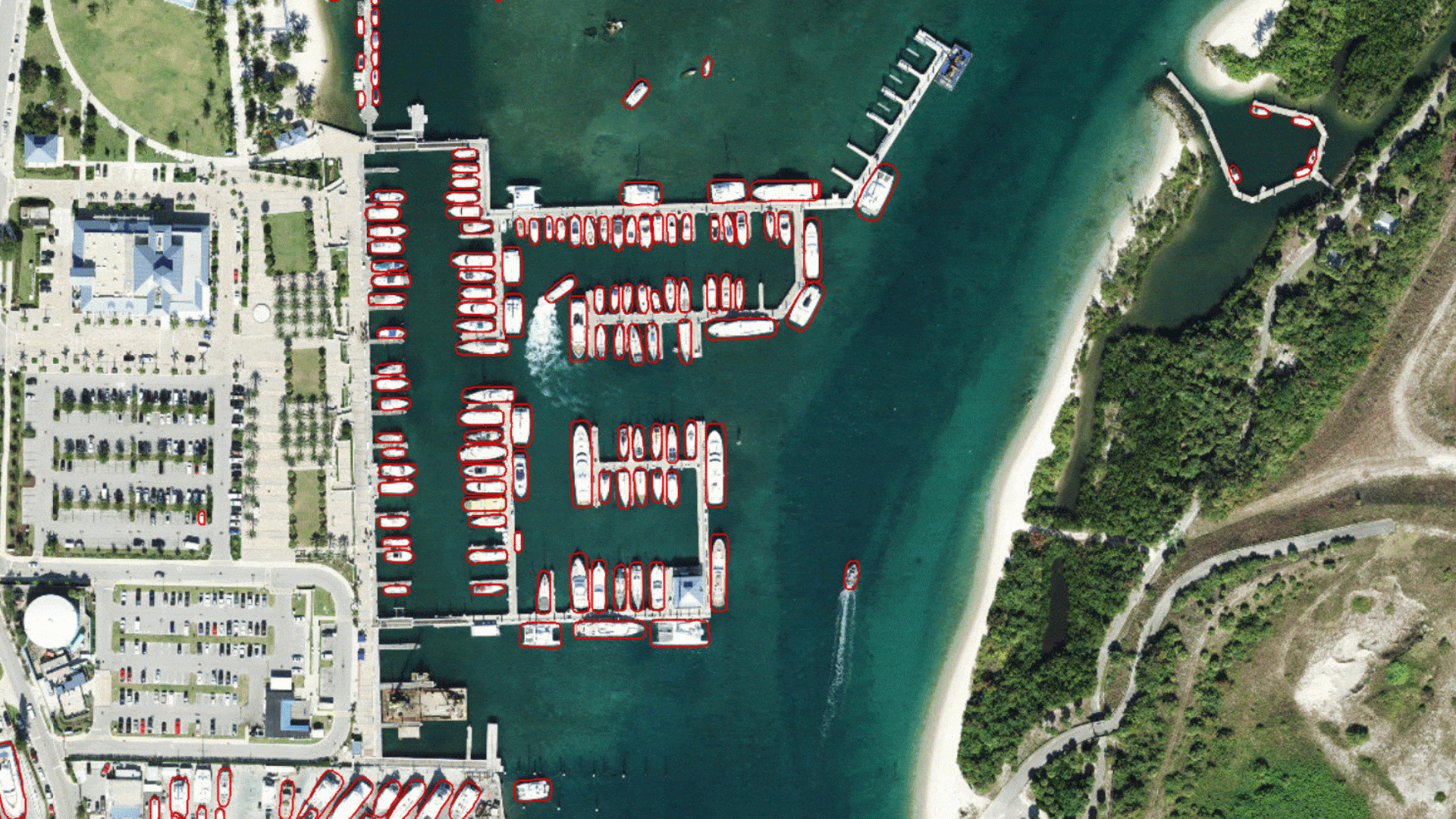
State and Local Government
Pool Segmentation
Tax assessors at local government agencies often rely on expensive and infrequent surveys, leading to assessment inaccuracies. Finding pools that are not on the assessment roll is valuable to assessors and ultimately results in additional revenue for the community. This AI model helps automate the task of finding not only where pools are but also calculate their area. It also assists swimming pool maintenance companies in their marketing efforts and supports public health and mosquito control agencies in mitigating vector-borne diseases.
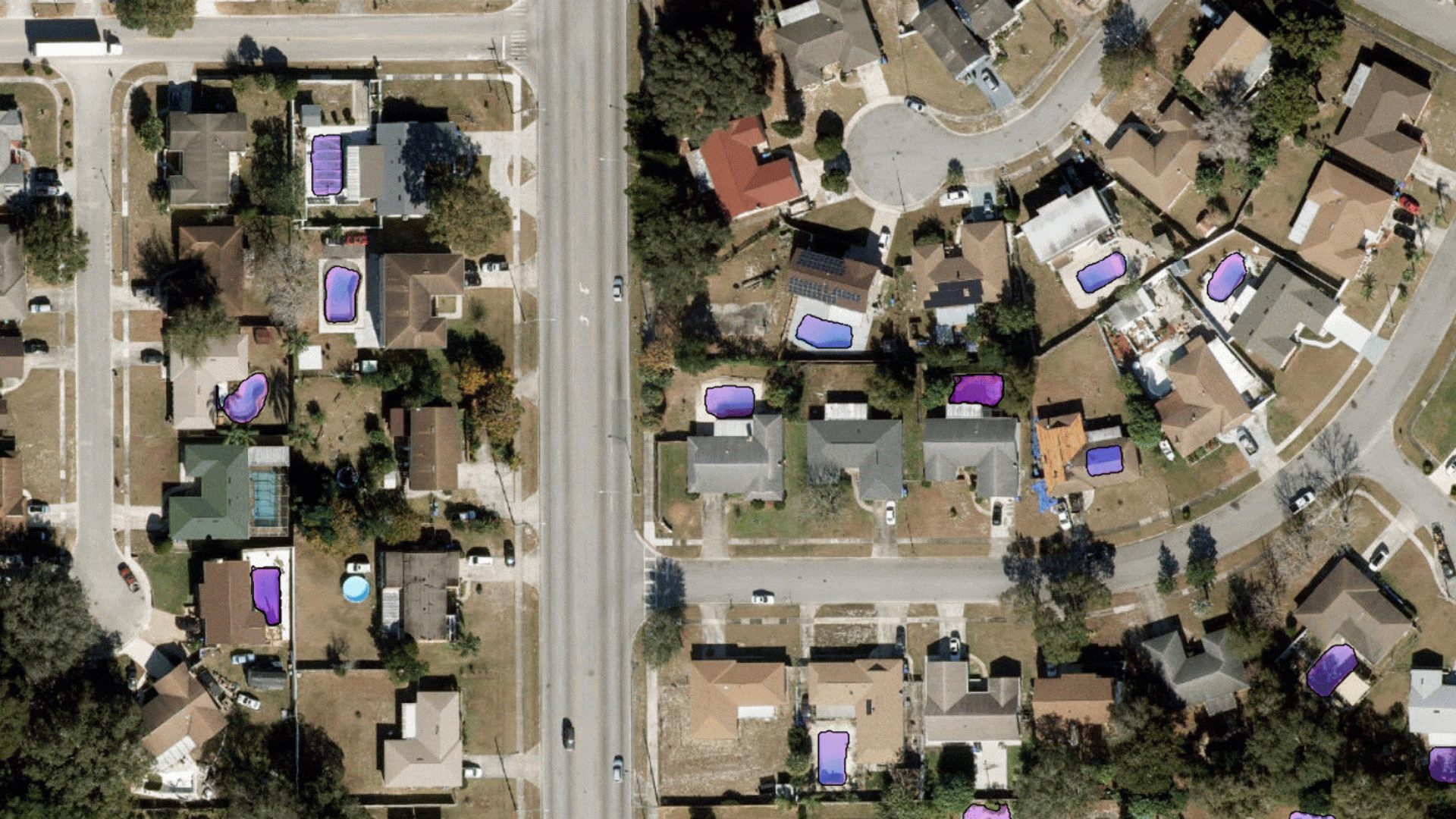
Optical Character Recognition
Text is prevalent in the natural scenes around us in the form of road signs, billboards, house numbers, and place names. Extracting this text can provide additional context and details about the places the text describes and the information it conveys. This model can be applied to various tasks, such as automatically detecting and reading text from billboards and signboards, identifying house numbers in street-level imagery, digitizing scanned maps, and so on, and converting images containing text to actionable data.
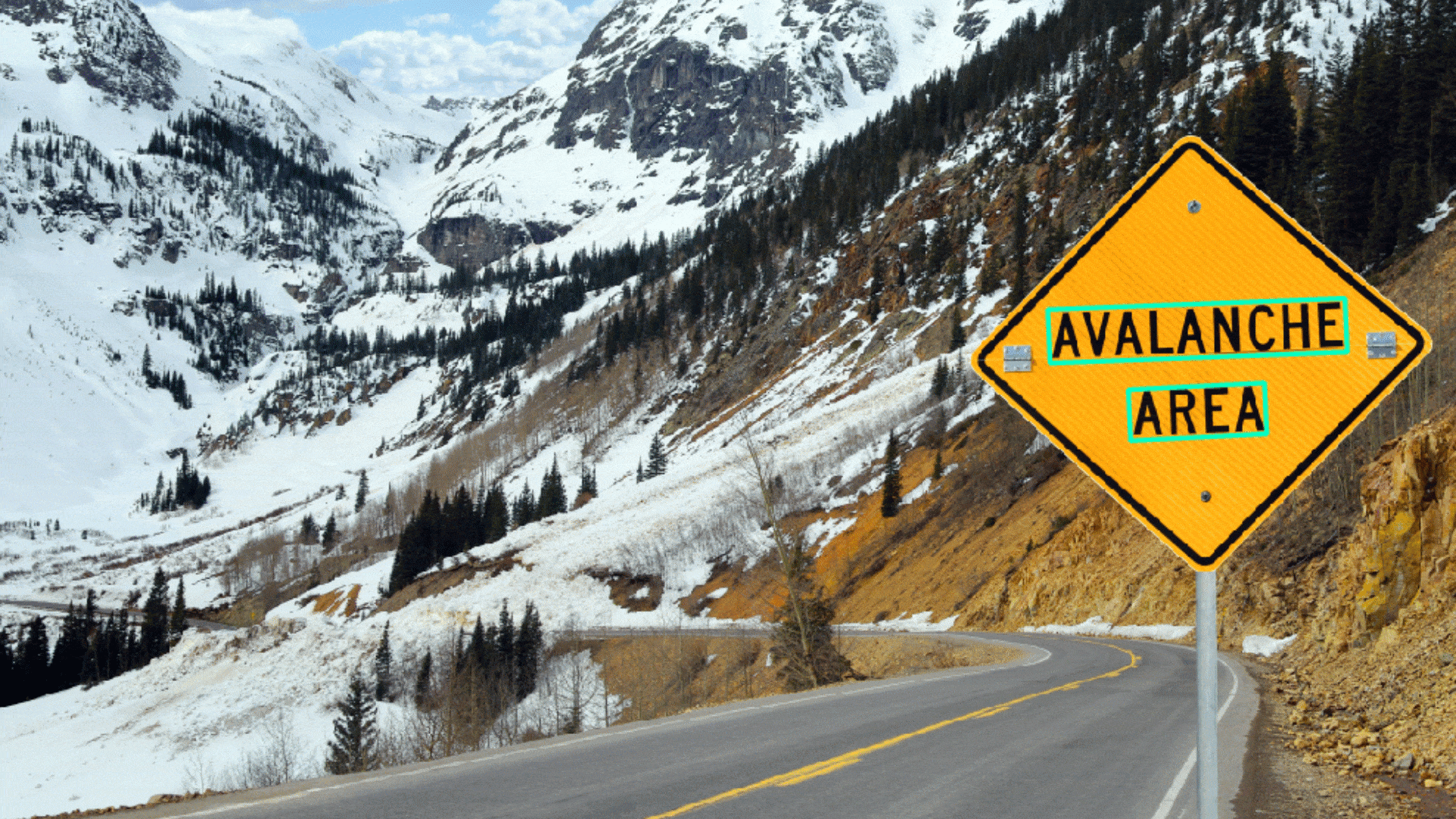
General Purpose Models
Common Object Detection
The model can be used in ArcGIS Survey123 to detect common objects in photos that are captured with the Survey123 field app. The detected objects can be used to extract attributes, annotate objects, or redact them from photos collected during surveys. The use of AI models in smart assistants augments field data collection workflows, transforming the mobile device camera into a tool that can recognize objects relevant to the workflow at hand. It can also make data collection more efficient and less error-prone.
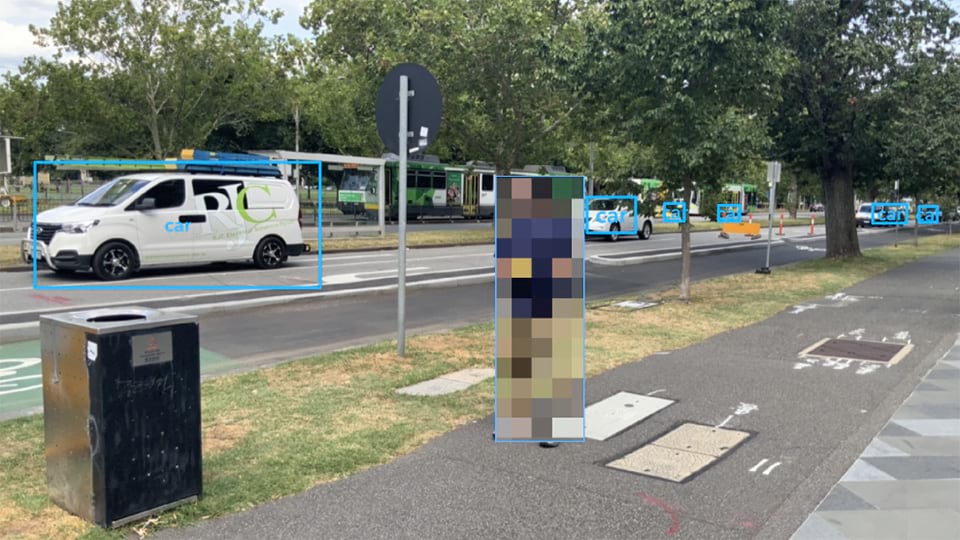
Segment Anything Model (SAM)
If any of the above models still don’t meet your needs and you wish to extract other kinds of features from imagery, we’ve got you covered! Meta’s Segment Anything Model (SAM) is a foundational computer vision model that can extract features of all kinds in imagery, generalizing across domains without additional training. We have packaged it as a Deep Learning Package (DLPK), allowing you to use it seamlessly with ArcGIS and apply it to overhead and oriented imagery. See some of the results of this model in this story.
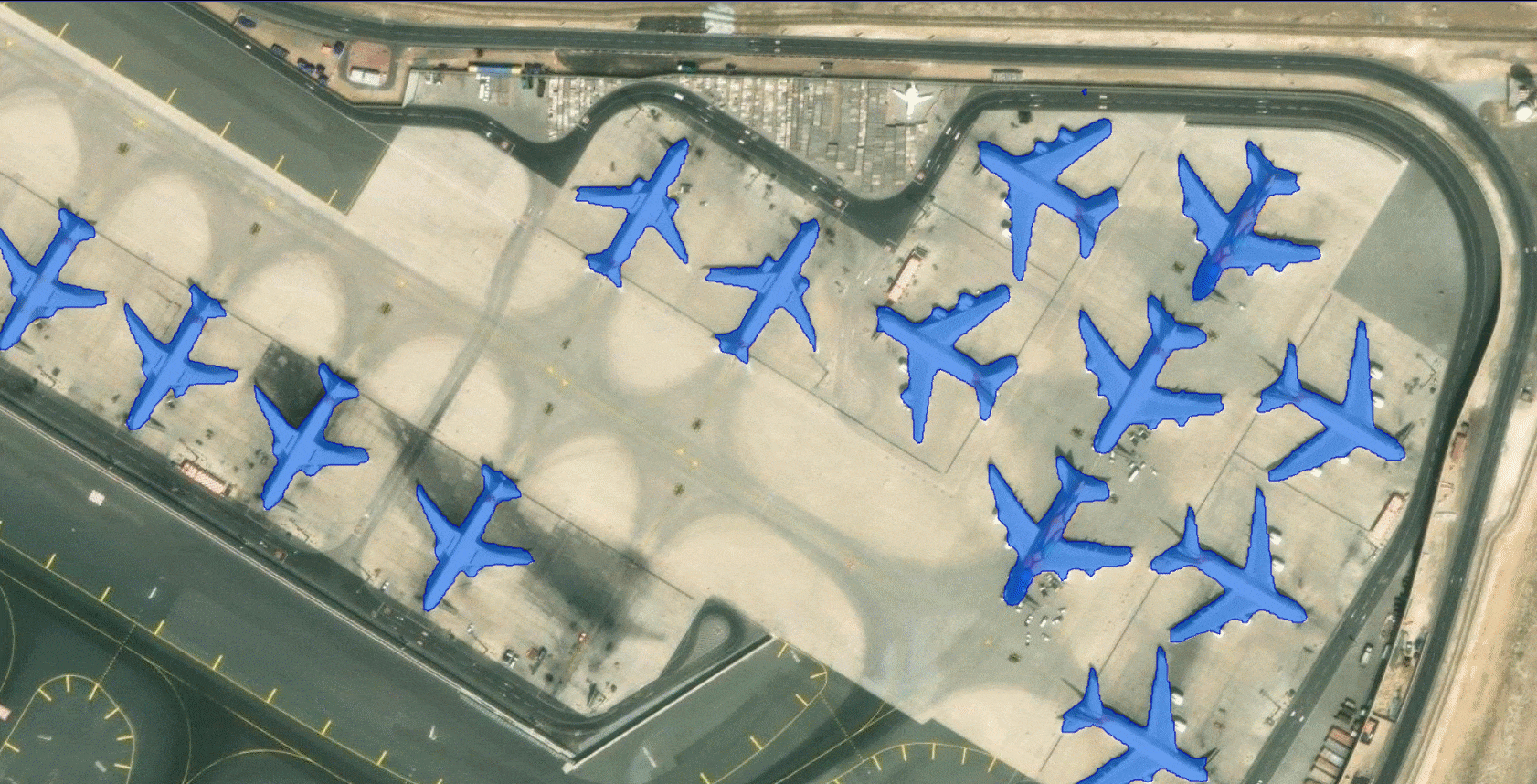
Access the Pretrained Deep Learning Models
The ArcGIS Living Atlas of the World is a resource for exploring pretrained deep learning models and other items. To navigate to the Living Atlas, you can follow these steps:
- Open your web browser and go to the ArcGIS Living Atlas of the World
- Once you’re on the website, you can use the search bar at the top of the page to search for “dlpk packages”.
- Hit enter or click on the search icon to initiate the search.
- The search results will display a list of available dlpk packages related to your search query.
- Click on the package that interests you to view more details and access the comprehensive instructions for getting started with the model.
You can also browse through the models’ pages and their guides here.
If you have any questions or need further assistance, feel free to reach out to the Imagery & Remote Sensing community and we can assist you with your inquiries.
Enjoy exploring these pretrained AI models, and let us know which other models you would like to see!
Article Discussion: