Afia Boney, a first-year master’s student at the Okayama University Graduate School of Environmental and Life Science, won the Best Story Map category of the Your Caribbean Story Student Competition for her research on “Prediction of Rainfall in Trinidad and Tobago using Machine Learning”. As a continuation of her project using machine learning for rainfall prediction, she explored the ArcGIS Learn Time Series model. In this blog, she has shared her story, inspiration, and the results of her study. Handing it over to Afia!
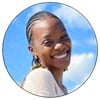
-By Afia Boney
Trinidad and Tobago is a twin-island nation located in the Southern Caribbean. Its location and geology make it susceptible to natural hazards. One of these hazards is flooding. With its proximity to the equator, the islands experience two opposing seasons: dry (January-May) and wet (June-December). During the wet season, the hurricane season concurrently adds to potential flooding hazards. As a resident of La Horquetta, a suburban town in the east of Trinidad, I have witnessed several floods over the years.
La Horquetta
The Trinidad and Tobago Housing Development Corporation (HDC), formerly known as National Housing Authority (NHA), developed one of the biggest residential projects called La Horquetta Housing Development which is situated in the Tunapuna-Piarco region. Nestled south of the Borough of Arima, it is bordered to the north by the Churchill-Roosevelt Highway, to the west by the Arima River, to the south by the Caroni River, and to the east by the Aripo Savannas. The housing development commenced in 1976 and was completed in the 1980s. The recent expansion of Greenvale La Horquetta was the final phase of the housing development, completed in 2014.
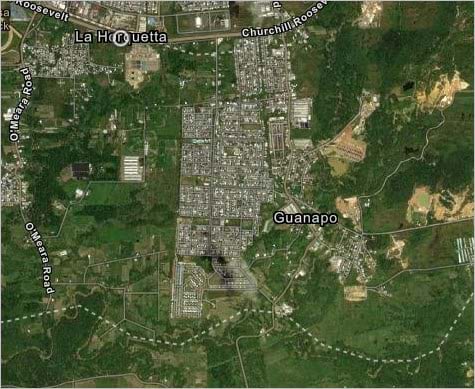
The community prides itself on having its own police station, health center, post office, schools, churches, community center, swimming pool, village plaza, farmers’ market, basketball and netball court, and football fields. Having such amenities has allowed the community to host several activities for the young and the elderly.
Unfortunately, despite all the life the community has, behind all the infrastructure, the community is continually plagued with flooding. From as early as I can remember, the community has been susceptible to the primary and secondary effects of flash flooding. However, it is not localized to only La Horquetta. Over recent years, Trinidad and Tobago has been experiencing major flood hazards, and this is comparable to global patterns.
During my tenure in form 6 (high school), my internal assessment for my final secondary-level geography examinations was based on the flooding hazard in St. Ann’s, Trinidad. As a resident of not only La Horquetta but Trinidad and Tobago, I have witnessed the impact of natural hazards on the national landscape. This has heavily influenced my interest in the field. I believe the governing bodies and society could benefit from predicting future rainfall patterns using historic precipitation data. Machine learning techniques are increasingly popular as an approach to rainfall prediction as opposed to traditional numerical models. In this blog, I have discussed the results acquired using the ArcGIS Learn Time Series model and past historic data.
Rainfall prediction and machine learning
I have used Neural Prophet in the past, which uses a neural network built on PyTorch (an open-source machine learning framework that is based on the Torch library) for modeling time-series data. To know more about the results, you can refer to the Living in the Fear of Flood story.
As a continuation of my research to forecast rainfall, I tried the ArcGIS learn time series model that uses convolutional neural networks curated for time series datasets. These include InceptionTime, ResCNN, Resnet, and FCN.
Machine learning workflow:
For a more detailed view of the workflow, sign in with your Learn ArcGIS account to access this ArcGIS Notebooks notebook.
Results
The following sections detail the forecast results.
2010-2016 data to forecast 2017-2021
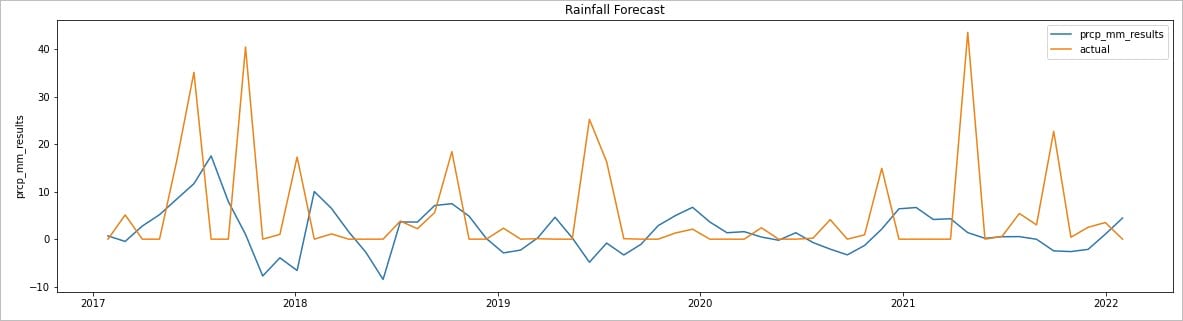
The actual compared to the predicted in the graph overlap at a certain period. But this is not highly accurate. Next, I increased the sample data and reduced the prediction time.
2010-2020 data to forecast 2021
The prediction for 2021 using 2010–2020 overlaps at certain periods but is not highly accurate. Next, I used sample data from 1980–2016 to predict 2021.
1980-2020 data to forecast 2021
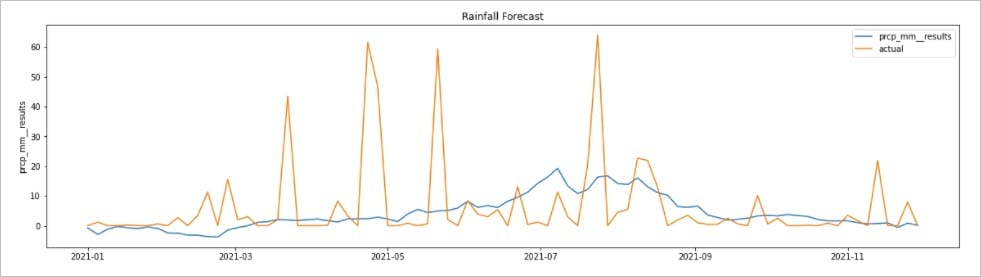
The graph shows a similar trend at the beginning of the year; however, as we move toward the later months, the predicted trend is different from the actual trend. Outliers are not predicted accurately to the actual rainfall.
Future Work
I would like to include precipitation values before 2010 and see if the time series model can predict rainfall with better accuracy. I have worked with univariant time series analysis—that is, my observations have only been dependent on precipitation data. In the future, I would like to explore multivariant time series analysis wherein I can incorporate other variables air temperatures and wind speed along with precipitation.
Why this study matters
I have been speaking about flooding and rainfall prediction. But why does all this matter? As climate change mechanisms morph into more complex phenomena, paleoclimatic records are being discovered and used to provide information for scientists and civilians alike. It is from this knowledge that we can foster a better understanding of the disasters that accompany the changes in the climate system and, in so doing, be prepared to attenuate the risks that they may generate. Climate change is intensifying the risk of heavy rainfall worldwide due to the warmer atmosphere. Areas that are most vulnerable need to be protected at all costs.
Data Sources
- Precipitation data was acquired from Trinidad and Tobago Meteorological Service.
- Forecasting monthly rainfall in California using Deep Learning Time Series techniques
- Forecasting Air Temperature in California using ResCNN model
ABOUT THE AUTHOR
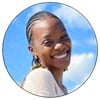
Afia Boney
I am an international student at Okayama University from Trinidad and Tobago. After graduating from the University of the West Indies, Mona Campus, Jamaica, with a Bachelor of Science in Geology and a minor in Chemistry, I returned to my homeland and worked in records and inventory management, and then as a Land Assistant Officer at the Ministry of Agriculture, Land, and Fisheries. In 2020, I was awarded the Monbukagakusho scholarship from the Government of Japan, to pursue my master’s degree. My research is concentrated on photogrammetry and object detection. However, I love Geography and mingling with GIS software. Aside from my research interests, I enjoy trying new recipes, dancing, and hiking. One of my bucket list items is to go skiing even though I’m not too fond of the cold weather.
Article Discussion: