Today was a fun and exciting day at the Esri Federal GIS Conference 2021 highlighted by great user presentations, inspiring talks, and a powerful technology showcase. The plenary session showcased technology ranging from augmented reality to 3D to IoT, and of course, deep learning. The imagery and remote sensing demonstration showed how AI was effectively put to use in a SAAS environment. Driving the AI was a pretrained model that is downloadable for all users from ArcGIS Living Atlas. This is just one of the many models that have been released on ArcGIS Living Atlas of the World.
Ever since the pretrained deep learning models were released on ArcGIS Living Atlas, they have been well received. These models are pretrained by Esri on large volumes of data and can be readily used (no training required) to automate the task of digitizing and extracting geographical features from satellite imagery and point cloud datasets. These models are available to anyone with an ArcGIS Online subscription.
Eight new and updated models were released at the Federal GIS Conference plenary session today. With this release, you now have 12 pretrained deep learning models that you can use. Go to the geoprocessing tools in ArcGIS Pro, point the deep learning tools to the models and at the raw data, and the model will extract geographical features at the click of a button. See a quick video tutorial of how this works.
Building Footprint Extraction
The Building Footprint Extraction model is the most popular model so far. This deep learning model is used to extract building footprints from high-resolution (10–40 cm) imagery. Building footprint layers are useful in preparing base maps and analysis workflows for urban planning and development, insurance, taxation, change detection, infrastructure planning, and a variety of other applications.
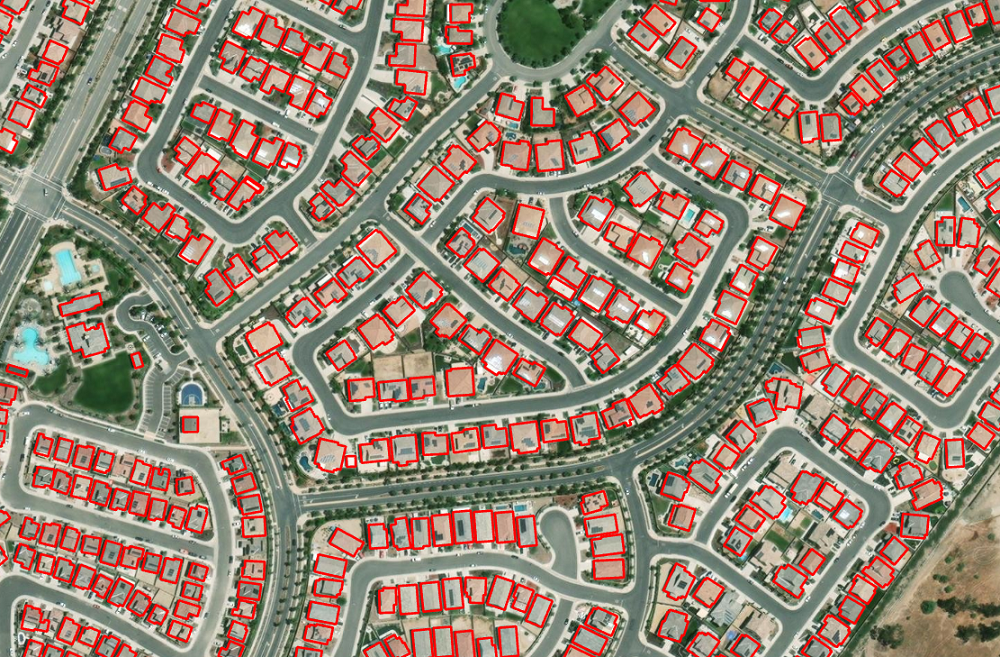
While its designed for the contiguous United States, it also performs well in other parts of the globe. Here’s a story map presenting some of the results. This model has been updated and trained on more data. This new model works well even when buildings are in close proximity–something that the original model did not. See the difference in the results in the following images:
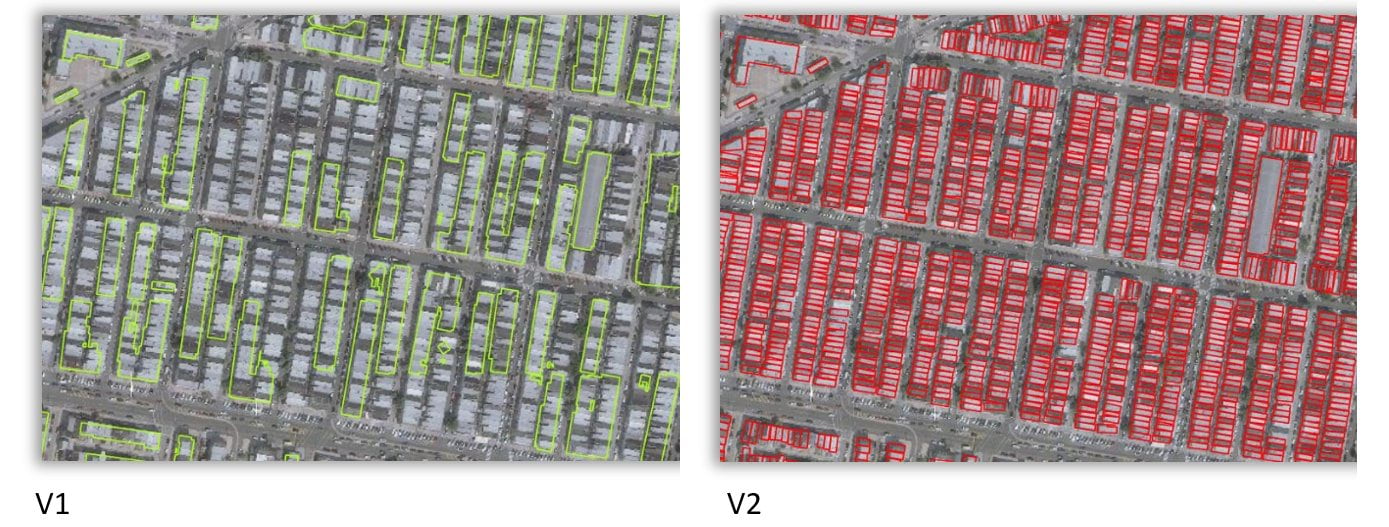
You’ll also notice a significant drop in false positives over water, dock yards, and places where buildings typically don’t exist.
Road Extraction
The new Road Extraction model is used to extract roads from satellite imagery. Roads are one of the primary GIS layers required by any county, city, state, or country governmental agency for infrastructure planning, urban planning, and developing an effective, efficient information model. Digitizing and updating roads can be time consuming. This model automates most of the digitizing process. It is based on the MultiTaskRoadExtractor in arcgis.learn model, which is a state-of-the-art model that provides connected road segments such as those shown in the following image:
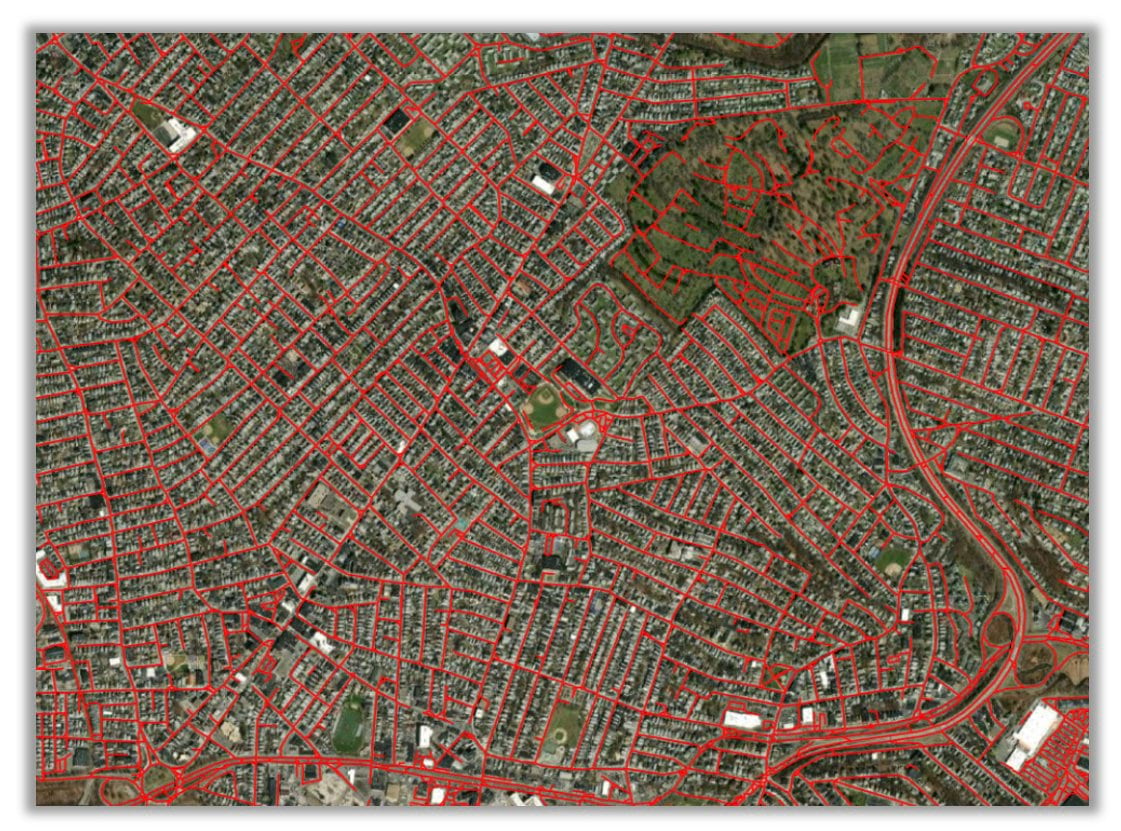
Extracting road networks from satellite images often produces fragmented road segments when a semantic segmentation model such as U-Net is used. This is because satellite images pose difficulties in road extraction due to occlusion caused by trees, buildings (in off-nadir imagery), and shadows. This model uses multitask learning, which is inspired by how humans annotate roads by tracing them at specific orientations.
This model also works on dirt roads and well pad access roads such those shown in the following image:
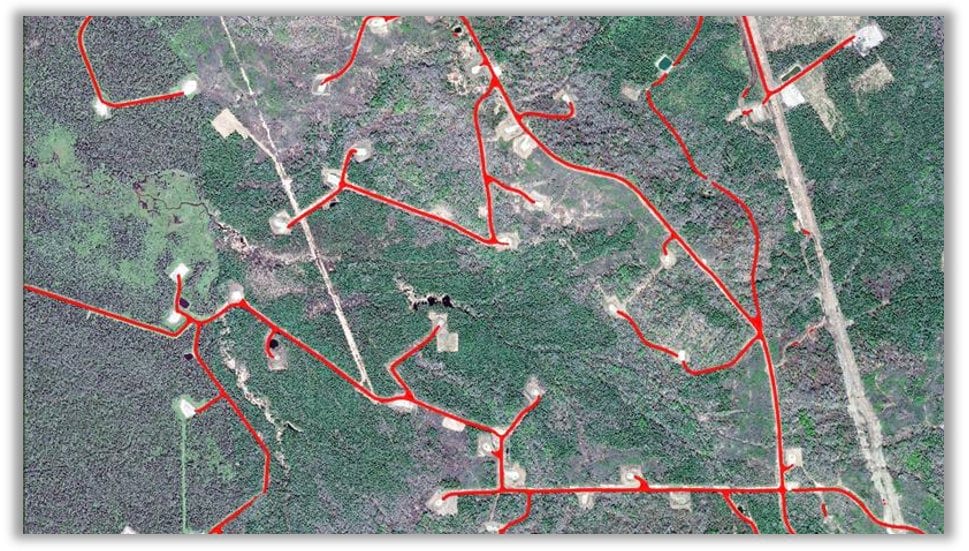
Land Cover Classification
In October 2020, Esri released its first land cover model that was trained on the National Land Cover Database (NLCD) dataset in the United States and works on Landsat-8 scenes. The resultant land cover maps can be used for understanding urban planning, resource management, change detection, agriculture, and a variety of other applications in which information related to the earth surface is required.
Today, a new Land Cover Classification model is being released that has higher resolution, as it works with Sentinel-2 imagery. This model works across Europe. It is trained on CORINE Land Cover (CLC) 2018 with the same Sentinel-2 scenes that were used to produce the database. Land cover classification is a complex exercise and is difficult to capture using traditional methods. Deep learning models have a high capacity to learn these complex semantics and provide superior results, as shown below.
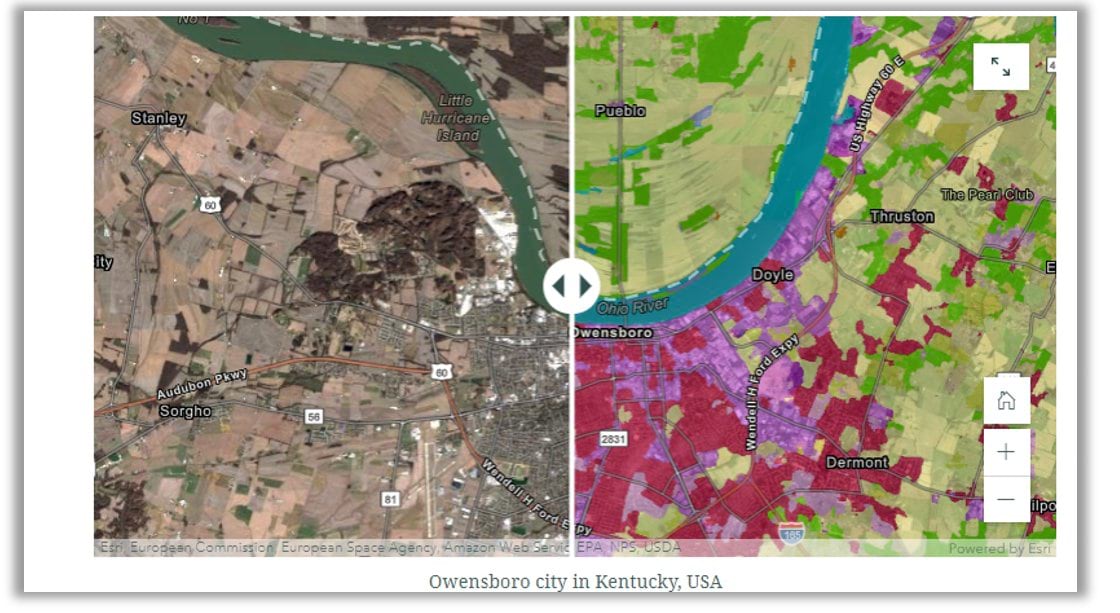
This story map shows the results of this model across several geographies.
This model can also be used for change detection, as you can run it on imagery from two different times and see the change in land cover, such as that caused by a wildfire. In the image below, you can see the growth of urbanization. New residential areas are shaded in red.
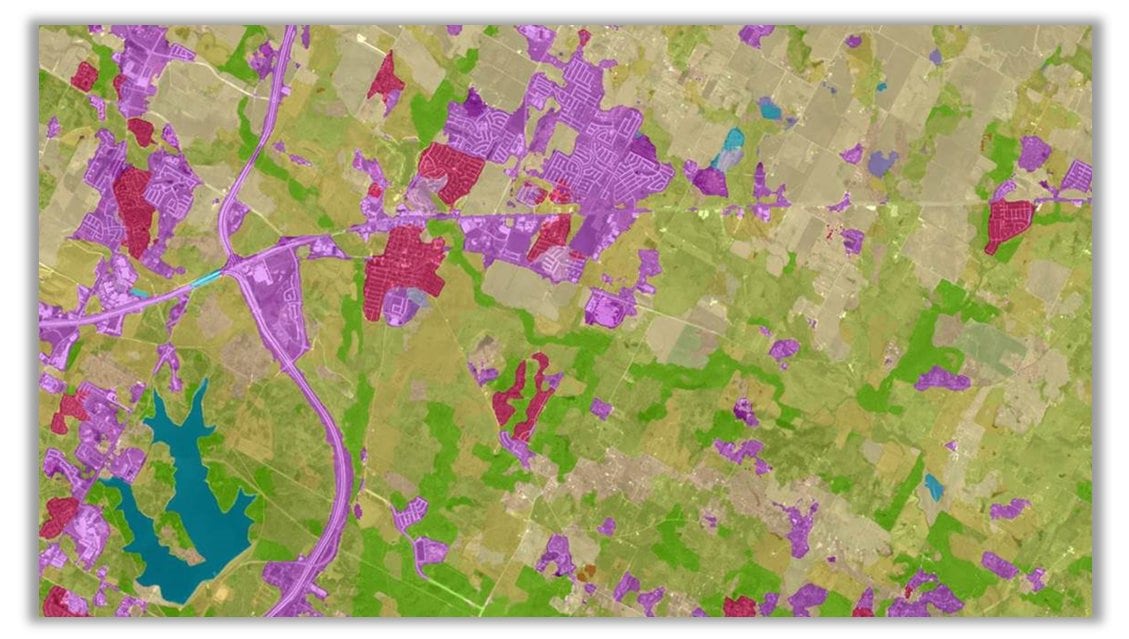
Human Settlements
While high resolution maps provide value in understanding human settlement patterns, creating small scale maps derived from relatively lower resolution satellite imagery brings its own value to understanding regional or global growth patterns, population distribution, resource management, change detection, and a variety of other statistics. One example is vaccination planning; only by finding all the unmapped villages can you be sure that your vaccine is reaching all the people that need it.
The following image shows the results from the new Human Settlements model that works on Landsat 8 imagery and extracts such settlements:
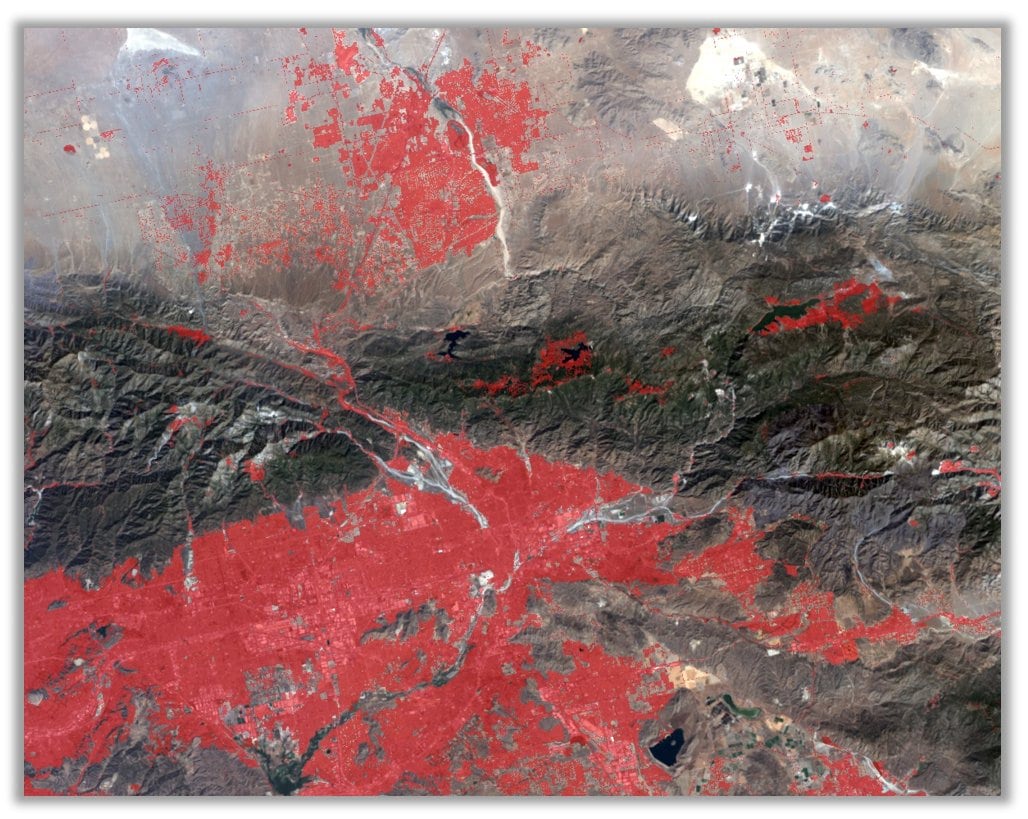
You can see how urbanization is affecting areas across the globe with this model. For example, you can see how the human footprint has increased around Sharjah in the UAE from 2015 to 2021 in the following images:
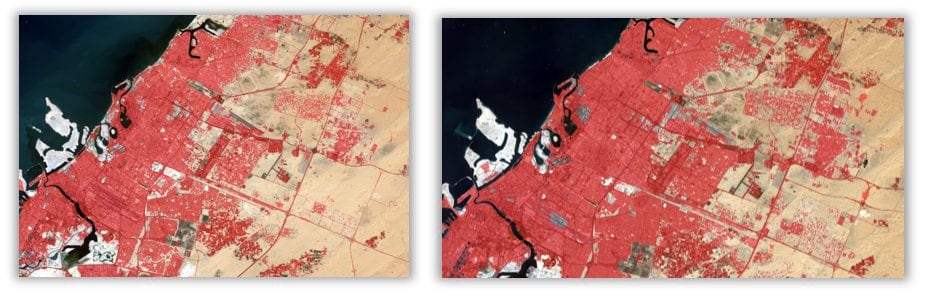
We have also released a Human Settlements Extraction model that works on Sentinel imagery.
Shipwreck Detection
In addition to aerial imagery, these new models include one that detects shipwrecks using bathymetric data. Albeit a niche industry, it is a critical requirement to keep S57 nautical charts up to date. Unmarked shipwrecks can lead to disasters damaging vessels or ports resulting in loss of life and property.
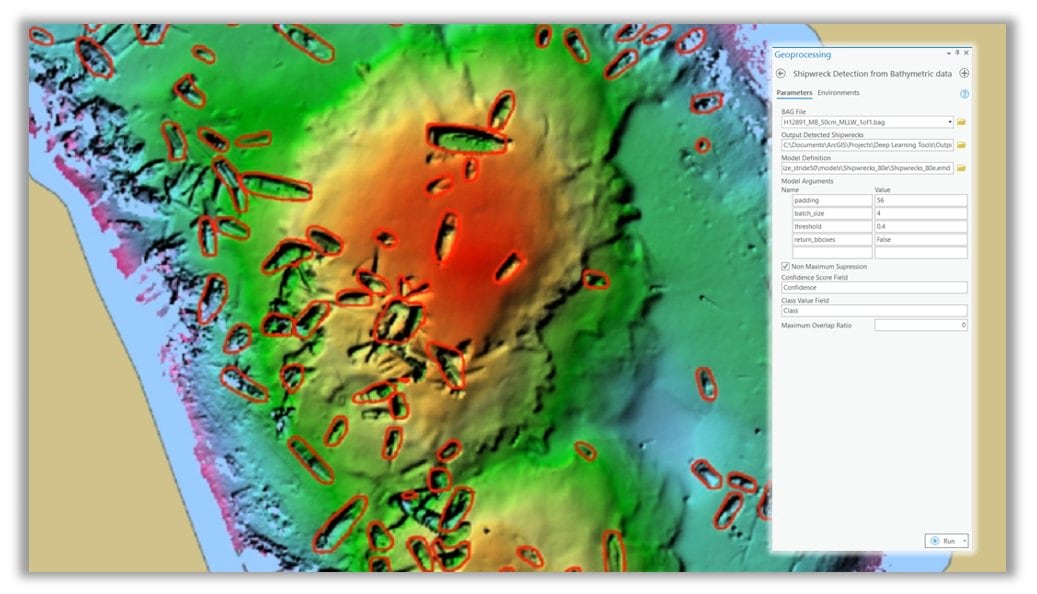
This model includes a geoprocessing tool that provides the necessary preprocessing steps and simplifies the process.
License Plate Blurring and Face Blurring
With the increasing number of sensors, influx of data, and democratization of a lot of that data, issues such as privacy are of concern. We have released two models to address this need. These models are used to anonymize or redact faces and car license plates from street-view imagery. You can use these model with the Classify Pixels Using Deep Learning tool in ArcGIS Pro.
Sample results from the model are shown in the following image:
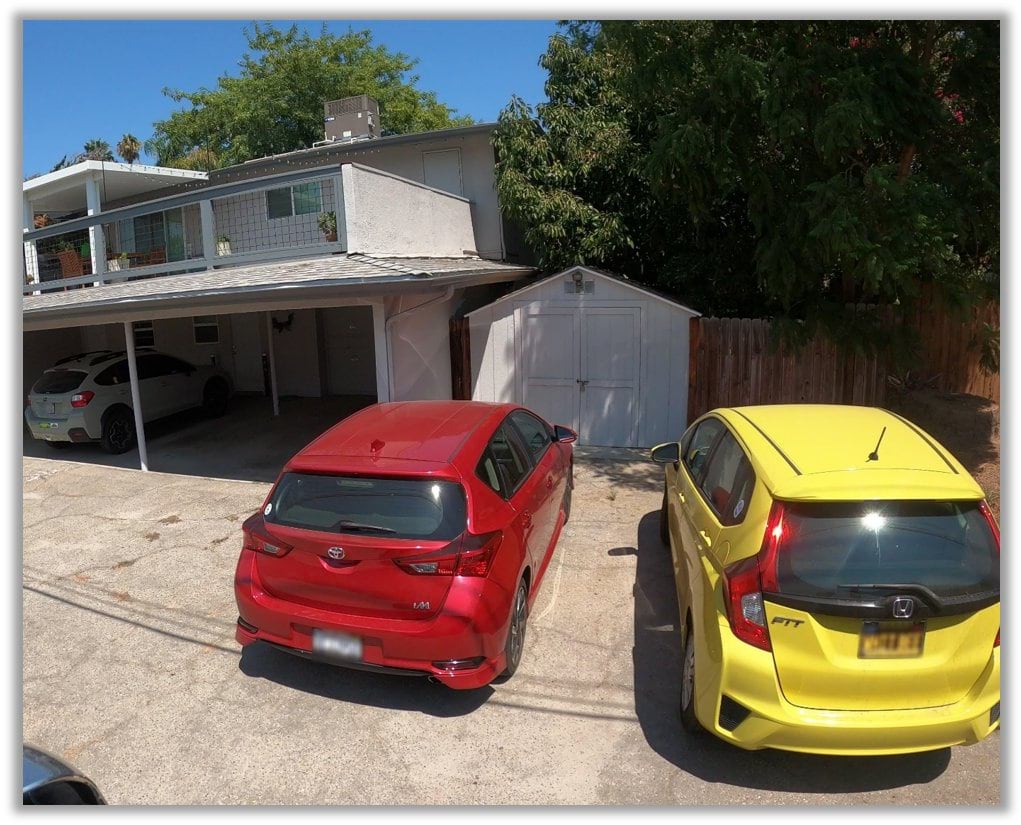
These are just a few of the models that have been developed over the past few months to automate and simplify your workflows. Try them out. You can use ArcGIS Pro, ArcGIS Enterprise, or ArcGIS Online. Each model includes helpful documentation to get you started.
Resources to get you started
Introducing pretrained deep learning models
Deep Learning with ArcGIS Pro Tips and Tricks: Part 1
Deep learning models in arcgis.learn
Article Discussion: