Esri provides access to a curated library of more than 75 pretrained geospatial artificial intelligence (GeoAI) models that are accessible from ArcGIS Living Atlas of the World. These models are suited to perform a variety of AI tasks that address different business needs, such as extracting building footprints for urban planning, classifying structures for insurance purposes, or generating change maps from time series data.
In the wake of disasters like floods, fires, and earthquakes, the need for models that can detect or classify damaged infrastructure, as well as identify threats such as wildfires and floods, has become increasingly critical. Therefore, Esri has extended its library of models to address this urgent need, providing powerful tools for damage assessment and classification during disaster events. The following AI models are specifically designed to help first responders and analysts quickly identify areas at risk, assess the extent of damage, and manage emergency operations to streamline and enhance disaster response efforts.
This article provides an overview of each of these models as well as instructions on trying them out with your own imagery.
1. Damage Assessment (Drone Imagery)
This model addresses the need for rapid damage assessment in disaster-stricken areas, helping emergency response teams analyze the severity of damage from drone and aerial imagery. It is trained on the Low Altitude Disaster Imagery (LADI) v2 dataset, which includes over 10,000 aerial images labeled by Civil Air Patrol volunteers. The model automatically identifies key features such as roads, damaged infrastructure, and hazards like floods or debris. By accelerating the identification of critical areas, the model helps emergency managers prioritize response efforts. It works with high-resolution aerial imagery collected by drones or small planes.
2. Wildfire and Smoke Classification
With wildfire becoming increasingly frequent and destructive due to the changing climate, early detection and monitoring are crucial for minimizing damage. This model enables the automatic classification of wildfires and smoke, detecting both active fires and early signs of smoke that may indicate the start of a fire. It uses imagery from aerial drones and ground-based camera systems, functioning in a variety of settings and lighting conditions. This allows wildfire management teams to continuously monitor remote forested areas prone to wildfire, facilitating quicker interventions and more effective resource allocation.
3. Wildfire Delineation
Wildfire delineation is essential for accurately mapping the spread of active fires and assessing their impact. This model addresses the need for precise fire boundary detection by segmenting wildfire-affected areas using Sentinel-2 imagery. It helps emergency responders with risk assessment, evacuation planning, and resource allocation. In addition to delineating wildfires, the model can be applied to monitor volcanic lava flows, offering flexibility in managing different types of natural hazards. The model’s high temporal resolution enhances its utility in real-time disaster monitoring.
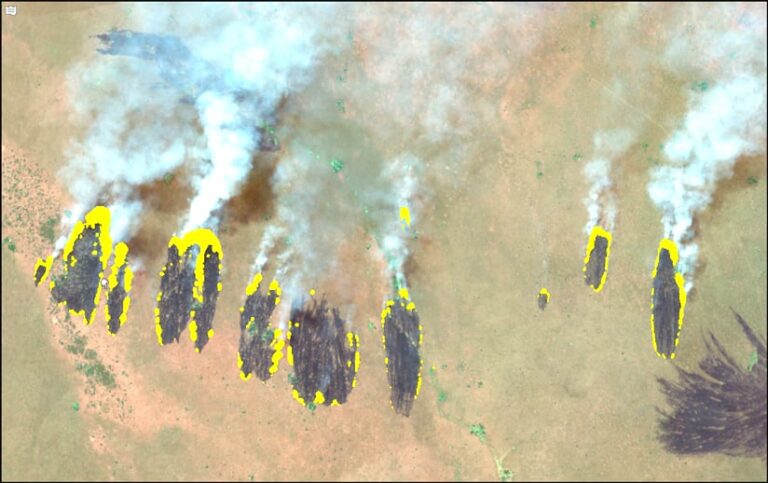
4. Prithvi—Burn Scars Segmentation
Burn scars present a challenge for postwildfire assessment, as distinguishing between burnt and nonburnt areas is critical for effective recovery and landscape management. The Prithvi model, developed by NASA and IBM, addresses this by automating the detection of burn scars from multispectral satellite imagery acquired by the Landsat or Sentinel-2 sensors. It is fine-tuned on the Harmonized Landsat (HLS) Burn Scar Scenes dataset, making it highly effective for identifying burn scars on a large scale. The model’s application of machine learning allows it to map the aftermath of wildfires, enabling emergency responders to assess damage and plan recovery efforts more efficiently.
5. Prithvi—Flood Segmentation
Flood monitoring and management are critical for mitigating the impact of floods, particularly in disaster-response efforts. This use of the Prithvi model automates the detection of flood-affected areas using high temporal resolution multispectral satellite imagery (Landsat and Sentinel-2). Fine-tuned with the Sen1Floods11 dataset, this model precisely identifies submerged regions, enabling responders and policymakers to assess flood extents more efficiently. By leveraging earth observation data and deep learning, Prithvi enhances the ability to monitor floods in real time, offering valuable support for evacuation planning, resource allocation, and postdisaster recovery efforts.
6. CLIP Zero-Shot Classifier
The CLIP Zero-Shot Classifier, a unique model in its class, offers a flexible solution for classifying images without the need for task-specific training. This model is powered by OpenAI’s Contrastive Language-Image Pretraining (CLIP) and allows users to define custom categories, making it ideal for disaster response, urban monitoring, and environmental analysis. This model works with both individual images and large image collections, performing well across different lighting conditions and locations. It not only assigns a class to each image but also provides a probability score, helping users prioritize results more effectively.
Next Steps
To explore Esri’s pretrained damage assessment and classification models, follow these steps to try them out with your own imagery in ArcGIS Pro, ArcGIS Enterprise, or ArcGIS Online:
- Fire up your web browser and navigate to ArcGIS Living Atlas of the World.
- At the top of the page, search for “dlpk”, short for deep learning packages.
- Browse the search results to find deep learning packages relevant to your workflows.
- Click any package to view more details, access comprehensive instructions, see model metrics, and explore guides to help you get started with the model.
- Explore the pages and guides (links.esri.com/pretrained-models) to learn how to integrate these models into your workflows.