The integration of cartography with AI—especially geospatial artificial intelligence (GeoAI) and large language models—is a transformative development in the fields of data analysis, natural language understanding, and spatial analysis. Cartography acts as a critical enabler for GeoAI and large language models because it provides a way for users to visualize complex data, extract meaningful insight from it, and communicate their findings effectively.
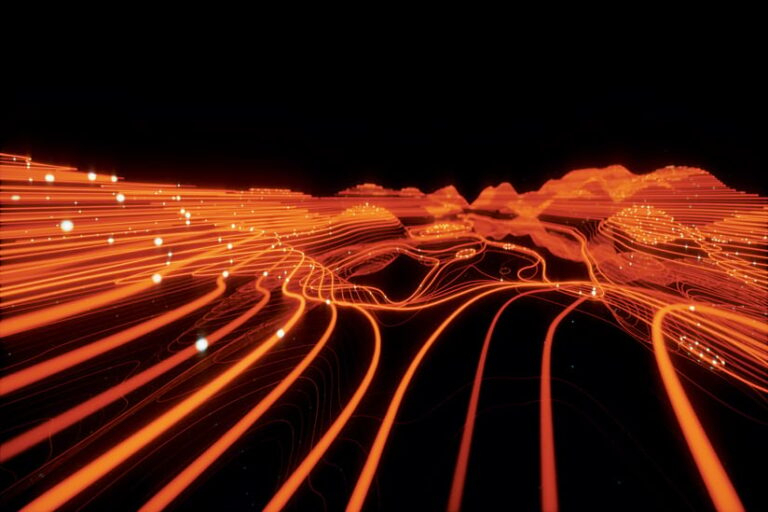
Four Concepts
The synergy between cartography and AI is crucial, as it allows for more advanced analysis and better decision-making by enhancing people’s capacity to interpret, visualize, and predict spatial patterns. In a world where humans are trying to tackle a changing climate, address the spread of urbanization, and manage ever more destructive natural disasters, the fusion of cartography and AI is increasingly vital, since all these issues require understanding spatial patterns and relationships.
Cartography’s role in GeoAI and large language models is extensive and revolves around the following four concepts:
Enabling the Visual Representation and Interpretation of Spatial Data
Cartography enables GeoAI-based models to present spatial relationships visually, making data more accessible and comprehensible. Maps can display the geographic distribution of data points, trends, and patterns that would otherwise be difficult to discern as raw, numerical data. This is especially important in GeoAI, where the data often comes from various sources, such as satellite images, sensor data, and social media geotags.
By integrating cartographic principles, GeoAI-based models can be used to create thematic maps that make it easier for decision-makers to understand complex geospatial data, facilitating data-driven planning and resource allocation. In fact, in this context, perhaps a new term can be coined: MapAI.
Enhancing Spatial Analysis Through Machine Learning
Cartography enhances GeoAI-based models by providing spatial context, which is essential for making accurate predictions and analyses. Cartography helps encode spatial features such as proximity, elevation, and land-use patterns, which can be critical for understanding the dynamics of geographic phenomena. For instance, GeoAI-based models can predict patterns such as deforestation, urban sprawl, and disaster risk by incorporating spatial relationships into analysis.
For large language models, cartography contributes by enabling a better understanding of spatial references in texts. When large language models are trained on text that includes geospatial information—including place-names, regions, and spatial relationships—cartographic data can help these models ground their language comprehension in real-world locations. This improves their ability to perform tasks such as generating travel recommendations, summarizing regional cultural differences, and analyzing location-specific phenomena within their historical and sociopolitical contexts.
Integrating Textual Data with Geospatial Data
Cartography provides a bridge for integrating textual data processed by large language models with geospatial data handled by GeoAI. Large language models can analyze a large corpus of text—such as news articles, social media posts, and historical documents—to extract geospatially relevant information. This information can then be geocoded and visualized through maps.
A practical example of this is disaster response operations. Large language models can process social media data to extract information about the location and severity of an event like an earthquake or a flood. By employing cartographic tools, GeoAI can map these reports to provide real-time situational awareness. This allows emergency responders to identify affected areas and allocate resources more effectively. The integration of large language models, GeoAI, and cartography thus facilitates a more comprehensive analysis, providing a nuanced understanding of events as they unfold.
Improving Decision-Making and Policies
The combination of cartography, GeoAI, and large language models can significantly enhance decision-making processes. Maps have always been critical tools for policymaking, as they help policymakers understand complex geographic relationships and patterns. With GeoAI, the predictive capabilities of AI models can be layered onto maps to show future trends, risks, and opportunities in various regions. This is important, since geographically contextualized information is key for effective communication and, ultimately, underpins decision-making.
In a sea of data, maps offer a good way to recognize patterns and detect anomalies—including clusters of disease outbreaks, trends in migration, and changes in the environment—and cartography is vital to how this data gets visually represented. For example, GeoAI can process remote sensing data to detect changes in forest cover over time. But it is the way in which this data is cartographically represented that enables map viewers to clearly understand these changes within their spatial contexts and forecast change by, for instance, identifying areas that are most at-risk of deforestation.
Furthermore, cartographic methods significantly improve the scalability of GeoAI. GeoAI often deals with vast datasets, and cartography allows these large datasets to be appropriately scaled and efficiently visualized through multilayered maps that show different aspects of the data at different scales. This allows map viewers to analyze both micro (local) and macro (global) spatial patterns, making it possible to compare trends across disparate regions while maintaining a high level of detail.
For large language models, cartographic knowledge improves their understanding of natural-language text that contains geographic references. These include mentions of locations, spatial relationships, and geographic features that are not always easy to interpret without spatial context. Cartography can inform large language models about spatial hierarchies—including relationships among cities, states, and countries—which refines tasks such as named-entity recognition and sentiment analysis as it relates to places.
Additionally, large language models often interpret and respond to location-based queries, such as when users ask about historical events, inquire about cultural norms, and request travel advice for specific regions. To answer these questions accurately and comprehensively, it is crucial for these models to have a deep understanding of geography. Cartography gives large language models foundational knowledge of spatial data, which helps them generate factual and contextually relevant responses.
As the use of AI continues to evolve, the role of cartography in refining and providing context to AI-based models will only grow. Thus, cartography is a fundamental part of the future of geospatial intelligence and AI-powered insights.
That is why the International Cartographic Association (ICA) is actively fostering the development of cartography’s role in AI by promoting research, collaboration, and the integration of geospatial technologies with artificial intelligence. Additionally, through initiatives such as the Commission on GeoAI (chaired by Dr. Samantha Arundel, director of the Center of Excellence for Geospatial Information Science at the US Geological Survey), the Commission on Geospatial Analysis and Modeling (chaired by Dr. Xintao Liu, associate professor in the Department of Land Surveying and Geo-Informatics at Hong Kong Polytechnic University), workshops, publications, and conference sessions, the ICA supports the development of new cartographic techniques that leverage AI.
Understanding spatial phenomena in a digitally connected world is an interdisciplinary undertaking, and the ICA seeks to bring together cartographic and geospatial professionals to ensure that spatial concepts and techniques continue to underpin reasoning in any spatial context.