In the large, urbanized area southwest of Washington, DC, residents of Fairfax County, Virginia, enjoy vast canopies of evergreen trees—including hemlocks—year-round. Unfortunately, there is a pest devastating hemlock trees throughout the southeastern United States. The hemlock woolly adelgid feeds on the sap and water storage cells at the base of a tree’s needles, where the pests also lay eggs, causing an infestation. The “woolly” part of the pest’s name comes from the fluffy white appearance of the insect’s egg masses.
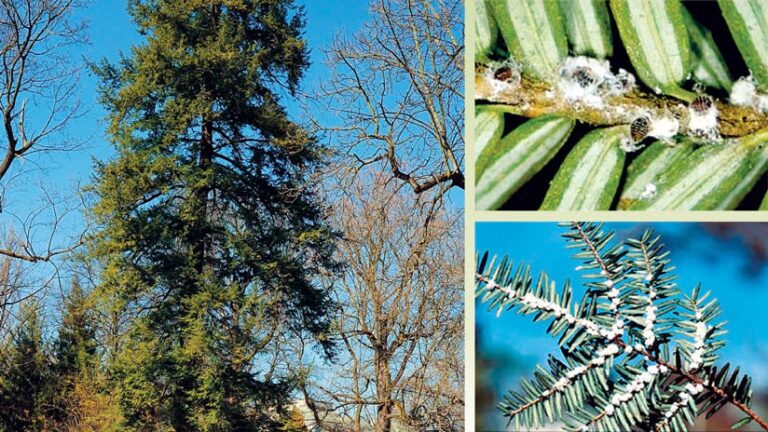
The Forest Pest Branch of the county’s Urban Forest Management Division (UFMD) is responsible for monitoring a variety of forest insect pests and tree diseases and provides public outreach and education to help residents take part in tree preservation efforts. Staff at the Forest Pest Branch understood that managing the hemlock woolly adelgid infestation and preserving Fairfax County’s existing contiguous tree canopy were critical.
Staff also recognized that mapping plays an important role in these endeavors. They needed to inventory the hemlocks and map out their locations as part of the management plan, but searching through aerial photography of thousands of acres to find them was going to be too time-consuming and labor-intensive. Staff needed a way to quickly prioritize field visits to areas that were likely to have large numbers of hemlock trees. From there, they could target areas for protective measures against infestation.
To do this, in 2023, UFMD urban forester Patrick O’Brien enlisted the county’s GIS Division. Fairfax County GIS analyst Greg Bacon decided to use ArcGIS technology and geospatial artificial intelligence (GeoAI) to automate the search for evergreens in aerial photography in hopes of finding the hemlocks among them.
Automating Tree Detection with a Deep Learning Model
Leveraging the analytical power of ArcGIS Pro alongside ArcGIS Image Analyst, Bacon created a unique workflow to find the hemlocks.
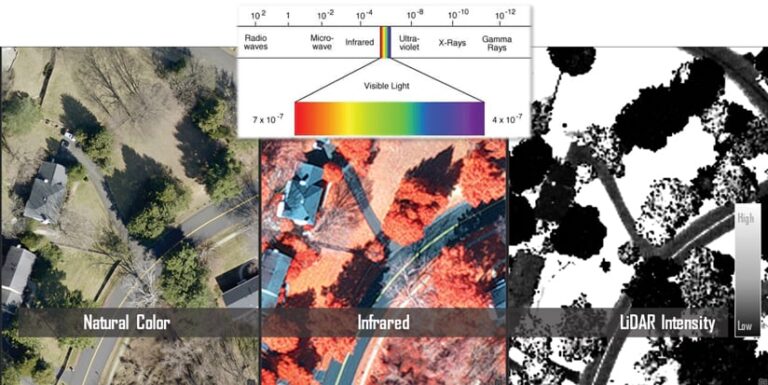
“UFMD is a longtime user of the county’s aerial photography. They’ve used the data in desktop and mobile mapping but have also worked with the GIS Division on more advanced projects such as land-cover classification,” said Bacon. “This project continued our collaboration in finding new ways for aerial photography to enhance their work and address evolving needs.”
Bacon selected leaf-off aerial orthophotography from 2022 as the input for a tree-detection deep learning model that is currently available as a pretrained model in ArcGIS Living Atlas of the World. ArcGIS pretrained models automate the process of digitizing and extracting geographic features from imagery and point cloud datasets.
This model was a great starting point for creating tree detection geometries on a variety of supported imagery. The model produced great results—yet it was just one part within a larger analysis pipeline. While the model was good at defining tree boundaries, the goal was to identify evergreen trees where foliage remains green and functional year-round.
To account for this, Bacon employed a series of ancillary data and techniques to further filter the data and identify areas with evergreens that potentially needed treatment. He used a variety of methods to aid in these selections, including the size of tree patches, the greenness of pixels within the imagery, infrared information within the source imagery, and lidar intensity values. This combination of factors allowed him to further distinguish between evergreen and deciduous tree species.
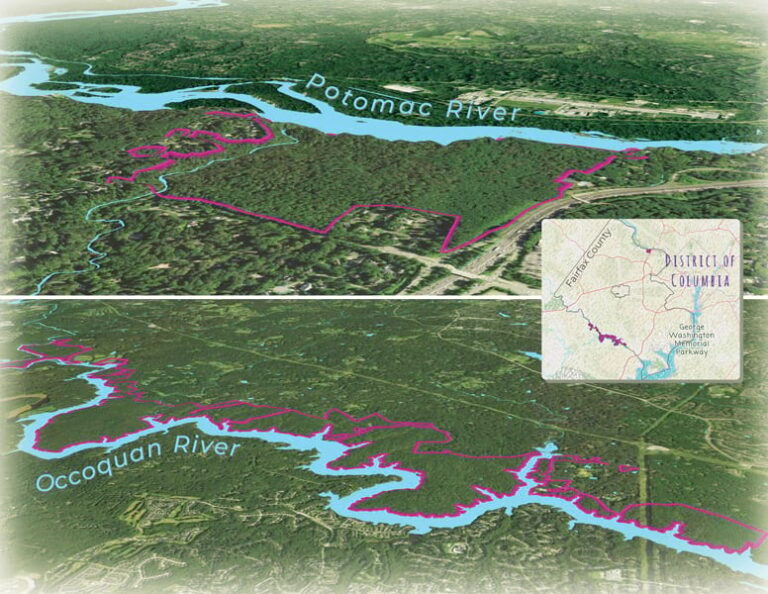
Working in a More Precise Target Area
All the data processing results were queried to provide a comprehensive picture of not only where Fairfax County’s evergreen trees were but also where hemlock trees were likely to be located. Once Bacon and the GIS Division made their selections in the imagery and generated outputs, the observations were converted to density surfaces. In the end, staff identified 33,110 evergreens out of 116,521 trees in the project zone—drastically narrowing the project down to a more precise target area.
The GIS Division shared its results with the Forest Pest Branch management team via a file geodatabase, and the urban foresters published the data to ArcGIS Online. This helped inform a treatment plan. From there, the Forest Pest Branch’s mobile team used ArcGIS Collector, which has since been replaced by ArcGIS Field Maps, to locate and treat infected trees in the study area.
While analysis of imagery data formed the core of the treatment plan, employing a well-rounded combination of GIS tools kept stakeholders informed and helped them quickly and efficiently allocate resources. The GIS Division will use imagery and conduct additional on-the-ground work to monitor the effectiveness of the treatment.
Using One Model to Train Another
The results of the hemlock woolly adelgid operation laid the groundwork for Fairfax County to do additional projects that involve using imagery to detect evergreen trees.
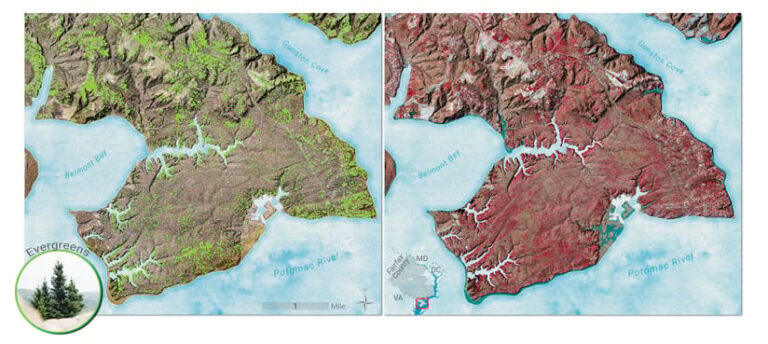
“Automating the process of mapping trees of interest, and doing that successfully with help from ArcGIS software and apps, established a benchmark for more efficient identification and monitoring moving forward,” said Bacon.
In 2024, the county used a selection of evergreen tree boxes to train a new deep learning model to find only evergreens. Staff employed the same three-band, three-inch-resolution imagery from 2022 that they used in the hemlock project to train the new model by creating image chips containing a variety of evergreen samples.
The resultant model was then run against images of the Mason Neck peninsula in southern Fairfax County—an area of approximately 9,000 acres that is nearly three times the size of UFMD’s hemlock project. The model mapped more than 36,000 evergreen trees throughout Mason Neck, and it performed best in underdeveloped areas that have dense clusters of evergreens.
Once again, having automated the process of using imagery to detect characteristics that separate evergreen trees from deciduous ones, UFMD was able to effectively plan more targeted mobile work in Mason Neck.
For more information on Fairfax County’s use of deep learning to target pest management work, explore the ArcGIS StoryMaps story Finding the Evergreen Needles in the Forest Haystack.